Crystal Ball: Business Resilience in 2030 and the Digital Dexterity Effect
Digital transformation is today’s tested and proven path to business resilience. We help manufacturers digitally transform to strengthen their resilience every day. But our era is defined less by permanence than by alacrity of adaptation. Manufacturers must already evolve their approach to business resilience to remain competitive.
Granted, the need for this evolution is neither intuitive nor obvious. And in fairness, resilient manufacturing enterprises in 2030 will look much like such enterprises do today. But the means for achieving and sustaining resilience by decade’s end will be radically different. Here’s why, and how to prepare for it.
Why business resilience will be different in 2030
Resilience describes a business’s ability to adapt to change effectively and efficiently. For many reasons, data-driven manufacturers are indeed able to adapt well. That’s why businesses that aren’t already data-driven are becoming so.
But digital transformation is a continuum. And few companies believe they have done all they need to do in their transformation. Most recognize that they are in the early or intermediate stages of digital transformation. That will change.
By 2030, businesses that lead their industry will have passed beyond the stages of digital transformation. The businesses will have entered a state of digital dexterity. In that state, business resilience manifests in sharply different ways than it does today.
How business resilience will be different in 2030
Business resilience based on digital dexterity has many facets. Among them, three stand out as being potentially unexpected. First, high-level objectives will remain vital to defining initiatives aimed at strengthening business resilience. But those objectives will not be the exclusive driver. Digitally dexterous manufacturers will employ a fluid governance model. This reflects the future reality wherein company-wide resilience initiatives will emerge from diverse organizational levels, and not always from the top.
A second difference in the way business resilience will manifest lies with the workforce. Digital dexterity means making sure that the workforce has access to, and understands how to use, your business’s data and technologies. Employees will have the information they need to understand how changing their own workflows could have wide-reaching benefits. They will be able to see ways to boost resilience from their specific position. These will be ways not obvious from a top-down view.
In fact, the top-down view will itself be different, which is the third key facet we are considering. Digital dexterity will mean that all executives – not just those in IT – feel at home with technology. In the same way that an executive today can assess how well one staff member is performing, they will be able to assess how well a technology is performing. For example, is a particular AI algorithm doing what it should, and is it enabling the desired business resilience outcomes? That level of digital literacy will be a basic requirement for leaders in digitally dexterous companies.
What you can do now to prepare for the future of business resilience
We’ve called out ways of enabling business resilience in the future that will be strikingly different. Preparing for those differences will set you on the right path. A cultural awareness and acceptance of shared responsibility for business resilience takes time to develop. But it also follows naturally as your workforce evolves on the path to digital dexterity. The key here is to ensure that everyone in your organization has the opportunity to participate in the digitization of their roles. Organizational change management will be instrumental in this workforce development.
Establishing a higher baseline of digital literacy among your leadership team takes time as well, and you’ll need to begin soon. Leaders must be able to evaluate technologies for their effectiveness as intuitively as they do employees, but that is predicated on educating and training leaders with the requisite skills. Ideally, upskilling and reskilling should always remain slightly ahead of technological change.
Business resilience in 2030 will be decentralized and deeply integrated in the manufacturing enterprises’ people, processes and technologies. It will be the inevitable outcome of your organization’s digital dexterity.
To learn more, please visit NTT DATA’s manufacturing page.
About the Author
Siva Gurupackiam is Senior Vice President of Manufacturing Industry Solutions at NTT DATA.
Placing Innovation at the Heart of Transformation
To build the right foundation for long-term growth, manufacturers must put innovation and digital strategies at the heart of transformation.
TAKEAWAYS:
● Massive geopolitical, technological and cultural changes have prompted unprecedented shifts in industrial manufacturing.
● As pressures mount to build smart products, digitize operations and exceed customer expectations, incremental approaches to change are falling short.
● Manufacturers need to design a transformation that is innovative, agile, cross-functional, and scalable to realize tangible business value.
Over the last several years, challenges related to geopolitics, technology, the pandemic and climate change have shaken the foundation of industrial manufacturing – creating tectonic shifts in how manufacturers think, operate and deliver their products and services.
The pressure to make products smart and connected, digitize the factory and operations, create more automated and reliable supply chains, and deliver on rising customer expectations presents significant opportunities to move toward radical growth. Yet many manufacturers’ current approaches – driven by siloed teams, functions rather than strategies, and static views of competitive and operational landscapes – present significant risks on the road to reinvention.
Legacy manufacturers trying to speed ahead into the future of smart products, digital platforms and new service-oriented business models urgently need a better way forward. A hybrid, innovation-led approach that incubates future businesses while simultaneously optimizing today’s products and operations can provide practical, value-driven solutions in both the short and long term.
Disruptive Forces Shift Operational Priorities
The range of disruptive forces manufacturers are experiencing has implications across the entire enterprise. These forces include:
- sector convergence
- power shifts within value chains
- evolving customer expectations
- volatile macroeconomic environments
- tougher workforce dynamics
- sustainability pressures
- transformative technologies
In response, manufacturers are prioritizing digital transformation and innovation-related investments. According to the EY January 2023 CEO Outlook Pulse survey, virtually all (97%) of industrial manufacturing CEOs indicated that continuing digital and technology transformation to deliver growth and operational advantages is either a very or fairly important near-term priority, despite near-term economic uncertainty.
Through these investments, manufacturers seek to revolutionize processes by implementing innovations such as digital twins, artificial intelligence and machine learning. At the same time, they are advancing product and service offerings across the value chain that are smart and connected.
However, to fully realize the significant opportunities arising from disruption and the value of these investments, manufacturers must tie them to a unifying vision of where their markets are headed.
“Legacy manufacturers trying to speed ahead into the future of smart products, digital platforms and new service-oriented business models urgently need a better way forward”
A Different, Innovation-Focused Approach to Manufacturing Transformation
Forging a new path to the future will require manufacturers to put their growth agenda at the center of transformation. This plan needs to be backed by a detailed strategic roadmap that accounts for both the innovations needed to succeed and the transformation required to launch and scale these breakthroughs in the market. It must also be developed with an enterprise-wide outcome in mind, breaking down functional and geographic silos.
While not every initiative needs extensive cross-organizational coordination and buy-in, many of the most prominent areas for manufacturers’ business reinvention are inherently interdisciplinary. Effective transformations demand engagement with a range of intersecting value drivers, including product and service innovation; customer experience; intelligent and sustainable supply chains; workforce and talent; and business model innovation.
Image 1: The value drivers of tomorrow
Image 1: A chart showing the value drivers of tomorrow that intersect include product and service innovation; customer experience; intelligent and sustainable supply chains; workforce and talent; and business model innovation. Source: EY
Once a decision is made to pursue small- or large-scale reinvention, manufacturers can maximize the speed, agility and long-term value generation by adopting the following best practices.
Start With the End in Mind But Also Know Where to Begin
With business challenges clearly defined, manufacturers should explore solutions using a future-back approach that starts with the end in mind. The critical first step involves rapidly defining and assessing tomorrow’s potential futures from the outside-in, and predicting how these future scenarios may shape customer needs, market conditions and value pools across time horizons. In parallel, organizations must also perform a thorough review of their current capabilities, particularly those that may play an increasingly important role in future competitiveness.
Taken together, assessments of a company’s current state and potential futures serve as the foundation of a new strategy that will act as the transformation’s North Star – guiding it relative to the level of ambition, while optimizing development speed and investment spending. This strategy should also provide a framework for cross-enterprise transparency and engagement.
Image 2: Our approach to transformation
Image 2: A chart showing the continuous innovation process which defines the future growth agenda, designs the transformation plan to deliver results both at the enterprise and functional level. Source: EY
Design the Transformation With a Hands-On Approach to Innovation
Rapid prototyping is an essential step for manufacturers to substantiate their future views and refine perspectives on must-have innovations. Multiple, accelerated iterations of both physical products and digital offerings are important for assessing features and costs in the context of various customer expectations, demand levels and competition scenarios.
Making the future tangible will pay dividends in the design phase of the transformation. Understanding necessary enterprise-wide adaptations – from specific technology or talent enhancements to new business models – will inform an achievable, cross-functional plan. The ability to “show your work” will also help build support across various internal constituencies as they are enlisted in the effort.
Start with the end in mind
Image 3: A chart showing the continuous innovation process which defines the future growth agenda, designs the transformation plan, analyzes existing products and operations, connects new products, embeds digital platforms to redesign As-A-Service (XaaS). Source: EY
Accelerate Results By Incubating New Business Model Concepts
When future growth depends on technologies, customer expectations or new value pools that are not addressed by a manufacturer’s current core competencies, innovation at the business model level may be a solution. For example, what are the implications of collecting, analyzing and monetizing data from a new connected product? Successfully assessing and addressing these impacts could enable manufacturers to leapfrog the competition.
“To fully realize the significant opportunities arising from disruption and the value of these investments, manufacturers must tie them to a unifying vision of where their markets are headed”
Given the enterprise-wide implications of such changes, legacy manufacturers should prioritize small-scale experimentation with a lean, internal start-up style approach. In conjunction with ongoing physical product innovation, manufacturers will want to invest in teams to explore the internal and external infrastructure and capabilities needed to support an operating model for an offering’s full lifecycle. This approach can be particularly beneficial for evaluating the technology infrastructure required for a potential new business model. By using focused, lean teams, manufacturers can leverage agile sprints to further define capabilities and architectural requirements as the transformation progresses.
Identify Ecosystem Partners to Address Critical Capability Gaps
As manufacturers innovate, they will have to decide whether their long-term strategies are better served by seeking ecosystem partners versus internally developing or acquiring new capabilities. Assessing targeted value pools and key differentiators of success can help inform these decisions.
Manufacturers whose future offerings are likely to depend on the secure sharing and analysis of data at scale will want to consider partnering with technology providers as a more efficient path to market. However, the factors to consider when choosing a mission-critical partner can be extensive, particularly given the high cost of potential failure. Legacy manufacturers can benefit from a structured process to weigh the benefits of various collaborators.
Manufacturers exploring business model innovation may also find a need for ecosystem partners outside of the technology sector. For example, future mobility business models may involve close collaboration among vehicle OEMs, energy, infrastructure and insurance firms.
Key Questions For Manufacturers Considering Reinvention
The urgency around transformation has escalated as manufacturers grapple with multi-faceted disruptions. Reactive, short-term focused responses limit the vision for enterprise-wide transformation. When thinking about the right approach, manufacturers should consider the following questions:
- Product and service innovation: How do we evolve our product portfolio to create dynamic products and services that address customer demands for smarter and more connected features, customization, and safety/security?
- Customer experience: How does a shift from B2B to B2C or D2C impact how we operate today?
- Operations:
a. How can we leverage emerging technologies to future-proof our supply chain?
b. How do we design our future manufacturing capabilities as a competitive differentiator? - Workforce and talent: How can we advance innovation as a core capability through hiring, developing, training and incentivizing our employees?
- Business model innovation: How do we innovate to drive the core business to peak profitability while simultaneously incubating new growth engines?
Answers to these questions may help leaders address strategic or operational gaps. With a clear vision of their organization’s future ambitions in mind, and a strong strategy for innovation-focused transformation, leaders can position their organizations to accelerate growth and leapfrog their competition. M
About the Authors:
David Takeuchi is EY’s Global Strategy and Transformation Business Model Innovation Leader
Jerry Gootee is EY’s Global Advanced Manufacturing Sector Leader
Claudio Knizek is EY-Parthenon’s Global Advanced Manufacturing and Mobility Leader
M2030 Perspective: FOMO Can Spark Next Digital Revolution
What the past tells us about an AI and ML enriched future
TAKEAWAYS:
● Manufacturers can leverage AI/ML strategies to overcome the prohibitive cost related to data-driven decision-making.
● AI and ML are business investments not technology investments.
● In the future, AI will drive people, process and technology changes that deliver manufacturing ROI.
Past is prologue when it comes to AI
More than two and a half decades ago the world was in a state of panic and desperation over technology. The situation was simple: business solutions that ran supply chain, financial, and payroll systems – to name a few – could have a basic flaw in how dates were stored, and on January 1, 2000, they could simply stop working and business would stop working. The Y2K problem led to a massive investment in IT spending, turbo charging the technology capabilities that brought about the recent advancements in artificial intelligence and machine learning (AI/ML).
Then as it is now, we have a disruptive concept that threatens to upend business as usual, and executives can no longer avoid the hard discussions on how to prioritize technology investments. Then as it is now, we have a juxtaposition of business and technology interests intersecting a common goal of relevance and sustainability. The difference is that with artificial intelligence the fear of missing out is driven by excitement and opportunity.
“The difference between artificial intelligence and the Y2K problem is that with AI the fear of missing out is driven by excitement and opportunity”
The concept of data-driven decision-making, which is the basis of leveraging AI/ML in manufacturing, is not new but it has been relatively unattainable due to costs. Manufacturing is a people-driven enterprise that needs to maximize efficiencies to keep costs down and react to customer demands. The challenge is how to balance business operations with the disruption in leveraging new systems and process, balancing the potential skill gaps in using automated systems, and driving towards measurable business outcomes. As we move toward the year 2030, AI/ML can be the unifier of all those goals if manufacturers can map executive goals and business outcomes to people, process, and technology.
People power to drive top-down transformation
With the popularity of topics around generative AI and ability to access technology infrastructure for various back-office automation tasks there is urgency to innovate to stay ahead. The change that occurred since last year is driven by awareness, access, and actionable insights in the manufacturing space driving pragmatic business innovations. Executives have greater awareness of how to leverage AI/ML in business operations and are empowering their teams to invest in digital strategies around automation and efficiency. IT and business leaders within organizations are finding common cause in terms of goals for improving supply chains, understanding customer behavior, improving product quality, and investing in sustainable practices. The general concerns and narrative that AI may replace people is shifting to how AI is helping teach the workforce and automate complex production processes. While common misconceptions around AI sophistication and the practicality of certain use cases still exist, the urgency of change is overcoming the stagnant comfort of the predictable. As we move towards 2030, we will see more broad adoption of these technologies to work side-by-side and seamlessly in performing business actions severely reducing manual repetitive tasks.
Pragmatic solutions to drive maximum ROI
Concepts like Industry 4.0 drive business goals towards automated dark factories heavily leveraging IoT sensors that constantly improving plant output. Looking beyond factory operations, AI/ML solutions can optimize entire value chains as illustrated in a sample view of an equipment as a service manufacturer/distributer.
Figure 1: Leverage AI/ML solutions to drive enterprise value in manufacturing and after-market service.
The benefits earned from leveraging AI/ML solutions to track asset management and performance, labor, and financial planning are an example of data-driven decision-making and leveraging “one version of the truth” in business operations with clear ROI.
Considering these use cases can be setup very quickly and incrementally, they can drive manufacturers’ changes and urgency to adopt measurable business innovations.
Investment in business versus technology
Manufactures, like other businesses, are loathe to spend money on IT for the sake of re-platforming the business. Perception of IT spending as a costly task changes significantly with AI/ML-powered solutions.
The target goals for these solutions are business productivity, plant scrap reduction, financial visibility, and improved revenue, which are markedly more impactful than purchasing a new business application. Other tangible business applications are better customer and employee experience driven by personalized and contextual actions. The implicit benefits of cloud-based architecture and improved cyber security are attractive but not as much compared to improved margins and responsive business operations. Focusing on technology as a means for better business results is a big difference in driving future investments in the next several years. Manufacturers will invest in approaches that drive better, transparent, explainable, and faster time to value that provide seamless decisions between different business systems with more emphasis on action versus interaction. Focus will be on how automated decision-making results in improved productivity versus the current focus on user experience and complex manual tasks.
Bringing digital revolution 2.0 into focus
As manufacturers begin adopting data-driven decision-making tools, the current AI/ML strategies will change to adapt to more complex ecosystems. While we already have focus on sustainability and automated decision-making, the future will bring more emphasis on circular economy value chains involving leveraging data across different companies across the product lifecycle and responding proactively to customer needs. By 2030, this will open up an avenue to track products, customer use, recycling, and maintenance, which will expand business opportunities for new product development and new manufacturing processes. This will ultimately and hopefully lead to digital revolution 2.0! M
About the author:
Sandeep Anand is Senior Director of Decision Analytics and Science Platform at Infor. He has over 15 years of experience building and delivering AI/ML solutions. Experience includes solutions around yield/scrap, supply chain improvements, and smart asset management strategies. He leads the AI/ML practice at Infor, a leading enterprise cloud solutions provider.
Crystal Ball: Quo vadis, Industry 4.0 and digital transformation?
In the latest in our Manufacturing in 2030 Crystal Ball series, we look at where Industry 4.0, transformation and digital value creation are headed
Global economic disruptions include supply chain bottlenecks, trade restrictions and rising prices for raw materials, all of which give manufacturers a big headache. Producers are also under increasing pressure to readjust their manufacturing footprint and reduce consumption to meet sustainability targets. Most manufacturers are still stuck with a geographic footprint set down in the 1980s, driven by ongoing labor arbitrage around the globe. Large factories are still predominant in low-cost regions like Asia, Eastern Europe, and South America. Accelerating trends toward automation, upskilling and high demand for customized products, combined with increasing regulatory requirements for sustainable manufacturing, are pressuring companies to rebalance this equation. To increase efficiency and respond to threats and trends, many producers have implemented Industry 4.0 technologies.
But quo vadis? Where are these trends going?
The Industry 4.0 vision – smart, networked factories, closer to customers
Industry 4.0 technologies offer no less than the ability to redesign the global manufacturing footprint, to position factories closer to markets, reduce logistical nightmares and increase the visibility of suppliers and customers.
The digital transformation offered by Industry 4.0 technology has four central goals:
1. Customization, insight-driven manufacturing and lot sizes of one
With customers increasingly demanding highly personalized products, mass customization has become a competitive playground. Western manufacturers need to differentiate their offering (via customization) from cheaper offshore competition to retain their markets. Industry 4.0 includes a wide range of technology innovations like 5G, artificial intelligence (AI), machine learning (ML), PLM and digital twins, MES, IoT platforms, and additive manufacturing (3D printing). These can support the agility, flexibility, and efficiency to offer customers such highly personalized products and services, thereby enhancing their brand experience, increasing loyalty, satisfaction, and ultimately, profitability.
Infor customers in manufacturing have switched from series production to highly customized operations in segments as diverse as the fabrication of individual sports rifles for biathlon athletes, medical science equipment, commercial laser pointing products, the fenestration industry (producers of windows and doors) and industrial cranes. Product individualization based on unique combinations of modular components and very late customer change requests have become essential ways to improve the customer experience. The vision is that consumers can switch their product configuration on the fly.
With an increasing shift of focus toward software-driven experience-based services, there will be pre-purchase changes for specific experiences. Today, some cars already come with three driving modes: sports, comfort and eco. In the future, cars may be chosen for events instead of a car’s lifetime – a camper van just for the summer holidays, an SUV for the winter holidays, a limousine for the business trip. All these will be equipped with event-based options, possibly an extra seat row for the children and special cooling options for the summer holidays, heated steering wheel and a snow-drive option for the winter holidays, and additional horsepower for a long-distance business trip.
2. Incremental innovation and transformation to product-as-a-service
Product lifecycles are becoming shorter as customer attachment to one-size-fits-all physical products decreases. Companies no longer compete exclusively on product features, instead offering value-added services, and manufacturers are adapting their operations to optimize business efficiency. In automotive, software-enabled service platforms are a new ground for differentiation, replacing hardware-related factors such as engine power and precision-engineered door handles. Success in this realm requires more knowledge about customers, software-based services and the ability to innovate and respond quickly to changing customer preferences.
3. Digital innovation reducing market distance
To reduce delays and transportation costs, leading manufacturers build smart factories closer to the customer. Redesigning the manufacturing footprint is especially imperative for the manufacturing industry, with its notoriously complex, fragile, and logistically challenging global operations and supply chain. New business models and technologies help reduce the distance to the end consumer. With digitalization, automation and robots, factories need fewer workers but more skilled ones. Thus, locations in Western countries become attractive despite higher labor costs.
With the digital revolution unfolding, there will be more ways to overcome global footprint constraints, such as these two concepts.
First, a widespread network of high-tech automated micro-factories. The micro-factory was invented in 1990 by the Mechanical Engineering Laboratory of Japan, referring to cell-based manufacturing in a multitude of small and highly automated plants, to deliver customizable designs locally. But it has not yet become widespread. Today, we observe much interest, but few operational implementations of micro-factories. Despite the appeal of quick deployment, higher flexibility, lower capital investment, and reduced environmental impact, the implementation of micro-factories is limited to start-ups and new business models. Even the discussion about micro-factories has quieted down. The assembly line still plays a major role, and the efficiency of a modern automated volume production site with just-in-sequence operations are hard to beat with a more distributed approach to production. Gartner® places Mobile Factories just before reaching the tipping point of “Peak of Inflated Expectations” on the just released Hype Cycle™ for Advanced Technologies for Manufacturers, 2023 and classifies them as: “5-10 years to mainstream adoption.”1
So, the micro-factory concept could become a strong factor to increase the value of customization and higher flexibility, fueled by zero distance and innovation in computing, robotics and AI. But it is not yet a top priority for operational executives.
Second, a relocation to high-cost countries for access to resources and customers. In contrast to the slow development of micro-factories, a counter-trend of companies setting up rather large factories in Western countries is burgeoning. First, there is a plethora of gigafactories. Introduced by Elon Musk, the term refers to giant plants to produce power units generating gigawatts of power via EV batteries. Gigafactories are set up in places like Canada, Austria, the United States, and Norway, by companies including Volkswagen, Northvolt, Miba and Frey. In addition, Infineon and Intel launched large factories in Austria and Germany to produce their core microchips, while Rheinmetall created a factory for producing electronic components for the F-35 fighter plane. This trend is driven by access to excellent logistics and highly qualified resources rather than by cheap labor.
4. Agility to deliver business and technology requirements
An effective platform enabling swift reaction to change, adjustment of the global footprint and further efficiencies via automation requires a comprehensive digital transformation. But for many organizations, challenges to connect separate technologies, true digitalization of processes and the complexity of existing systems continue to be roadblocks to executing a digital vision.
McKinsey has outlined critical success factors, including these four:
– A truly scalable operating model
– Distributed technology allowing local and independent innovation
– Access to reliable and current data when needed
– Strong adoption and change management
Cloud ERP at the core of Industry 4.0
Historically, manufacturers have architected their organizations and IT portfolios with a traditional monolithic enterprise-centric and on-premises ERP. But this inside-out model, with static, scheduled interfaces to the “outside world,” is unfit for tomorrow’s intelligent factories in response to an even more dynamic, constantly changing and truly real-time business environment. Legacy ERP systems focus on structured data from ERP transactions, not unstructured data that has become business relevant (e.g., IoT, clickstream, social media). This siloed, intra-organizational and structured-data focus does not provide the agility to adapt to and get ahead of current and anticipated business conditions.
The demands of the modern economy and the forces discussed above have led to replacement of monolithic ERP with a more modular, standardized cloud-based “hub and spoke” network approach, which can adapt and scale in line with business priorities. The capabilities to integrate, manage data, secure data and applications, and identify and provide optimal user experience have become the focus of overall enterprise strategy. The modular ERP approach is built around a core, vertical-specific ERP solution as a strategic integration platform to connect to supplemental applications, potentially from multiple vendors. This core ERP becomes the foundational platform for agility and innovation at scale. Additionally, embedded and advanced capabilities enable more intelligent automation by leveraging AI- and ML-driven insights and actions to improve the manufacturing process.
Digital cloud platform impact on time-to-value
The Infor digital ERP platform is designed to shorten time-to-value and improve customer experience to ensure success and improved business outcomes.
It is an industry-specific solution with preconfigured processes built in, and incorporates learnings from the sales process into the provisioned product. This is coupled with a heavy investment in tooling and content to shorten the initial implementation effort, cost and risk, and have the catalogue of predefined industry processes to accelerate deployment. The cloud simplifies updates and eliminates the need for major upgrades and for a large technical team to implement and maintain business applications.
It is set to become the central platform for dynamically driven innovation. Every company that joins increases the industry cloud scale in sectors such as automotive, industrial manufacturing and A&D, and accelerates the need and pace for relevant industry-specific additions. In 2030, industry communities will drive standardized cloud-based functionality based on their needs for manufacturing functionality, compliance with regulation, and process optimization through deep strategic interaction to set priorities per industry. Upgrades will be deployed immediately as standard additions with transparent and global KPIs throughout their entire manufacturing footprint. Over time, there will be a rich library of relevant tested use cases for process optimization based on AI per industry, available to all participants.
And the winner is … the smart, agile factory
Success belongs to the prepared. In today’s digitally transforming and connected discrete manufacturing industry, being prepared requires a re-evaluation of traditional business processes, technology capabilities and the main traditional asset, the global manufacturing footprint. Critical across each of these dimensions is effectively connecting systems and locations in an open and standardized structure and harnessing the power of data.
Manufacturing companies started to invest in new digital technologies such as AI to improve operational efficiency in areas such as demand forecasting, fault finding in production and warehousing. But today, most organizations struggle to roll out new technologies to the global universe. Those who will seriously invest in their digital platform to build a dynamic, intelligent and connected factory network put themselves into a favorable position to deploy consistent innovation steadily, quickly and globally. This change will not happen overnight. The move toward digital workflows and transparent open standards requires the whole organization to shift. Those who commit to that journey will be able to use the digital innovation benefits immediately to their business advantage in 2030. They will gain the ability to instantly respond to market disruptions, customer requirements and regulation, and create new products and services on the fly.
In an increasingly uncertain market environment, success will reward manufacturers that most effectively align new data management skills with modern information technology platforms to render their factories truly smart and insight driven. This includes the enablement of closeness to customers and their need for experiences rather than traditional products, decision-making adapted to swift reaction to change, and real-time, dynamic, sustainable and market-relevant manufacturing.
About the Author:
Dr. Henning Dransfeld is Director Industry & Solution Strategy at Infor. He is responsible for the development and implementation of Infor’s growth strategy in the discrete manufacturing in segments including A&D and automotive manufacturing in EMEA. Dransfeld has years of experience as an industry analyst. At Information Services Group (ISG), he was responsible for manufacturing, data analytics and IoT across Europe. He also led numerous consulting projects in these and related specialties. Prior to that, Dransfeld held positions at Ovum (now Omedia), T-Systems, Forrester and Experton.
1Gartner Hype Cycle for Advanced Technologies for Manufacturers, 2023 Published 25 July 2023 – ID G00789107 By Marc Halpern, Sudip Pattanayak, Christian Hestermann, Alexander Hoeppe
GARTNER is a registered trademark and service mark of Gartner, Inc. and/or its affiliates in the U.S. and internationally and is used herein with permission. All rights reserved.
Crystal Ball: A Futuristic Workforce Odyssey
For humans and machines to work together by 2030, the workforce evolution must start now
Extrapolating from the results of NTT DATA’s recent research report, Innovation Index: Shifting from Disruption to Growth, Kim Curley, NTT DATA’s Digital Evolution Leader and Vice President of People & Organization, shares her insights about the future of the manufacturing workforce in the first of our Manufacturing in 2030: Crystal Ball series.
Picture this: the year is 2030. Across the U.S., the manufacturing workforce has evolved into a symphony of humans and machines working in harmony. This dramatic transformation has made the workforce a technology-enabled powerhouse. Artificial intelligence, robotics, and virtual reality lead the charge, transforming the factory floor into a buzzing and orchestrated hub of activity.
Workers use advanced technologies to perform their tasks more efficiently and safely, collaborating seamlessly with their robotic counterparts. The shop floor thrums with the smooth whirr of precise machinery and the hum of advanced systems. Augmented reality displays guide workers through complex tasks. And to make this possible, the workforce has become a melting pot of diverse talents and skills that is continuously learning and developing
It is a rosy view of the future, but realistic based on current data and trends that illuminate a path to this future state.
Changing Skillsets for 2030
The 2030 manufacturing workforce will be doing things quite differently than they do today. Four in 10 manufacturers have already begun leveraging augmented or virtual reality devices, and that will only increase. That usage will expand to support workforce instruction and training. One example of this could be teaching workers to switch over a production line. Providing that training in a virtual reality environment would mean the company doesn’t need to shut down real-world production.
As the usage of advanced technologies rapidly expands, the skillsets of the manufacturing workforce must expand, too. In addition to physical technical skills, workers will need to strengthen their understanding of technologies as well as problem solving, critical thinking and leadership skills. To build that workforce over time, you must start by expanding the skillsets of managers and leaders now. Starting today is critical to being successful in 2030, indeed, 39% of manufacturers report that they have already increased their investment in leadership development.
A More Resilient Enterprise
The evolved workforce will enable significant benefits. The big one is resilience. An augmented workforce better enables the company to respond to changes in the marketplace. With such a workforce, companies can train faster, reset the lines, and change worker skillsets more rapidly. Paving the way for this evolution, upskilling and reskilling programs for new and/or existing workers are underway today at 54% of manufacturers. More broadly, making your workforce future-ready entails steps such as improving safety, productivity and talent; incentivizing workforce agility; teaching new leadership skills; engaging the workforce; and maintaining a future-focused mindset. We’ll look at each in turn.
Improving Safety, Productivity, and Talent
The NTT DATA 2023 Innovation Index survey showed that businesses are increasingly addressing potential disruptions in strategic plans because these events present opportunities for those businesses that can act with resilience and speed.
So, it is not surprising that manufacturers are placing increased importance on their workforce and the workforce’s ability to adapt and move quickly. The more connected and engaged the workforce is, the better it can shift and flex when the unexpected happens.
Three vital areas come immediately to mind when considering how these coming changes address the problems facing today’s manufacturing industry. The first is safety. As noted earlier, one of the best commercial applications of virtual technologies is training. Training workers to complete physically demanding or potentially hazardous tasks in a completely safe environment — without the cost of physically constructing such simulated environments — is a game changer. Fully 77% of manufacturers report plans to use these technologies within the next two years. That’s well ahead of the cross-industry average of 64%. Using these tools for ongoing training programs helps ensure that workers know exactly what they need to do and how to do it. And it does that while protecting their safety and the safety of the products they manufacture.
A second area of lift is in productivity — specifically, decreasing production errors and downtime. The proliferation of sensors enabled by 5G, advanced computing and machine learning technologies gives us better insights than ever before. These insights enable us to better plan and use scarce resources, another game changer.
The third area is talent. Emerging technologies in the factory call for a new-collar workforce that understands both manufacturing and Manufacturing 4.0. As the industry increases its use of and innovation with advanced technologies, more visionaries will be attracted to manufacturing. At the same time, advocacy initiatives like the Manufacturing Institute’s Creators Wanted program help spread information about this shift in manufacturing work so the next generation of young talent is aware of these opportunities to work with technology. This shift in the reputation of the industry overall can help bring the best and the brightest talent into manufacturing. The workforce will transform from the outside in as it changes from the inside as well.
Incentivizing Workforce Agility
For people and technology to align more closely, it will be important that the workforce sees the benefits of change. Many additional technologies come into play with a technology-enabled workforce. Remote collaboration tools and digital twins can allow manufacturers to assess and optimize processes virtually. These tools may require their users to have specific skills, such as a familiarity with the way data is used to create digital twins, to realize their full value. An agile and augmented workforce will be prepared with those skills or will be readily able to develop them.
The heightened importance of the workforce will call for momentous changes. Historically, manufacturing has considered the human as a component of the manufacturing line. The concept of human capital itself came from the manufacturing industry. Shifting our thinking changes how we develop people and give them desirable career progressions. It also impacts how we pay and incentivize people.
Adopting practices for compensation, rewards, and training from other industries to attract and keep the best and brightest can be beneficial. In high tech, for example, companies such as Google, Apple, and Facebook prioritize employee development and learning opportunities. They offer training programs, mentorships, and career advancement pathways. Some 84% of manufacturers have at least piloted investments in training for employees in areas such as factoring customer behavior into decision-making. To date, though, just three in 10 manufacturers report adjusting total rewards packages to increase their competitiveness when searching for new talent or retaining existing workers.
New Leadership Skills
The path to a future-ready workforce will include overcoming hurdles. One of the biggest is the need for a radically new style of leadership. Leaders in-house today often have massive institutional knowledge and a long history of viewing the workforce in a particular way. Having such leadership has often led to success. But going forward, that will need to change. That is a challenge. You have to teach the most senior leaders to see the workforce — both its potential and its needs—differently. But even incremental change comes slowly. Presently, for example, only 13% of manufacturers report being highly effective at delivering on worker-centric policies such as flexible working options and schedules.
Certainly, you cannot change a whole company’s culture unless you’re working from the top down as well as the bottom up. But once you do, you are positioned to develop and sustain a culture of innovation, experimentation, and resilience.
Engaging the Workforce
The workforce can and should be an active part of its own evolution. First, engage with your workforce – truly engage. Understand who your people are and what is important to them. Find ways to connect them to your company’s purpose and the impact you want to have on the world around you. The stronger those connections are, the more powerful a force your workforce can be in driving the transformation you’ll need over the coming years.
Second, expose more of the workforce to technologies and how these tools can help them and the business. Manufacturers are slightly behind the cross-industry average at the use of updated tools and technologies, with only 29% considering it important to employee satisfaction and engagement. Our Innovation Index shows that the best ideas to advance the business most often come from the individuals who already know the most — your workforce.
A Future-Focused Mindset
Mindset is everything. Without a growth mindset at every level of your organization, you will struggle to accomplish a transformation that will drive success between now and 2030. This mindset must be pervasive, encompassing everything from policies to hiring practices, investment decisions and the employee value proposition you put into the marketplace. When you embrace a mindset that says we are going to try new things and grow, and your workforce embodies it every day, you will create a resilient organization that is comfortable pushing boundaries to create the future.
About the author
Kim Curley is Digital Evolution Leader and Vice President of People & Organization at NTT DATA.
M2030 Perspective: More Robots, Better Jobs. But How?
Manufacturing Leadership Journal content and MLC resources are exclusively available to MLC members. Please sign up for an account or log in to view this content.
M2030 Perspective: Exploring the Promise of Industrial AI
AI promises to foster a mutually augmented human-machine relationship, making the workforce more productive and strengthening manufacturing capability.
Artificial intelligence is already becoming a commodity tool that is permeating our lives. If you have used Google maps, Apple maps, or shopped on Amazon, then you have already been touched by AI.
Driven by Data
AI is essentially the simulation of human intelligence using computers. Machine learning (ML) is a subset of AI that is used to automatically learn from data without being explicitly programmed. The two key words here are simulation and data. While we’ve all heard about the great promise of AI, it is still a simulation of human intelligence. Right now, human intelligence is superior to artificial intelligence in many sectors, and certainly in manufacturing. Part of the reasoning here, is that AI has yet to be trained in the vast field of manufacturing. Such training requires data. One thing that we do know from manufacturing is that we have significant levels of automation, and that automation has serious amounts of sensors and sensing capabilities on board. So, while there is still much to be learned about AI in manufacturing, we do have substantial amounts of data for that training.
Understanding the Opportunity
Many initial forays in using AI in manufacturing have been tried. One approach is to take all of the data coming from manufacturing operations and feed them into commercially available AI algorithms to find patterns linking various elements of the manufacturing process to the outcomes (e.g., quality, process health, and the tuning of operations). Many of these attempts are what one would call “black box”, in that they are basically just throwing the data at AI systems to see what happens. This approach has had some limited success. However, more successful approaches have used data with models that are well established but augmented by AI. Augmented is the key word here. An AI augmented model approach uses models based on what we know about our manufacturing processes and supply chains and augments those models to yield more accurate and refined results.
For example, new generation design (CAD) tools employ AI to help design components based on variables such as the load requirements on a part, while minimizing its weight. The approach is generally called generative design, and it creates some very complex structures. One might think that such complex parts can easily be 3D printed – just hit the print button! – as we can now 3D print almost any geometry. However, a person with significant experience in 3D printing will tell you that this kind of print button does not exist and there remain numerous limitations on 3D printed parts. A part generated by an AI design alone may not, in fact, be printable. However, an expert who understands both the goal and the limitations can work with the AI system to generate a lightweight part that can effectively support the required load while still being printable. This is where artificial intelligence works together with real intelligence. The AI is augmenting the human designer, and the human designer is augmenting the AI in this instance. The key is that both are working together.
Improving the Models
Today, we are just starting to take our data and augment our models to help us better understand our processes. Within a few years, we will have improved models that better utilize sensor data to help us predict the future health of our processes. For example, higher end machine tool manufacturers are starting to place more sensors, such as accelerometers, on their spindles. They train their AI algorithms to understand the sensor output when the spindle is healthy, and then have the algorithms use that knowledge to determine if the spindle has developed a problem. Furthermore, the algorithms can often determine a pending problem, and when it is going to happen. So now, a plant might shut a machine down for maintenance in advance of a breakdown. Thus, repair might happen during the weekend, when the machine is scheduled for maintenance, as opposed to waiting for it to break at an inopportune time such as in the middle of a shift.
“AI has yet to be trained in the vast field of manufacturing. Such training requires data. While there is still much to be learned about AI in manufacturing, we do have substantial amounts of data for that training.”
Such applications of AI will not be a silver bullet. The interpolation/extrapolation limitations of AI will still be in place. That is to say, there is a good chance that AI will not do well outside the scenarios that generated its original training data. So, if a completely new problem or situation is presented to AI, its response can be highly unpredictable. Therefore, we will have to understand its limits. For example, there is no algorithm that can accurately predict when a spindle will fail, until the spindle starts to fail. Statistics can be used to estimate failure (for example, based on experience the spindle may be expected to last so many hours of operation), but until the actual failure starts to occur, one cannot use AI, or any other techniques, to precisely predict that failure. For example, statistically speaking, the spindle might be expected to last for 10,000 hours of operation. However, if a severe crash occurs, that life expectancy might be substantially shortened. That being said, if crash data are fed into AI models, we might get a better feel for how much time was taken off the spindle life due to the severity of the crash, so there is another instance where we can augment our spindle life model.
Augmenting the Human Workforce
Just as with automation, people often express concerns about AI replacing humans in manufacturing jobs. Certainly, we will not see the Skynet AI scenario from the Terminator movie series that is in total control. What we will see is that the AI will augment the human workforce making it more valuable and effective. So once again, rather than artificial intelligence, we will see augmented intelligence. Just as with the earlier generative design example, we can use AI to help the human workforce, and the human workforce can be used to train AI.
Mixed Reality AI
One great technology advancement that is presently coming on-line and will be fully integrated into manufacturing operations by 2030, is mixed reality, which is a blending of the digital and physical worlds. For example, in a plant many workers may have to wear safety glasses, so why not make them augmented reality (AR) goggles? Such goggles could ensure that an operator follows the right procedures and adheres to all the required safety protocols. It could also ensure the correct insertion of all of parts into an assembly. Such an approach is the best of both the human and automation worlds.
Automated assembly makes sense in a highly controlled environment when thousands or tens of thousands of assemblies are being made and the components of the assembly are always in the same location and orientation so that automated units such as robots know exactly where and how to put up these parts. But if a part is backwards or falls over for some reason, the robot may have great difficulty picking it up. A human, however, is very adept at determining a part’s location and orientation and picking it up to put into an assembly correctly. Furthermore, AR goggles could ensure that the person picks up the right components and puts them into the right locations. An added benefit to this, is that a digital passport can then be generated for the assembly that records the correct assembly procedure documenting every process step ensuring the assembly’s quality.
“Augmented is the key word here. An AI augmented model approach uses models based on what we know about our manufacturing processes and supply chains and augments those models to yield more accurate and refined results.”
Such a capability can also be used to help to train new workers in a facility and reduce their errors substantially, especially if the AR is used to train the AI by learning directly from the actions and decisions of experienced workers. This does not eliminate the human from the job, as a human is still necessary to physically execute the task. But AI could learn the most effective manner to execute a task and then guide a novice through a similar procedure using AR. All this can be done while building the product’s digital passport, conducting time and motion studies, and ensuring that all safety protocols are followed. It is truly an approach that augments and adds value to the workforce.
Privacy Implications
If we are recording every move and motion of the workforce, this does raise some privacy concerns which will need to be addressed in the years ahead. We will need to understand what we are learning and recording from the workforce and ensure that their data are protected accordingly. This is not unlike Google maps. We share our location with Google, and in turn, Google provides us with directions to where we want to go. Of course one major concern here is that Google might use our location data to track us and provide that information to third parties. The same is true in manufacturing. How do we ensure privacy among workers and businesses? This does open up a can of worms as, right now, different geographic regions (e.g., EU, U.S., China) have different regulations regarding personal data and its privacy. So, we will certainly have to address these issues which could become increasingly complex for multinational companies over the next few years.
Furthermore, the ability to share production data from one production facility to other facilities, or from one machine tool to another, is going to become important as lessons learned in one location could be equally as valuable to others at different locations. This highlights the value of data, but it begs the question, why would factory owners open their cyber doors to others and share their data? Clearly, new business and privacy models and rules must be developed to make it both worthwhile and safe for companies, and industries, to share some of their data.
Looking Ahead
So, I believe that by 2030 we are going to see AI capabilities permeate throughout manufacturing. We are also going to see it really take off in mixed reality settings that will increasingly look like something out of a science fiction movie. This will result in a safer, more efficient, and higher value-added manufacturing ecosystem. But it will also result in some very different business and socio-economic models that will change the way manufactures operate, think, and profit in the years ahead. M
About the author:
Professor Thomas R. Kurfess is Executive Director at the Georgia Tech Manufacturing Institute, Professor and HUSCO/Ramirez Distinguished Chair in Fluid Power and Motion Control at the George W. Woodruff School of Mechanical Engineering at the Georgia Institute of Technology, and a member of the MLC’s Board of Governors.
Will AI Enable Autonomous Plants and Factories?
Let’s Talk About AI event speaker, Dr. Hiroaki Kanokogi, shares how AI allowed Yokogawa to autonomously run a chemical plant for 35 consecutive days
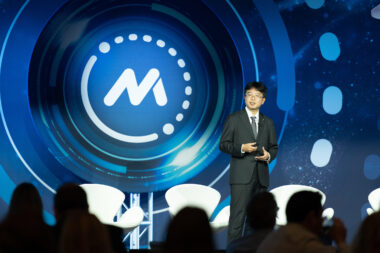
When toddlers learn to stack blocks, they learn by trial and error — often with immediate feedback from a parent or other adult. It is a model-free learning process, or reinforcement learning, that does not require them to learn Newton’s equation to figure out how to stack the blocks.
For Dr. Hiroaki Kanokogi, Yokogawa Digital Corporation’s President and CEO, reinforcement learning (RL) in artificial intelligence (AI) has direct uses in a manufacturing environment. In fact, as Kanokogi shared during the Manufacturing Leadership Council’s Manufacturing in 2030 Project: Let’s Talk About AI event, RL was a building block when Yokogawa used AI to autonomously control a Japanese chemical plant for 35 days earlier this year.
In his presentation at Let’s Talk About AI, Kanokogi shared that there are serious challenges to applying RL to real world manufacturing. First, he said, traditional RL takes 1 million to 1 billion trials to go beyond human learning, and second, manufacturers must include safety assurances.
To overcome the first of these challenges, Yokogawa and the Nara Institute of Science and Technology developed scalable reinforcement learning called Factorial Kernel Dynamic Policy Programming (FKDPP) specifically for plant control. FKDPP allows for faster learning (typically in about 30 trials) and robust protection against disturbances. Yokogawa was able to demonstrate that FKDPP can autonomously stabilize water levels in a fundamental three tank level control experiment significantly quicker than traditional proportional-integral-derivative (PID) control.
At Let’s Talk About AI, Kanokogi shared four videos that chronicled FKDPP’s iterative attempts to stabilize the water. In the first iteration, AI does not know anything yet, so when the valve is opened the water level goes all the way up. In the 20th iteration, AI can control the water in a somewhat stable manner, but it varies and resembles a human’s performance on the task. For the 25th iteration, AI learns how to regulate the variation. By the 30th iteration, the FKDPP perfects the process. Kanokogi pointed out that this final iteration demonstrated that once AI finds a good way, optimization of this process is AI’s strength.
For the second challenge around safety assurance, Yokogawa was able to prove AI can satisfy this need during this year’s 35-day autonomous factory operation. The company first built a good simulation model by using domain knowledge in a digital twin so the AI could learn. Step two called for simulation and evaluation using both past and live data. Finally, the company ensured safety and control in the actual plant using Yokogawa’s integrated process control system, CENTUM™ VP DCS.
For Yokogawa and its autonomous operation, Kanokogi reported that he and his team continue to look at problems in the factory where AI can be applied. While the first-of-its-kind, 35-day automation demonstration is truly impressive, he sees manufacturing working in an autonomous plan-do-check-act (PDCA) loop by 2030. This loop will run continuously, and AI will help the plant improve itself. While there is no need for human intervention during this loop, Kanokogi pointed out that AI cannot add new sensors or integrate new technologies, so human experts will maintain a defined role in manufacturing.
Like a toddler with blocks, autonomous factory operation might be in its nascent years, but with the help of AI and Yokogawa’s FKDPP technology, maturation by 2030 is possible.
Manufacturing in 2030 Project: Let’s Talk About AI was held Dec. 7-8, 2022 in Nashville, Tenn. The event was part of MLC’s Manufacturing in 2030 Project.
The Need to Accelerate Industrial AI Adoption By 2030
AI will become a key player in driving manufacturing competitiveness in the years ahead.
David R. Brousell, Co-founder of the NAM’s Manufacturing Leadership Council, called on all manufacturers to accelerate their understanding and use of new Artificial Intelligence (AI) technologies in his opening speech at the MLC’s latest Manufacturing in 2030 event, Let’s Talk About AI, in Nashville, Tenn., this morning.
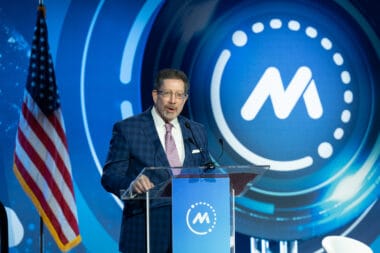
“The stakes for our industry and our country couldn’t be greater as our economy becomes increasingly digital,” asserted Brousell. “Global competition for dominance in AI is underway, with manufacturing as a key player in the race. Our competitiveness as an industry at home and abroad will increasingly be defined by AI expertise, application, and experience – and in a trusted and responsible way.”
Technologically, he noted, AI is finally coming into its own after a long development period and researchers now estimate that the value of the global AI industry will rise rapidly from $93.5 billion in 2021, to a substantial $1.8 trillion by 2030.
AI is also a pervasive technology, he continued, meaning that it will be incorporated into many other technologies including semiconductors, software applications and platforms, and communications equipment. It will increasingly power the operation of front-office applications, ERP, PLM, MES, CRM and other key operational applications. Robotic systems, too, will increasingly be guided by AI.
And although only 9% of respondents to a recent MLC study said that they saw AI and ML as a game-changer for the industry today, by 2030, 53% said that they believed it would indeed become a game-changing transformative force.
Citing a U.S. Patent Office report from October 2020 that stated, “AI is poised to revolutionize the world on the scale of the steam engine and electricity”, Brousell stressed that’s why manufacturers now need to better understand how AI may shape how they run their factories and plants, how it will influence their workforce strategies, what business benefits may attend AI use, and what challenges the industry must overcome to realize its potential in the years ahead.
However, “as with any important technology,” he added, “let alone one as unique as AI, there will be a learning curve replete with twists and turns.”
One of those twists is the fact that AI remains a controversial technology. Some see it as an existential threat to humanity; others see it unleashing a new wave of productivity and efficiency and enabling people to have better and more rewarding business lives.
Brousell also believes that AI’s unique ability to learn, and what that ability implies for predicting machine and operational patterns and behavior, qualifies it to be “in a special place among all of the technologies associated with digital transformation.”
“I can’t think of another technology that we have employed in our factories and plants,” he added, “that requires us to ask the question: do we need a code of ethics for AI use?” According to recent MLC research, he noted, 75% of manufacturing executives already believe a code of ethics will be needed in the years ahead.
Nevertheless, predicted Brousell “AI is here to stay”, and that its influence will only grow in operations, in the workforce, in the interactions across supply chains, and with customers and partners in the years ahead.
“AI”, he concluded, “is truly a force to be reckoned with” for the future of manufacturing, and manufacturers now need to act with urgency to accelerate its adoption to drive competitiveness in the years ahead.
Ten Takeaways from M in 2030 Project A Lens on the Future Panel
With the recent release of the Manufacturing in 2030 Project survey A Lens on the Future, MLC assembled an expert panel of speakers to discuss the results and bring context to the survey findings.
Moderated by David R. Brousell, the MLC’s Co-Founder, Vice President and Executive Director, the panel included Dennis McRae of West Monroe, Greg Wagner of EY, Chirag Rathi of Infor, and Joe Zakutney of NTT DATA.
Here are ten key takeaways from the discussion:
1. 84% of survey respondents expect an increased pace of digital adoption in the next decade
Dennis McRae, West Monroe’s Senior Partner and Practice Leader, Consumer & Industrial Products, noted that the acceleration rate depends on the size of the company, but he said the biggest thing is if you haven’t started your Manufacturing 4.0 journey in a meaningful way, now is the time to do it to maintain competitiveness.
“One of the first things you can do is really establish a team focused on digital with the right leaders, the right innovators, and also the right disruptors who can really challenge and do what leadership needs to get done,” McRae said.
2. 58% of survey respondents have autonomous factory operations on their radar by 2030
Chirag Rathi, Senior Director, Industry and Solution Strategy at Infor, pointed out that the desire for autonomous operations is not new and that General Motors was talking about this in the 1980s. Citing deep-learning and self-learning algorithms in machine learning, digital twins, blockchain, and autonomous transportation, he sees autonomous operation as a game changer, but he cautioned that full autonomy is unlikely in many circumstances.
“The cost of doing full autonomy in most industrial manufacturing processes might be too high,” he said. “So you will have part autonomy in several manufacturing arenas where the business case makes sense, but it will be a decision made on a case-by-case basis.”
3. 76% believe manufacturing should adopt an AI code of ethics
For Joseph Zakutney, NTT DATA’s Vice President, Manufacturing Industry Consulting and Digital Transformation, thinking about AI’s future means protecting against biases and cyberattacks, while accounting for safety.
“Procedures will need to be put in place to make sure that we’re complying to [a code],” he said. “We need to make sure that the software that we are releasing is fair, reliable, explainable, takes data protection and government regulations into consideration, and is focused on the well-being of society.”
4. Almost half of respondents indicated they expect workforce shortages to continue through 2030
Traditionally, manufacturing doesn’t have the best image according to Greg Wagner, EY’s Data Driven Manufacturing Leader. It is considered dirty, loud, and can be physically intense at times, but Wagner pointed out that those seeking purpose-based work should be attracted to manufacturing. The old adage of “being a cathedral maker and not a bricklayer” fits here, according to Wagner.
“If we change the paradigm and what we’re looking for, the types of job experiences we can give people, and use automation to get rid of some of those menial tasks that people don’t enjoy and free up their capacity to focus on bigger problem solving, it will mean more impactful types of roles,” Wagner said. “That’s going to really start to attract people and start to soften some of that gap we see right now in hiring.”
5. 81% of manufacturers are looking for greater speed and flexibility
When we think about speed and flexibility, what people really want is responsiveness, according to Wagner.
“If we really want to be able to respond quicker, we need to know what’s going on and we need to invest in better end-to-end visibility of what’s happening within our factories and what’s happening across our network so that we can be more adaptive and have the right insights to drive that change,” he said.
McRae added, “There’s a big opportunity for manufacturers in terms of connecting with their customers, building that client experience, and really monetizing a lot of the data that’s already in the business.”
6. By 2030, 50% of respondents believe digital adoption will be a game changer.
For Rathi, we’ll be closer to Industry 5.0 by 2030 with hyper-customization, responsive and distributed supply chains, and business model innovations. In fact, Rathi said we already have the building blocks to make this a reality.
“We have certainly got a lot of the raw materials to make that transformation happen,” he said. “So we will have a lot of transformative changes by that time period.”
7. Digital acumen is important across functions and at various leadership levels
One challenge McRae sees is getting everyone on the same page using the same language so that data assets are understood across company levels by all who manage data and products in the business.
“It’s not just around different levels, but also across functions,” added Wagner, noting that there is a wide array of digital understanding and how those technologies can be applied.
8. Hiring for a digital background versus a subject matter expert depends on the project
“I think we’re seeing the rise of data scientists and citizen data scientists at some organizations. At the same time a lot of data DIY products are becoming available, and they will become more prevalent by 2030, meaning that people with subject matter expertise will be able to basically design and develop their own data science projects,” Rathi said. Because of that, he believes subject matter experts will be in higher demand in most cases vs. data scientists.
9. For companies slowing down their digital projects due to the economy, focusing on specific things can help prevent losing ground
“Digital adoption is really a people play right now,” said Zakutney. “I’d stay focused on people and process, because ultimately, that’s what you’re going to end up automating [when funding comes back].”
“You can’t quit your digital investments,” added McRae. “If you don’t become digital, you’re going to be left behind. At the same time, prioritizing those digital investments specifically around areas that are going to improve your customer experience and take costs out over the next few years are going to help you win.”
10. Beyond the panel discussion, the survey report provides insightful data about the future of manufacturing
The MLC’s Manufacturing in 2030: A Lens on the Future research survey includes front-line insights from over 260 senior manufacturing industry executives, spanning multiple functional roles, and representing large-, medium-, and small-sized manufacturing companies from multiple industry sectors. Armed with this rich combination of real-world predictions and forward-thinking understandings, the MLC hopes that manufacturers can better plan their longer-term future and find ways to enhance their value, competitiveness, and contribution to society. Download the complete survey data and report.