Welcome New Members of the MLC February 2025
Introducing the latest new members to the Manufacturing Leadership Council
Euan Anderson
VP of Manufacturing – KCNA
Kimberly-Clark
https://www.kimberly-clark.com/en-us
https://www.linkedin.com/in/euan-anderson-39316547/
Stacy Brozen
Director of Continuous Improvement NA
CNH Industrial
https://www.cnh.com/
https://www.linkedin.com/in/stacy-kelley-brozen/
Tim Windingstad
Director of Manufacturing – Fabrication
Hatco Corporation
https://www.hatcocorp.com/en
https://www.linkedin.com/in/tim-windingstad-04446b198/
Troy Youngbauer
COO
Galloway Company
https://www.gallowaycompany.com/
https://www.linkedin.com/in/troy-youngbauer-8a9012b/
Dialogue: Finding the Human Edge in Manufacturing AI
Dow’s Tim O’Neal shares how data, AI, and intentional talent placement drive operational excellence
Penelope Brown, MLC: Well, hello, everybody, and thank you for joining us for the February 2025 edition of Executive Dialogue, and today we are super excited to be talking to Tim O’Neal of Dow. He is their Global Director of Operational Excellence and Leveraged Services, and he is also one of the Manufacturing Leadership Council’s newer members of our Board of Governors. We’re really excited to have him on board and to get to know him a little bit today. So, Tim, thank you for joining us.
Tim O’Neal, Dow: Thank you for having me. I appreciate the time to talk.
PB: Let’s talk a little bit about you. Get to know you a little bit. I know you’ve been with Dell, you know, for a while. What’s your career evolution been like there? And what’s your current role? What does that entail?
TO: I’ve been with Dow 28 years. It’s going on a quick 29 years coming up soon. But I’m currently the Global Director of Operational Excellence and Leveraged Services. My role is really to support our businesses and our plant assets through best practices, like quality, continuous improvement, digital, environmental and analytical services, external manufacturing and including operations, talent management like recruiting, onboarding and with learning and talent development as well.
I began my career in 1997 in Seadrift, Texas as an environmental protection engineer. From that role I quickly moved into direct manufacturing, and I progressed through the manufacturing journey with roles in multiple technologies and production plants.
I was blessed to lead large world scale plants as well as the smaller batch facilities, where you can switch products and create different products within hours and not days. I was the site director before moving into a global environmental health safety and sustainability role to drive our corporate world leading operations, sustainability goals.
And you and I 1st met when I became the digital operations, IT/OT director responsible for all the manufacturing digital teams, and from that role I then joined this global director role in operational excellence.
PB: So let’s pick up a little bit. I know you just mentioned part of it is recruiting and that talent development piece. You know, really improving performance of both people and processes through that continuous learning and building those effective teams. What do you think, is the most important thing for leaders to know when it comes to encouraging team members to really perform at their best?
In operations and manufacturing, we can measure everything, but you want to make sure that you’re measuring those items that can lead to actions, whether it’s defects, outliers, and trends.
TO: To me, it’s always about placing talent and people intentionally. I see it almost like, I think, three bubbles. There’s this intersection of skills, passion in terms of what people want to do, and then what the organization needs and values. If we take the time to understand our individual team members, that intersection is where discretionary effort is born which leads to performing at our best.
PB: Afew months ago you were on one of MLC’s Decision Compass calls. For those of you that may be watching this and not in the know, these are virtual working group meetings that we host.
You were discussing how Dow uses data to predict operational failure. Borrowing from that theme, how do you think the data can most easily be translated into action for manufacturers?
TO: First and foremost, I really think about measuring where it matters. In operations and manufacturing, we can measure everything, but you want to make sure that you’re measuring those items that can lead to actions, whether it’s defects, outliers, and trends. Early in my career a very experienced leader had mentioned to me one time and really gave me some great advice. He said, “Tim, manufacturing plants will whisper to you and let you know when something is not exactly right.”
And so our challenge is to really understand that and place a measurement system in place to detect the most probable failures in our facilities.
I believe in the future we will continue to see AI as a collaborative partner enhancing our capabilities and really helping us meet some of the dynamic challenges in changing marketplace in our manufacturing industry
PB: Borrowing some from that data theme, we’re hearing a lot of that from our members. It’s been an ongoing struggle to get data to a really usable format. But a lot of our members and the manufacturing community at large is really trying to get their data in shape to start using AI right? So you want to have that data in a good position to be able to feed into those algorithms and give you those actionable insights.
There’s a lot of discussion about that growing role of AI within manufacturing operations. Looking toward the future, do you see AI mostly staying in a role as strictly a digital tool? Do you see it becoming a collaborative team member? Or do you see it potentially moving into what might be considered a leadership role?
TO: First, we have a long history in our industry of AI in the early forms, if you think about machine learning, automation and optimization. However, we are in the earlier learning stages of our Generative AI journey. I see AI’s role in manufacturing and decision making as multifaceted, and it’s continuing to evolve.
We envision AI, not just as a digital tool, but an integral part of our team helping us drive efficiency and innovation. I believe in the future we will continue to see AI as a collaborative partner enhancing our capabilities and really helping us meet some of the dynamic challenges in changing marketplace in our manufacturing industry.
PB: Yeah, it’s an exciting time. And I’m really quite keenly interested to see where this all ends up going in the next 3, 5, 10 years. We’ll really see what happens there.
Talking again on that AI theme, MLC just in December, hosted our Future of Manufacturing Project event down in Orlando and we had an AI theme around that. And one of our panel discussions talked about how AI might impact decision making specifically. For example, where that decision is made such as within maybe a functional role, or why it was made based on the type of decision. What types of decisions do you think AI is going to end up influencing the most?
As long as we have reliable and governed data where folks understand that you have one source of truth, I believe that’s where we’ll have the best decision-making capabilities.
TO: I personally believe it’s limitless and I’ll go back to your statement regarding data. As long as we have reliable and governed data where folks understand that you have one source of truth, I believe that’s where we’ll have the best decision-making capabilities. And it could be structured or unstructured data, but I think where AI really will help us make decisions is to make those trends and make the data very visible and be much more quicker to analyze, so that we can make sound decisions.
For our teams, the early areas where we’re piloting this is really in plant reliability, where you can make decisions about taking equipment down and doing maintenance in a timely manner. And I think this is where AI can look at multiple pieces of data and maybe give us a quicker glance at what could potentially be a failure in our, in our manufacturing facilities.
PB: Well, Tim, thank you so much for your time today. We are, again, very happy to have you on our Board of Governors, in addition to all the other insights that you share with us and share with our members.
Thank you and we hope to see you again soon.
TO: Yes, absolutely. Thank you for the time, and I appreciate a chance to catch up.
PB: Thank you. M
About the Interviewer:
Penelope Brown is Senior Content Director for the NAM’s Manufacturing Leadership Council
Survey: M4.0 Appears Poised for A Significant Leap
Manufacturers are ramping up digital investments, with AI, automation, and smart factories reshaping the industry faster than ever.
KEY TAKEAWAYS:
● Digital transformation is a game changer. Sixty percent see it as a defining shift for the industry, marking a strong rebound in enthusiasm after a dip in 2024.
● Manufacturers digital maturity continues to increase as 75% believe they are at a mid-level maturity—up significantly from the 2024 and 2023 survey results.
● AI expectations have rebounded. A full 80% now fully or partially agree that self-managing and self-learning facilities powered by AI and machine learning are on the horizon.
Manufacturers are charging ahead with their digital transformation efforts. The Manufacturing Leadership Council’s Smart Factories and Digital Production Survey reveals an increase in smart factory maturity and optimism about continued digitization and adoption.
Fueled by an expectation of economic growth and a corresponding plan to increase or maintain smart factory investments, respondents share an outlook that includes AI adoption, end-to-end digitization, and belief that the game changing power of digital transformation is moving beyond mere table stakes.
Section 1: Economic Outlook and Investment Trends
Manufacturers are optimistic about the economy in 2025, with 69% expecting moderate growth and no recession, while only 8% anticipate an economic slowdown. Encouragingly, 11% expect inflation to ease and growth to rebound, signaling greater confidence in market stability. (Chart 1)
This optimism extends to smart factory investments, with 89% of manufacturers planning to maintain or increase spending in 2025. Within that number, it’s notable that 44% expect to ramp up investments, aligning with a broader push for digital transformation. (Chart 2) In the 2024 version of this survey, only 9% expected to increase their Manufacturing 4.0 investment.
Meanwhile, only 8% foresee a decline in spending in 2025, underscoring that despite economic fluctuations, smart factory adoption remains a strategic priority. The data suggests that as confidence in economic growth solidifies, companies are doubling down on automation and digitalization efforts to remain competitive.
1. Strong Majority See Growth on the Horizon
Q: What is your company’s outlook for the economy in 2025? (Select one)
2. 89% see smart factory investments increasing or remaining unchanged
Q: How do you expect your company’s outlook for the economy to influence M4.0 smart factory and production technology investments for 2025? (Select one)
Section 2: Smart Factory Maturity and Adoption
The digital transformation journey continues to advance, with manufacturers steadily maturing their smart factory strategies. Compared to previous years, the percentage of companies rating themselves at lower maturity levels (1-3) has declined significantly, from 57% in 2024 to just 16% in 2025. Meanwhile, those ranking their factories at a mid-level maturity (4-7) increased to 75%—up from 42% in 2024 and 58% in 2023—and 9% now place themselves in the advanced range (8-10). (Chart 3)
While progress is evident, most companies are still in experimental phases—40% are piloting small-scale projects and 6% are implementing on a single-project basis. Meanwhile, 34% are scaling these initiatives company-wide. Only 15% are just beginning their digital journey, emphasizing a strong industry-wide shift toward implementation. (Chart 4)
In fact, only 28% believe their factories are very smart or somewhat smart today. That number is expected to skyrocket to 76% by 2027. (Chart 5)
For this to happen, specific functional areas must advance. So it is a positive that manufacturers aspire to reach advanced adoption in every area included in our survey. Many find themselves in the intermediate range, with procurement/inventory management the area most ripe to move from early adoption to this stage. (Chart 6)
Despite this, full integration with business strategy remains a work in progress, with just 24% rating their integration at eight or higher on a 10-point scale, though this is an improvement from 7% in 2024. (Chart 7)
3. Smart factory journeys continue to mature
Q: How would you assess the maturity level of your smart factory journey? (Scale of 1-10, with 10 being the highest level of digital maturity)
4. Most experimenting with small-scale pilots or scaling smart factory practices
Q: Which activity best describes the primary stage of your company’s M4.0 digital efforts today? (Select one)
5. Smart factory explosion expected by 2027
Q: How “smart” do you consider your factory and plant operations to be today and what do you anticipate they will be by 2027?
6. Manufacturers still striving to reach advanced digital adoption stages
Q: At what stage of digital adoption are the following functions in your company? (Rate as early, intermediate or advanced)
7. Smart factory strategy becoming more integrated with business strategy
Q: To what extent has your smart factory strategy been integrated with the company’s overall business strategy? (Scale of 1-10, where 10 is fully integrated)
Section 3: Digitization and Automation Growth
Manufacturers anticipate a significant leap in factory-wide digitization by 2027. Today, only 2% report being extensively digitized, but this is expected to surge to 38% within the next two years. Similarly, partial digitization is set to grow from 47% to 52%, while the number of companies still in planning stages will plummet from 43% to just 5%. (Chart 8)
Production and assembly processes are on a similar trajectory. Only 6% of companies currently consider these processes extensively digitized, but this figure is projected to jump to 45% by 2027. (Chart 9)
Integration with customers and suppliers, however, remains a weak spot—just 2% report extensive digital connectivity today, though 22% expect to achieve this by 2027. (Chart 10)
Taken together, these findings highlight a growing recognition of end-to-end digital transformation as essential for operational agility and supply chain resilience, while identifying an opportunity for improved digitization between manufacturers and their customers and suppliers.
8. Significant end-to-end digitization on the horizon
Q: To what extent are your factory operations fully digitized end to end today, and what do you anticipate they will be by 2027?
9. 92% predict extensive or partial digitization of production/assembly by 2027
Q: To what extent are your production/assembly processes digitized today, and what do you anticipate they will be by 2027?
10. Digital integration with customers and suppliers lags behind in-house digitization
Q: To what extent are your production functions digitally integrated with customers and suppliers today, and what do you anticipate they will be by 2027?
Section 4: Future of Factory Models and AI-Driven Operations
Manufacturers envision a hybrid future for their factory networks, with 44% expecting a mix of small and large facilities, reflecting the need for both localized agility and economies of scale. Large-scale production remains dominant, with 40% favoring large factories or networks of large facilities, while only 10% see a shift toward smaller, more agile production models. (Chart 11)
AI-driven automation is also gaining traction. Almost one quarter (23%) of manufacturers fully agree that factories will evolve into self-managing, self-learning facilities, a dramatic increase from just 6% in 2024. Another 57% partially agree, suggesting growing confidence in AI’s ability to optimize operations. This shift reflects the industry’s increasing focus on AI-driven decision-making and predictive analytics as key enablers of the smart factory vision. (Chart 12)
11. Only 10% see small, agile factories and networks as the future
Q: What is the expected future state of your factory model?
12. Expectation of self-managing, self-learning factories increase significantly
Q: Thinking about the impact of technologies such as AI and machine learning, to what extent would you agree or disagree with the following statement: “Tomorrow’s factory will evolve to be a self-managing and self-learning facility.”
Section 5: Technology Adoption and Priorities
The 2025 survey highlights strong momentum in advanced technologies, particularly cybersecurity (73% scaling/at scale), IIoT sensor networks (46% scaling/at scale), and advanced analytics (56% scaling/at scale). AI adoption is also accelerating, with 22% of manufacturers now scaling/at scale with AI solutions, and another 42% piloting at least one AI project. Machine learning follows a similar trend, with 21% scaling/at scale and 29% planned for future deployment by 2027. (Chart 13)
AI’s perceived impact is rising sharply—34% now see it as very significant, compared to just 10% in 2024. (Chart 14) This signals a shift from experimentation to real-world application, as manufacturers increasingly leverage AI for predictive maintenance, process optimization, and adaptive automation. However, emerging technologies like the metaverse, blockchain, and exoskeletons remain niche, with over 70% of companies having no plans to adopt any of them. (Chart 13)
13. Cyber, advanced analytics, IIoT sensor networks top production operations tech list
Q: Where does your company stand in regard to the following technologies in its production operations?
14. AI’s expected significance increases
Q: Looking ahead over the next few years, how significant an impact will AI have on your production operations?
Section 6: Challenges and Benefits of Digital Transformation
Manufacturers continue to grapple with major roadblocks in their smart factory journeys. Nearly half (49%) cite outdated legacy equipment as their top challenge, up from 39% in 2024, highlighting the complexity of modernization. Workforce-related barriers are also growing—43% point to a lack of skilled employees, a sharp increase from 24% in 2024. Resistance to change remains a persistent issue, with 42% citing organizational culture as a challenge, though this has improved from 53% last year.
But it’s not all bad news. In 2024, a lack of leadership buy-in and access to adequate budget/investment both ranked among the top four roadblocks. Improvements have been seen in both these issue areas. Leadership buy-in and increasing budgets may very well prove to be leading indicators that the current issues discussed above may become lower hurdles rather than roadblocks. (Chart 15)
Despite these current roadblocks, companies recognize the benefits of transformation. Nearly two-thirds (63%) rank operational efficiency as the top expected gain, a significant jump from 40% in 2024. Better decision-making (49%) and cost reduction (44%) follow closely, demonstrating that digital adoption is increasingly seen as a performance and profitability driver. (Chart 16)
Notably, the percentage of companies expecting digital transformation to create a competitive advantage has soared from 11% in 2024 to 38% in 2025. While the majority still see digital transformation as table stakes, the dramatic increase in those who see it as a competitive advantage marks a major shift in strategic thinking. This shift suggests that as adoption accelerates, digital capabilities are becoming key differentiators rather than mere necessities for survival. (Chart 17)
15. Legacy equipment, lack of skilled employees and organizational culture are biggest smart factory roadblocks
Q: What do you feel are your company’s primary roadblocks to implementing your smart factory strategy? (Select top 3)
16. Better operational efficiency outpaces all other smart factory benefits
Q: What are the most important benefits and opportunities your company hopes to realize from embracing a smart factory strategy? (Select top 3)
17. Digital transformation still seen as table stakes for majority, but increasing number see it as a competitive advantage
Q: Do you believe that digital transformation of your company’s manufacturing operations will create a unique competitive advantage for your company or is it merely table stakes to remain in the game?
Section 7: The Strategic Value of Digital Transformation
After a dip in enthusiasm in 2024, manufacturers now overwhelmingly see digital transformation as a game-changer—60% believe it signals a new era for the industry, up from just 26% last year. Another 40% still see it as significant but not transformative, while no respondents consider it insignificant. Apart from our 2024 survey, these numbers have remained largely consistent over the past four years. (Chart 18). M
18. After dip in 2024, majority now see digital transformation as a clear game changer
Q: Ultimately, how significant an impact will digital transformation have on the manufacturing industry?
About the author:
Jeff Puma is content director at the NAM’s Manufacturing Leadership Council.
Enabling Transformation with Smart Operations
Increasing visibility and speed of execution through Manufacturing 4.0 and connected systems are critical to meet manufacturers’ needs.
TAKEAWAYS:
● Manufacturers need digital tools and capabilities to address the increased business volatility that may impact their success.
● The key to success is how quickly manufacturers can identify and address the array of problems and opportunities that arise.
● Software systems that once enabled business transformation may now be a constraint, so manufacturers need updated technologies.
Manufacturing companies are continually balancing capability with opportunity. At the highest level, the challenges that manufacturers face today are the same as they’ve always faced—namely, how to deliver the best product or service at a cost that allows them to make a profit and invest in future opportunities. The new challenge manufacturers are now facing is how to address the significantly increased business volatility that can impact their success. Rapidly changing market dynamics, regulatory changes, technology advances, resource constraints, nationalism, and competitive pressures are forcing companies to react faster than ever before just to stay competitive. While many of these factors are not new problems, and every problem can be solved, developing an effective solution for addressing them simultaneously can seem like an unsurmountable challenge.
The key for success is how quickly manufacturers can identify and address the array of problems and opportunities that arise. Companies need to mitigate quality problems discovered in the field before they impact customers, margin, and future sales. They also need to identify potential shortages before they affect production. Manufacturers need to identify customer opportunities with material sourced before they lose out to the competition. They need to mitigate potential machine failures before they impact production. Moving too slowly can often make the solution worse than the problem. For example, building up too much inventory to address a shortage as the market starts to cool adds inventory and potential obsolescence costs.
Manufacturers may struggle to have the visibility they need to identify opportunities and challenges quickly. Manual processes and disconnected systems not only make visibility difficult, they can also make the rapid analysis and remediation required painfully slow. In such instances “heroes” are born: they make a phone call, call in a favor, work endless overtime hours, pay expediting costs, or throw additional resources at the problem to get to a resolution. These workarounds can be costly, cause disruption, and are normally not sustainable for long periods of time.
“Connectivity enabled through modern technology, strong organization, and innovative leadership enables a manufacturer to respond faster and more efficiently.”
Manufacturing 4.0 promises to help address these issues. Connectivity enabled through modern technology, strong organization, and innovative leadership allows a manufacturer to respond faster and more efficiently. Digitalization of the business provides the path to minimize the lag between the discovery of problems and execution of solutions. Optimizing the entire business requires a connected enterprise. This connection enables the visibility required to execute faster. Connecting engineering to manufacturing with a common product master enables faster and more accurate execution. Enabling a seamless flow between product engineering, manufacturing, quality, suppliers, supply chain, sales, and service enables digital execution between business processes. Looking at the business holistically as a connected enterprise enables smart operations.
With the advent of artificial intelligence (AI) and the dependency on data for better results, data availability and quality are becoming ever more critical. While manufacturers have been using predictive AI for years to predict machine failure and optimize production, they’re now using generative AI to improve quality and manufacturing efficiencies. To maximize the benefits and opportunities of AI for manufacturing, the next step will be to bring the operational technology data (OT) and back office/information systems (IT) data together so that correlations, predictions, and recommendations can be more accurate. Having a common platform that brings these data together enables an operator, supervisor, or maintenance technician to have visibility of their business and make better decisions faster. Persona-based workbenches enable the user to obtain the exact information required at the time required. The objective is to provide the information and tools necessary to better understand “their business” and be able to execute as required. The result will be users that are more productive, capable, and accountable to the business.
For many, software systems have served as a critical enabler of this business transformation, but they can also sometimes impede further progress on the journey. This has been the case for manufacturers that have selected systems that optimize specific areas of the business without taking into consideration the holistic need for a complete business transformation. Disconnected systems and complex integrations that once enabled a business may not have the capabilities and functionality needed to support new business models or adapt to rapidly evolving business requirements. With the immense effort required to make changes, IT and the systems that support them have often become a business constraint rather than an enabler. Modern cloud native enterprise applications have helped to address these issues by providing integrated platforms that are also updated continually. Manufacturers can take advantage of newly embedded capabilities and technologies such as AI as they evolve without requiring a reimplementation.
While most rightfully see Manufacturing 4.0 or Industry 4.0 as a journey vs. a destination, many companies feel as though they have not progressed as much as they would like. The World Economic Forum found that more than 70 percent of companies that invested in Industry 4.0 projects failed to get the benefits anticipated. Why is this so difficult? What can be done to increase success?
“Success is determined by how much time and what level of resources manufacturers need to identify any problems or opportunities and be able to adequately address them.”
The challenge for many companies is that their Industry 4.0/digitization projects are managed in silos. Similar to an assembly line or manufacturing constraints shared in Eliyahu Goldratt’s book The Goal, only optimizing a part of the business implies that the business is still constrained. The real value comes with connectivity between the lines of business. A manufacturer may find it useful to use real-time machine data to help predict machine failures and production progress to then manually create a maintenance order. However, using that data to have the system automatically order maintenance parts, plan a maintenance order, and adjust production schedules is even better. Being able to simultaneously and automatically notify the machine supplier so that they can improve their quality takes it even further.
How companies measure their Manufacturing 4.0 progress is another challenge. A snapshot in time is not necessarily the best indicator of digital transformation success. A successful transformation is really about the ability for a manufacturer to support constant innovation, continual improvement, and the capability to effectively address whatever challenges or opportunities that arise now or in the future. For these manufacturers, the better measurement is their ability to act, not just their performance improvement at any point in time. If they have transformed effectively, they have the visibility necessary across the organization, access to tools to analyze data quickly, and the capability to execute on that information. Success is determined by how much time and what level of resources manufacturers need to identify any problems or opportunities and be able to adequately address them. The ability to do so in the best way possible is a part of the Manufacturing 4.0 journey leading to smart operations and the ability for manufacturers to successfully address the ever-evolving business volatility. M
About the authors:
John Barcus is GVP Manufacturing Industries Strategy and Advanced Technologies at Oracle Corporation.
AI-Driven Factories of the Future: It’s a Lot More than Just Autonomy
The future of manufacturing is about intelligently blending autonomous operations, augmented intelligence, and flexibility to redefine production.
TAKEAWAYS:
● Manufacturers can achieve greater consistency and scalability in operations by leveraging AI, robotics, and digital twins.
● Fully autonomous factories are often more obtainable for large-scale manufacturers with high-volume, low-variation production.
● Small and medium-size companies will likely derive more benefits from targeted lights-out processes, known as dim factory operations.
Imagine a factory that operates seamlessly around the clock, with artificial intelligence (AI)-powered robots building products at an efficiency and precision unmatched by human hands. Supervisors step in only for exceptions, while AI ensures optimal performance and quality. The concept of a lights out (or dark) factory is often attributed to a 1955 science fiction story, “Autofac,” by author Phillip Dick.
As manufacturers worldwide invest aggressively in artificial intelligence (AI), the idea of fully autonomous dark factories is becoming increasingly viable. These facilities promise significant improvements in throughput, quality, safety and efficiency. Yet, while the enabling technology exists, only 15% of manufacturers have transitioned to fully autonomous operations. The future of manufacturing isn’t just about going dark; it’s about intelligently blending autonomous operations, augmented intelligence, and flexibility to redefine production. Lights out or not, the ideal AI-driven factory of the future will be Scalable, Automated, Serviceable, Intuitive (Accessible), Flexible, and Interoperable.
The Quest for Competitive Advantage
Dark factories — operating 24/7 with minimal human intervention — are often heralded as the pinnacle of manufacturing efficiency. By leveraging advanced AI, robotics and digital twins, among other advanced technologies, these facilities can achieve unparalleled consistency and scalability.
The global AI in manufacturing market is valued at over $5 billion in 2024 and is growing at a 38.5% CAGR. According to the International Federation of Robots, there are now over four million operational industrial robots in operation, nearly double the number since 2016, with installations surpassing 500,000 units annually. Ninety-six percent of manufacturers intend to continue to increase AI investments over the next five years, and nearly half (49%) have fully integrated AI-driven product and/or service changes into their capital allocation process.
However, fully autonomous factories are not a one-size-fits-all solution. They require significant capital investment, robust digital infrastructure and standardized processes — factors that are often more attainable for large-scale manufacturers with high-volume, low-variation production. The stakes have never been higher for garnering return on innovation investments.
The Promise of Going Dark
Dark factories are best considered as weapons of mass production. They are most suited for larger manufacturers that have a) steady, high volume production runs, b) largely standardized production processes, c) precise specifications and quality controls, d) highly digitized assets and robust digital twins/threads, and e) a workforce that is prepared to embrace change. These larger manufacturers can also benefit from economies of scale that will improve the return on investment. Several examples are:
- FANUC, a pioneer in lights-out manufacturing, has been operating an autonomous factory in Japan since 2001. Robots are building other robots (quite a dystopian-sounding concept!) at a rate of about 50 per 24-hour shift and can run unsupervised for as long as 30 days at a time.
- Linglong Tire’s new $990 million European factory started mass production in September 2024. It integrates cutting-edge technologies such as artificial intelligence, mobile sensing, industrial big data and industrial robots to achieve full automation — from the warehousing of raw materials to the delivery of finished products, greatly improving product quality and production efficiency.
- Philips’ factory in the Netherlands uses 128 robots to produce electric razors with minimal human intervention on certain shifts. There are only nine human quality assurance workers who oversee the end of the manufacturing process.
A More Practical Path: Dim Factories
For many manufacturers, especially small to mid-sized enterprises, the dim factory model offers a more viable alternative. By automating specific processes or shifts, these manufacturers can enhance efficiency, reduce costs, and address labor shortages without the need for full autonomy. This approach balances technological ambition with operational practicality, allowing for incremental improvements tailored to unique business needs.
Small and mid-size manufacturers and contract manufacturers typically deal with lower production volumes, higher product variety and more variable production demands. For these manufacturers, dark factory operations may be less practical or desirable. These types of manufacturers, as well as many large enterprises, would likely derive more benefits from targeted lights-out processes — or dim factory operations — vs. fully autonomous production operations.
Consider that manufacturers of all sizes that have modernized, AI-led processes achieve 2.5x higher revenue growth and 2.4x greater productivity. Process modeling and automation have also unlocked efficiency gains across end-to-end supply chains — reducing energy consumption by 22%, reducing inventory by 27% and reducing waste by 55%. Gartner research estimates that 60% of manufacturers will have more than two lights-out processes in at least one of their facilities by the end of 2025. The cost/benefit of a fully automated dark factory must be weighed against the value of these targeted, AI-driven process improvements.
As manufacturers invest in AI, the idea of fully autonomous factories is becoming increasingly viable.
Industry segments will also influence the potential benefits of AI-driven factory operations. For instance, customers in some industry segments (e.g., consumer products) are demanding a greater degree of customization or personalization. A recent survey showed that over 50% of consumers prefer personalized products and are willing to pay a slightly higher price for them. Manufacturers who deal with such demands may derive benefits from employing micro-factories, a concept that was first introduced in 1990 by the Mechanical Engineer Laboratory (MEL) of Japan. These smaller, agile, and highly automated micro-factories are capable of providing high-mix, low-volume customized products with a high return on investment.
Digital twins are becoming the backbone for AI-driven factories, providing a collaborative, connected information conduit across product design, production engineering, manufacturing execution, automation, and data-driven intelligence. Applications of digital twins have moved beyond simulation and modeling to automatically effecting manufacturing operation adaptations that maximize throughput and asset utilization, adjust to production variations, and mitigate impacts of unplanned disruptions.
Converting a traditional factory to a completely dark factory is a significant and capital-intensive undertaking. Brownfield factories have the burden of technical debt, siloed data architectures and repositories, a patchwork of IT and OT systems, and an abundance of non-digitized assets, among other challenges. These manufacturing facilities can still reap the incremental benefits of business-driven process automations as cited above. Conversely, new greenfield factories can incorporate digital and AI/automation from the onset without the additional cost and complexity of legacy systems, processes, and architectures. Indeed, the preponderance of the AI-driven manufacturing lighthouses are digital natives that benefit from those advantages.
The bottom-line is that while we are seeing automation and AI being deployed increasingly across the manufacturing sector, dark factories are far from a panacea. AI investments should always be aligned with business drivers and outcomes, not just technological feasibility or FOMO (fear-of-missing out). Business drivers for AI investments may may range from workforce and supply chain challenges, shifting consumer demands, economic and cost pressures, sustainability imperatives, necessary safety and quality improvements, and increasing global competition.
Human + AI: The Augmentation Advantage
Rather than viewing AI as a replacement for human workers, the most successful manufacturers see it as a partner. This shift toward “augmented intelligence” emphasizes collaboration, where AI enhances human decision-making and performance efficiency.
The manufacturing automation wave that was prolific at the turn of the century largely consisted of automating manual processes and workflows, resulting in a reduction of about 2.5 million jobs, according to Human + Machine: Reimagining Work in the Age of AI. We are now in an era of augmented intelligence, where automation and AI are working in concert with people to improve overall performance and output. Instead of replacing jobs, new jobs are being created in areas such as AI, data science, robotics, model-based systems engineering and related functions. Manufacturers investing in upskilling and reskilling their workforce are not just future-proofing operations but establishing a catalyst for innovation.
Major Challenges and Opportunities
Achieving the transformative promise of AI-driven manufacturing is not without its challenges. Manufacturers consistently identify five critical barriers: data quality and integration, cybersecurity threats, technical debt, workforce preparedness, and ecosystem complexity. These hurdles underscore the complexity of transitioning to AI-powered operations.
- Enterprise data management
AI thrives on high-quality, well-integrated data, but for many manufacturers, this condition remains elusive. AI applications are most often hampered by inadequate data quality (57%), poor data integration (54%), and weak data governance (47%). Only one in five manufacturers have production assets with data ready for use in existing AI models. The bigger the manufacturer, the greater the problem. Over half (52%) of manufacturers view a unified IT/OT data platform as essential for enabling industrial DataOps. Achieving this requires investments in modernizing data infrastructure, breaking down silos, and adopting scalable solutions. - Cybersecurity risks
Manufacturing is now the most targeted industry for cyber attacks, with operational technology (OT) bearing the brunt. Cyber-attacks have increased by 75% over the past five years. The manufacturing industry was the most targeted industry in 2023, and over 70% of cyber-attacks were OT related according to Zscaler Research. Ransomware costs are forecast to reach $265 billion by 2031, up from $20 billion in 2021. Recognizing these risks, 75% of manufacturers have made cybersecurity a top priority, and 70% of CEOs plan to increase investments in data, technology, and cyber defenses. Proactive strategies such as robust OT/IT integration and real-time monitoring are essential for safeguarding AI-driven operations.
“Dark factories are best considered as weapons of mass production.”
- Technical debt
Outdated systems and fragmented processes create technical debt that stifles innovation. Technical debt would require over $1.5 trillion to fix — and costs the U.S. $2.41 trillion a year in cybersecurity and operational failures, failed development projects, and maintenance of outdated systems. This technical debt also constrains interoperability of systems between engineering and design and the factory and between OT and IT. - Workforce preparedness
The human element remains both a challenge and an opportunity for scaling AI in factories. Hiring and training (upskilling and reskilling) remain among the top constraints to scaling AI in engineering, design, and factory operations. Seventy percent of executives are focused on reskilling/upskilling (vs. hiring) compared to 41% in 2020. In the future, every worker will become a knowledge worker and data literacy will be a foundational requirement. Knowing how to perform a process doesn’t mean a person understands the process or the potential enabling or constraining factors. Decision-making will be augmented by AI-powered insights, and employees at all levels will need to become more comfortable understanding and acting on data. To prepare for this future, manufacturers must embed continuous learning into their culture, equipping employees to collaborate effectively with AI systems. - Ecosystem partnerships
Although 69% of businesses are engaging actively in collaborative ecosystems, a large proportion (60%) believe that multisided partnerships are difficult to execute in practice. Poor strategic alignment with partners is the leading inhibitor of ecosystem strategies, along with other factors such as limited awareness of collaboration opportunities, a resistance to share data, and limited vendor understanding of business objectives. Speed and security are the top criteria when considering potential ecosystem partners, further echoing the need to efficiently scale solutions while addressing critical data governance and security requirements. Seventy-one percent of manufacturing CEOs who have high-performing partner ecosystems affirm that those ecosystems are providing greater growth opportunities than traditional M&A.
An AI Readiness Checklist
The journey to AI-driven manufacturing is complex, but with the right strategies, manufacturers can unlock unprecedented levels of efficiency, innovation, and resilience. Before embarking on the journey toward AI-driven manufacturing or dark factory operations, assess your organization’s readiness in these critical areas:
- Operational readiness: Are your processes standardized enough to benefit from automation? How diverse is your product portfolio? Have you effectively incorporated lean manufacturing principles? How scalable are your current operations? Where are your major bottlenecks?
- Technological readiness: Do you have digitized assets, robust data platforms, and integrated IT/OT systems? To what extent are you employing digital twins/threads (e.g., for products, factory, production, and performance)? How much technical debt is embedded in your current operations? To what extent are you using cloud/edge computing? What are your current AI/ML use cases and what are the impediments to scaling?
- Workforce and organizational readiness: Is your team equipped with the skills and cultural mindset needed to work alongside AI? Have you adopted a continuous learning mindset? How robust are your training, development and change enablement capabilities? Do you have leadership buy-in, commitment, and alignment with long-term strategy?
- Financial readiness: Have you accounted for initial capital investment, R&D, and recurring costs? What are your expectations for ROI and the payback period? Have you established KPIs to measure expected performance improvements? What is your risk tolerance? Are there government and/or tax incentives that can be leveraged to offset some costs?
- Security readiness: What is the extent of your network security and segmentation? How are you protecting your IT and OT systems against dynamic and increasing cyber threats? How robust is your data encryption? How are you addressing the vulnerability of the human factor? How are you addressing potential vulnerabilities across your supply chain? What training have you implemented for management and threat detection and deterrence?
- Data readiness: Do you have access to reliable, well-integrated data to support AI applications? Do you have someone who is clearly responsible and accountable for data management and governance? How mature are your enterprise data management processes? Have you accounted for the potential impacts (e.g., compliance, cost, risk) of evolving global policies and regulations regarding AI, data privacy and protection, and data residency?
- Enterprise readiness (e.g., Suppliers, Partners): How are you managing integration of third-party systems and data? How robust are your suppliers’ capabilities and what are the major risks? How effective are your ecosystem partnerships and relationships with software and technology providers? What is your strategy for in-sourcing/outsourcing aspects of your operations?
Closing Reflections
The factories of the future won’t just be automated; they’ll be adaptive, intelligent and collaborative. Future manufacturing operations will range from dark factories to dim factories to micro-factories, and employ digital twins and digital commercial manufacturing exchanges that will enable secure, agile, on-demand manufacturing production.
When determining how to best improve or transform your manufacturing operations, avoid a binary view that only considers function allocation between humans and machines (automation); also consider augmented intelligence and performance benefits from human + AI collaboration. Regardless of the level of automation and AI, it is imperative to keep humans in-the-loop as they will ultimately be held accountable for outcomes of actions and decisions.
For small and medium-size manufacturers, the dim factory model offers a viable alternative.
While AI has had periods of disillusionment (i.e., AI winters) due to over-hyped expectations and technical limitations, the conditions are quite different this time. Today, our world is significantly more digitized, sensorized, connected, and software-driven. Instead of a lack of real-time data, we now are challenged with deriving decision intelligence from a tsunami of data. The next generation of workers are digital natives who embrace, rather than fear, technology innovation. Accelerating technological advances in areas such as computing power and speed, cloud/edge computing, computer vision, generative AI, digital twins/threads will make AI-driven factories — including dark factories — more viable over the next five+ years.
The future is here; it’s just unevenly distributed. AI is already delivering value for many manufacturers, ranging from predictive maintenance to supply chain optimization to quality control. AI-driven robots, machine vision, and optimization algorithms are improving efficiency and flexibility. Addressing challenges related to cost, security, integration, data management, and workforce development will be crucial to unlock the full potential and widespread adoption of AI-driven factories.
Now is the time to take steps forward toward this vision — whether that’s injecting AI to automate specific manufacturing processes, improve human performance, or build a fully automated lights-out factory. Let’s shape the future together with confidence!
AI-driven factories of the future was the theme of the Manufacturing Leadership Council’s Future of Manufacturing Project event in December 2024. The EY organization is a member of the MLC. M
About the authors:
Brian M. Legan Principal, Ernst & Young LLP | Industrials and Energy Innovation Leader
Disclaimer: The views expressed in this article are solely those of the author and do not necessarily represent the views of Ernst & Young LLP, other members of the global EY organization or other organizations cited.
Unlocking Manufacturing Excellence with Digital Twins
Manufacturing leaders can leverage digital twin technology to drive operational transformation while ensuring sustainable, long-term value creation.
TAKEAWAYS:
● Manufacturing organizations must develop comprehensive data governance frameworks before implementing digital twins to ensure long-term success.
● Successful digital twin initiatives require cross-functional collaboration and clear stakeholder alignment across all organizational levels.
● Leaders should prioritize cultural transformation and workforce development alongside technical implementation to maximize digital twin value.
Manufacturing stands at a pivotal moment of transformation. As Industry 4.0 technologies mature, digital twins emerge as a cornerstone of operational excellence, bridging the gap between physical assets and digital intelligence. Yet, successful implementation demands more than technological capability — it requires a comprehensive strategy encompassing organizational readiness, data capabilities, and workforce development.
Building the Foundation
Digital twin success begins well before implementation. Manufacturing leaders must first assess their organization’s digital maturity and readiness for this transformative technology. This assessment should evaluate current capabilities, identify gaps, and create a roadmap aligned with broader business objectives.
A robust data strategy forms the cornerstone of this foundation. Without it, organizations risk several critical pitfalls:
- Data Quality Challenges: Incomplete or inconsistent data can lead to unreliable simulations and eroded stakeholder trust.
- Integration Complexities: Manufacturing environments often combine legacy systems with modern equipment, requiring careful integration strategies.
- Performance Issues: Without reliable, real-time data, insights may lack timeliness and relevance.
- Resource Inefficiencies: Poor data management often requires costly retroactive cleanup and standardization.
The Human Element
Technology adoption success relies heavily on cultural transformation. Leaders must recognize that implementing digital twins affects roles across the organization, from operations to maintenance and quality control. Clear communication about how these changes improve daily work and contribute to business success proves essential for adoption.
Cross-functional collaboration becomes particularly crucial. IT and operations teams must work closely to ensure accurate data capture and integration. Similarly, input from frontline workers who operate equipment daily can provide invaluable insights that enhance digital twin accuracy and utility.
Implementation Strategy
For mid-market manufacturers, successful implementation often starts with simplifying data integration. This might involve:
- Utilizing middleware solutions designed specifically for manufacturing environments
- Implementing basic but effective security measures
- Establishing regular calibration and update schedules
- Appointing digital twin champions to oversee integration and maintenance
The key lies in starting small while maintaining accuracy. Unlike other digital initiatives where “quick and dirty” prototypes might suffice, digital twins require precision from the outset to deliver value.
Stakeholder Alignment
Digital twin initiatives affect multiple organizational functions and stakeholders. Success requires clear value propositions for each group and effective communication channels. Beyond traditional meetings, organizations benefit from hosting workshops, particularly those focused on innovation, to align stakeholders around a shared vision. These sessions typically introduce participants to methodologies like design thinking while providing structured environments to identify solutions to pressing challenges.
Cross-functional workshops serve multiple purposes:
- Surfacing technical opportunities and cultural barriers
- Revealing differing priorities and potential resistance points
- Creating opportunities for greater departmental synergy
- Building shared ownership of the transformation process
Organizations should ensure these collaborative sessions translate into tangible outcomes through post-workshop initiatives such as pilot projects and regular follow-up meetings.
Future Considerations
The evolution of digital twin technology continues to accelerate. Integration with artificial intelligence promises enhanced predictive capabilities, while extended reality visualization could transform how teams interact with digital twins. Blockchain technology may soon ensure data integrity across complex supply chain networks.
To prepare for these advances, manufacturing leaders should focus on:
- Building cross-functional teams bridging operational and information technology
- Developing clear governance frameworks for data sharing
- Creating scalable architectures accommodating emerging technologies
- Establishing strategic technology partnerships
Digital twins represent more than a technological advancement — they offer a pathway to reimagining manufacturing excellence. As organizations embrace this journey, success depends on fostering a culture of continuous learning and adaptation. M
About the Author:
Jason Hehman is the Industry 4.0 Vertical Lead and Host of The Modern Industrialist podcast at TXI.
Harnessing Manufacturing 4.0: A Supply Chain Perspective
Manufacturing 4.0 technologies—including AI, machine learning, the Internet of Things, and cloud computing—can improve supply chain innovation and performance.
TAKEAWAYS:
● M4.0 technologies can process the vast volumes of data generated at every step of the production and distribution processes.
● Adopt a 4-part strategy: Implement cloud-based supply chain platforms; use the power of AI and predictive analytics; create a strong data integration framework; and manage change effectively.
● To accelerate the adoption of M4.0 technologies, start with a clear vision and roadmap, collaborate with technology partners, and focus on scalable solutions.
As manufacturers embrace Manufacturing 4.0 (M4.0) and the concept of “smart” manufacturing, many focus on the shop floor, implementing advanced technologies to improve integrated, efficient, and agile production processes. For smart factories to reach their full potential, supply chains must change to be equally intelligent and responsive. M4.0 technologies such as artificial intelligence (AI), machine learning (ML), the Internet of Things (IoT), blockchain, and cloud computing are changing supply chain management. These tools enhance operations by improving visibility, streamlining processes, and enabling real-time decision-making. Deploying supply chain solutions is complicated, however, due to challenges like system integration, legacy infrastructure, and the need for stakeholder alignment. This article identifies the most pressing supply chain challenges in the M4.0 era and offers recommendations for deploying M4.0 technologies to foster success.
Understanding the Complexity of Modern Supply Chains
Supply chains today are more complex than ever. They span global networks, involve different stakeholders, and are influenced by dynamic external factors such as geopolitical events, natural disasters, and shifting consumer demands. To make matters more difficult, data across the supply chain are often siloed within different departments and systems. With growing market pressures and increasingly complicated logistics, manufacturers are challenged by limited visibility, slower decision-making, and the need to balance cost-cutting with responsiveness to client demands.
This complexity is compounded by the integration challenges posed by legacy systems that have historically supported supply chain operations. For example, many manufacturers still rely on outdated enterprise resource planning (ERP) systems, transportation management systems (TMS), and warehouse management systems (WMS), which are often unable to communicate with new technologies or provide real-time insights.
“The challenge lies in managing these data effectively, integrating them from disparate systems, and using them to make informed, real-time decisions.”
In the era of M4.0, where data are generated at every step of the production and distribution processes, manufacturers must focus on how to seamlessly connect disparate systems and manage increasing volumes of data. This is where advanced technologies like AI, ML, the IoT, and cloud computing can be transformative for supply chain management.
Challenges in Supply Chain Management in the M4.0 Era
While many of these advanced technologies hold immense promise, manufacturers need to address four critical hurdles to ensure these technologies are adopted and deployed effectively in supply chains:
1. Complexity of interconnected systems
Supply chains involve multiple parties, including suppliers, distributors, retailers, and logistics providers. Each stakeholder may have its own systems, business processes, and levels of technological sophistication. This interdependence makes it difficult to achieve seamless integration. The lack of standardization in data formats and systems, along with the difficulty of aligning these varied systems and technologies, adds to the complexity.
2. Data complexity and integration
A key feature of M4.0 is the sheer volume of data being generated by sensors, devices, and machines. In supply chain operations, these data are spread across different nodes of the network—from suppliers’ warehouses to distribution centers to customers. The challenge lies in managing these data effectively, integrating them from disparate systems and using them to make informed, real-time decisions. Effective data analytics, predictive modeling, and ML algorithms are required to extract meaningful insights from these data, and they are dependent upon high-quality data.
3. Coordination across multiple stakeholders
The need for coordination between various external partners often compounds the complexity of modern supply chains. Information and logistics must flow smoothly across borders, companies, and systems. However, data privacy concerns, differing technological capabilities, and trust issues between stakeholders can hinder effective collaboration. Achieving true end-to-end visibility across the entire supply chain requires close alignment of business goals and technological frameworks.
4. Legacy systems and change management
A significant barrier to the adoption of M4.0 in supply chains is the prevalence of legacy systems. Many manufacturers still rely on older ERP, WMS, and TMS platforms that are not designed to work with modern cloud-based solutions or to handle the volume of data generated by connected devices. Transitioning to more advanced systems often requires significant investments in software upgrades, infrastructure, and training, which can slow down the adoption of M4.0 solutions.
Strategies for Deploying M4.0 Technologies
To address the challenges outlined above, manufacturers need to take a strategic approach when they deploy M4.0 technologies. Here are four practical recommendations for overcoming these challenges:
1. Implement cloud-based supply chain platforms
One of the most impactful ways to simplify the complexity of interconnected systems is by implementing cloud-based supply chain management platforms. These platforms can integrate data across multiple systems and provide real-time visibility into the entire supply chain. With cloud technology, manufacturers can centralize data from suppliers, warehouses, distributors, and retailers in a single system, making it easier to track inventory, shipments, and demand trends.
“One of the most impactful ways to simplify the complexity of interconnected systems is by implementing cloud-based supply chain management platforms.”
By using cloud-based platforms, manufacturers can be more flexible, work better with outside partners, and make sure that information flows smoothly across the whole network. Furthermore, these platforms often have built-in analytics tools that provide insights into performance metrics, enabling manufacturers to proactively manage supply chain risks and opportunities.
2. Use the power of AI and predictive analytics
The complexity of modern supply chains is further compounded by the need for real-time decision-making and the ability to predict future trends. AI and ML are transforming how manufacturers can analyze and use data. Predictive analytics can help companies identify potential bottlenecks, forecast demand fluctuations, optimize inventory levels, and improve logistics planning.
For example, AI-powered demand forecasting tools can analyze historical data and identify patterns, which helps businesses plan more accurately for peak demand periods or seasonal fluctuations. AI can also be used to optimize routes in transportation logistics, reducing lead times and transportation costs. By implementing these solutions, manufacturers can make more informed decisions, improve efficiency, and reduce costs across their supply chains.
3. Create a strong data integration framework
A critical step in implementing M4.0 solutions is ensuring that the systems and data sources are properly integrated. Manufacturers should invest in data integration technologies that can connect disparate systems and allow for seamless data flow. This includes integration between legacy systems and new, cloud-based platforms, as well as between internal systems and those of external partners.
The concepts of data governance and master data management are increasingly important. Ensuring data completeness, standardization, and integrity is required to ensure the effectiveness of data-enabled capabilities of M4.0.
4. Manage change effectively
Adopting new technologies in supply chain management often involves significant change, not only in terms of technology but also organizational processes and culture. Managing this change is crucial for ensuring successful technology deployment and adoption. Manufacturing executives should focus on the following strategies to ensure smooth implementation:
- Stakeholder buy-in: Engage key stakeholders—supply chain managers, IT, procurement, and finance—early in the process to gain their support. Ensuring alignment across the organization will increase the likelihood of successful adoption.
- Training and upskilling: Provide employees with the necessary training to operate new systems effectively. Equip the workforce with the skills to use data analytics tools, AI applications, and new supply chain management platforms.
- Iterative deployment: Rather than a big-bang approach, consider rolling out new technologies in phases, starting with a pilot program. This allows the organization to test solutions in real-world scenarios, measure their effectiveness, and make adjustments before full-scale implementation.
Best Practices for Accelerating Technology Adoption
To speed up the deployment of M4.0 solutions and maximize return on investment, manufacturers should adopt three best practices:
1. Start with a clear vision and roadmap
Before implementing any technology, manufacturers should define a clear vision for what they want to achieve with M4.0 in their supply chain. This vision should align with the company’s broader business objectives, whether that is reducing costs, improving customer satisfaction, or enhancing sustainability. A well-defined roadmap will outline the necessary steps, timelines, and milestones for deployment. This roadmap should be flexible enough to adapt to changes in technology and business needs over time.
2. Collaborate with technology partners
To ensure successful deployment, manufacturers should collaborate closely with technology vendors, consultants, and integrators. These partners can help navigate the complexities of system integration, provide industry-specific insights, and accelerate the adoption of best practices. By choosing experienced technology partners, manufacturers can reduce implementation risks and ensure that their M4.0 initiatives are aligned with current technological trends.
3. Focus on scalable solutions
Manufacturers should prioritize scalable solutions that can grow with their business needs. M4.0 technologies should not be seen as a one-time investment but rather as an ongoing journey. By choosing flexible platforms and technologies that can scale and adapt over time, manufacturers can future-proof their supply chains and ensure they are well-positioned to take advantage of emerging technologies and evolving market conditions.
Embracing M4.0 for Supply Chain Innovation
In conclusion, deploying M4.0 technologies in supply chain management offers manufacturers an opportunity to improve efficiency, enhance resiliency, and gain a competitive edge. The complexity of interconnected systems, data integration, stakeholder coordination, and legacy systems must be carefully managed to achieve success, however. By adopting cloud-based platforms, using AI, ML, and creating strong data integration frameworks and effectively managing change, manufacturers can accelerate solution design, deployment, and adoption. With a clear vision and strategic approach, M4.0 can further a new era of supply chain innovation and performance. M
About the author:
Michael Platz is a senior leader of the Supply Chain Consulting practice at NTT DATA.
Scenes from MLC’s AI Conference
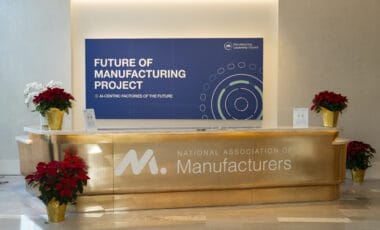
The opportunities and challenges of applying artificial intelligence in manufacturing operations were explored at MLC’s recent event in Orlando.
More than 190 manufacturing executives gathered in Orlando in early December of last year to talk about AI-Centric Factories of the Future. More than one dozen case studies, panel discussions, and presentations were delivered at the one-day conference, which was part of the Manufacturing Leadership Council’s Future of Manufacturing Project.
The goal of the Project is to help manufacturing executives understand the potential of advanced technologies to improve their operations in the years ahead.
The following are photos from the Orlando conference. MLC members can access full videos of the sessions depicted in the photos by going to the MLC Member Resource Center: https://manufacturingleadershipcouncil.com/member-dashboard/resource-center/resource_type/future-of-manufacturing-project/
Photos by David Bohrer, Senior Director, Photography, at the NAM.
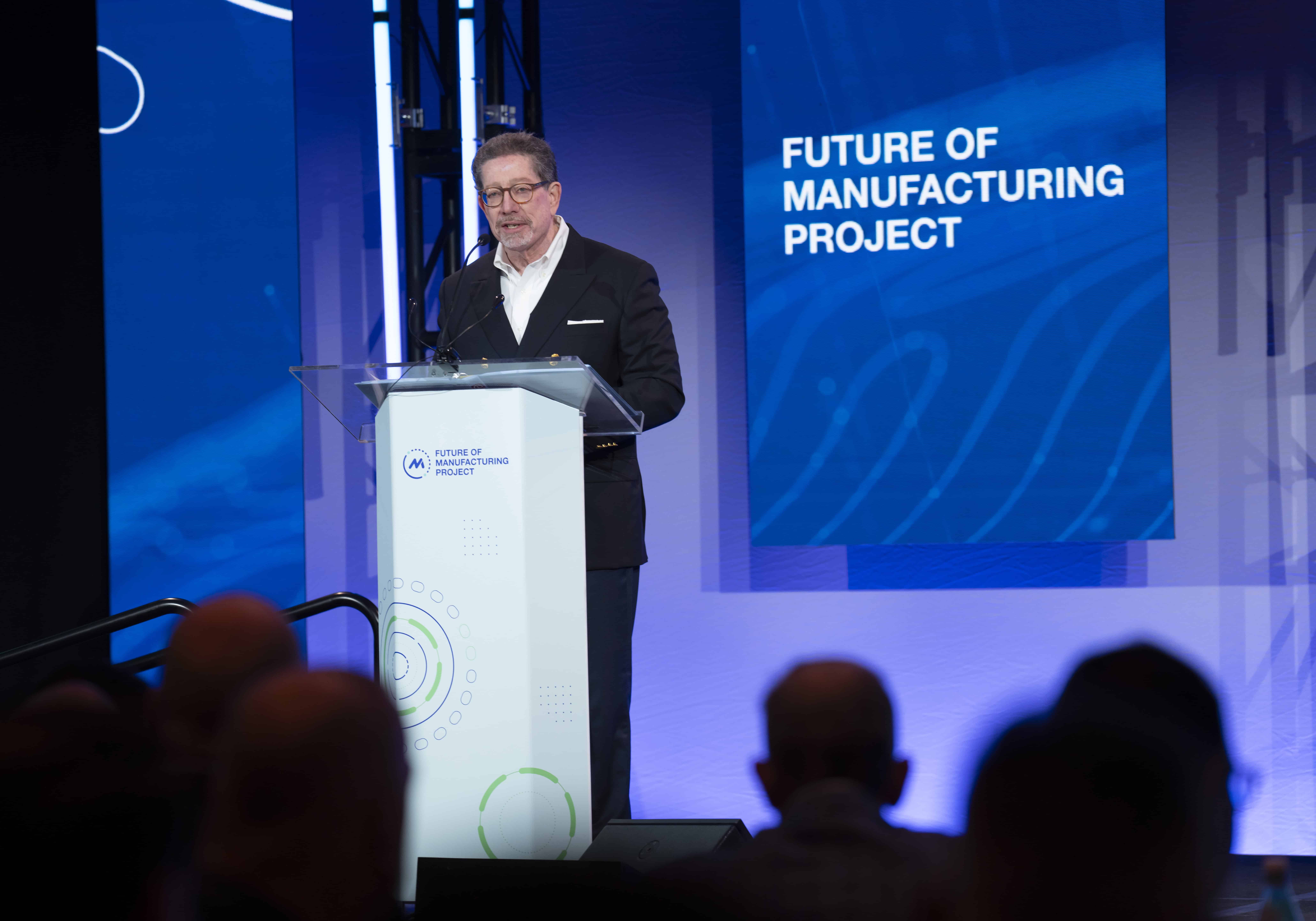
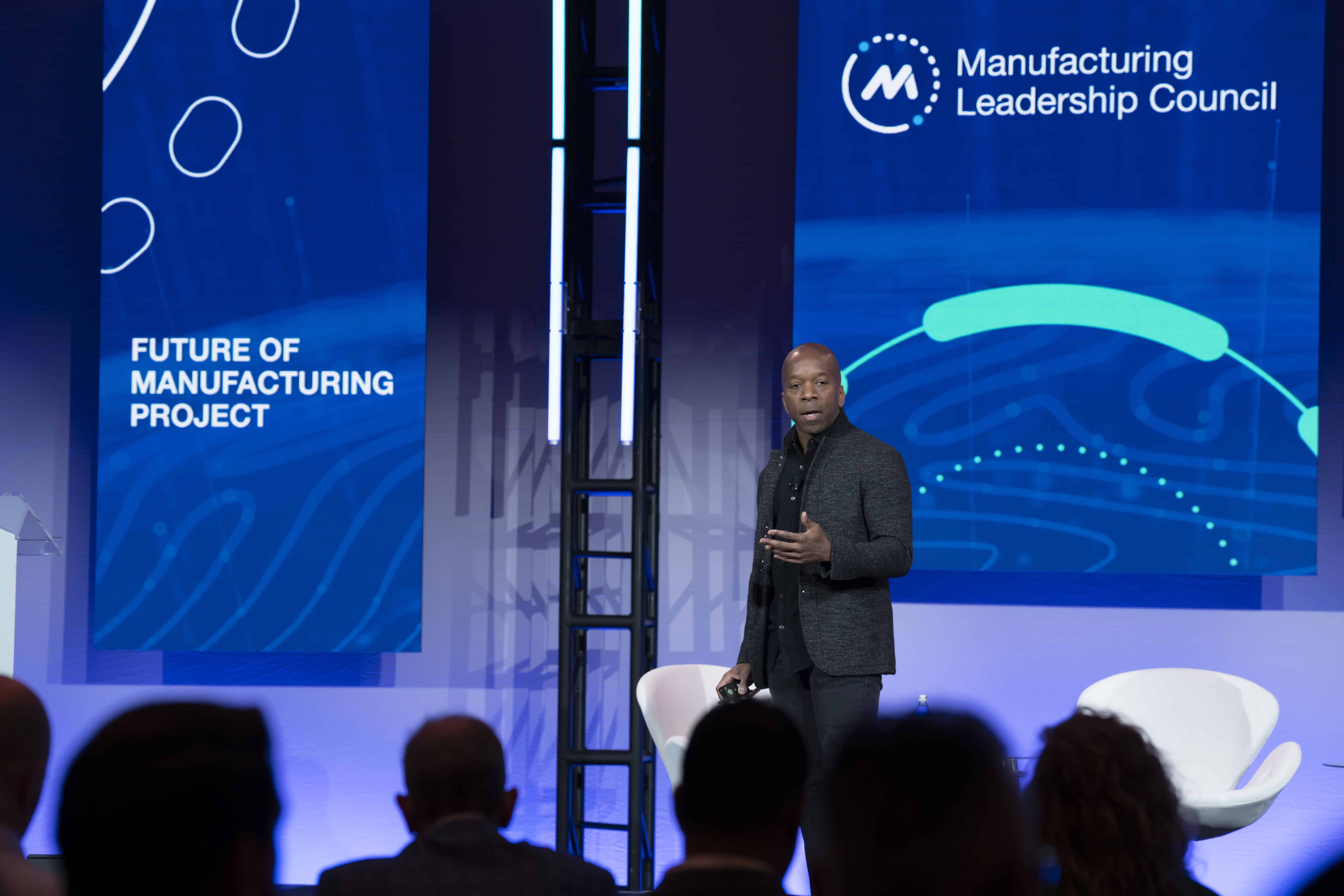
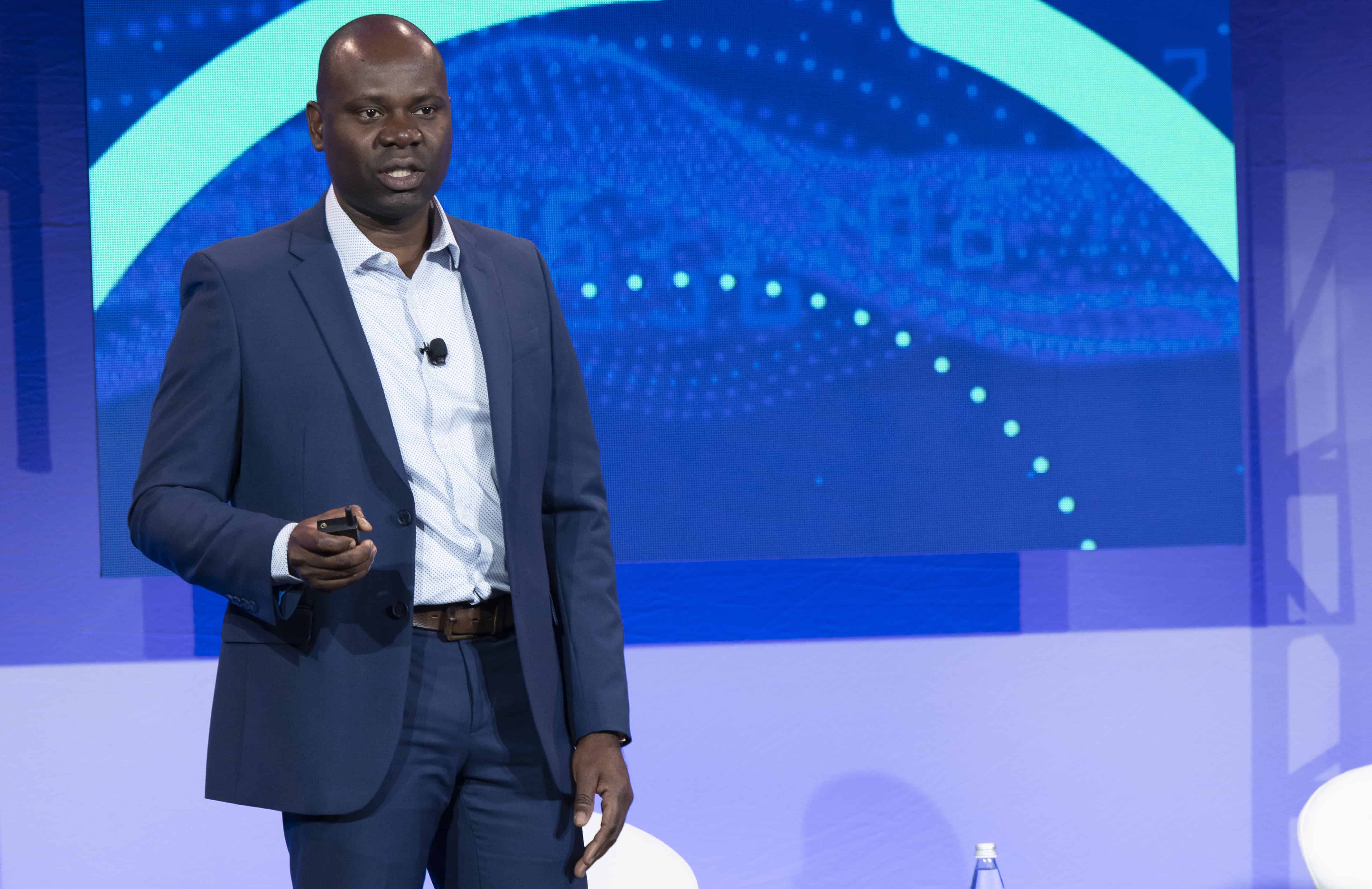
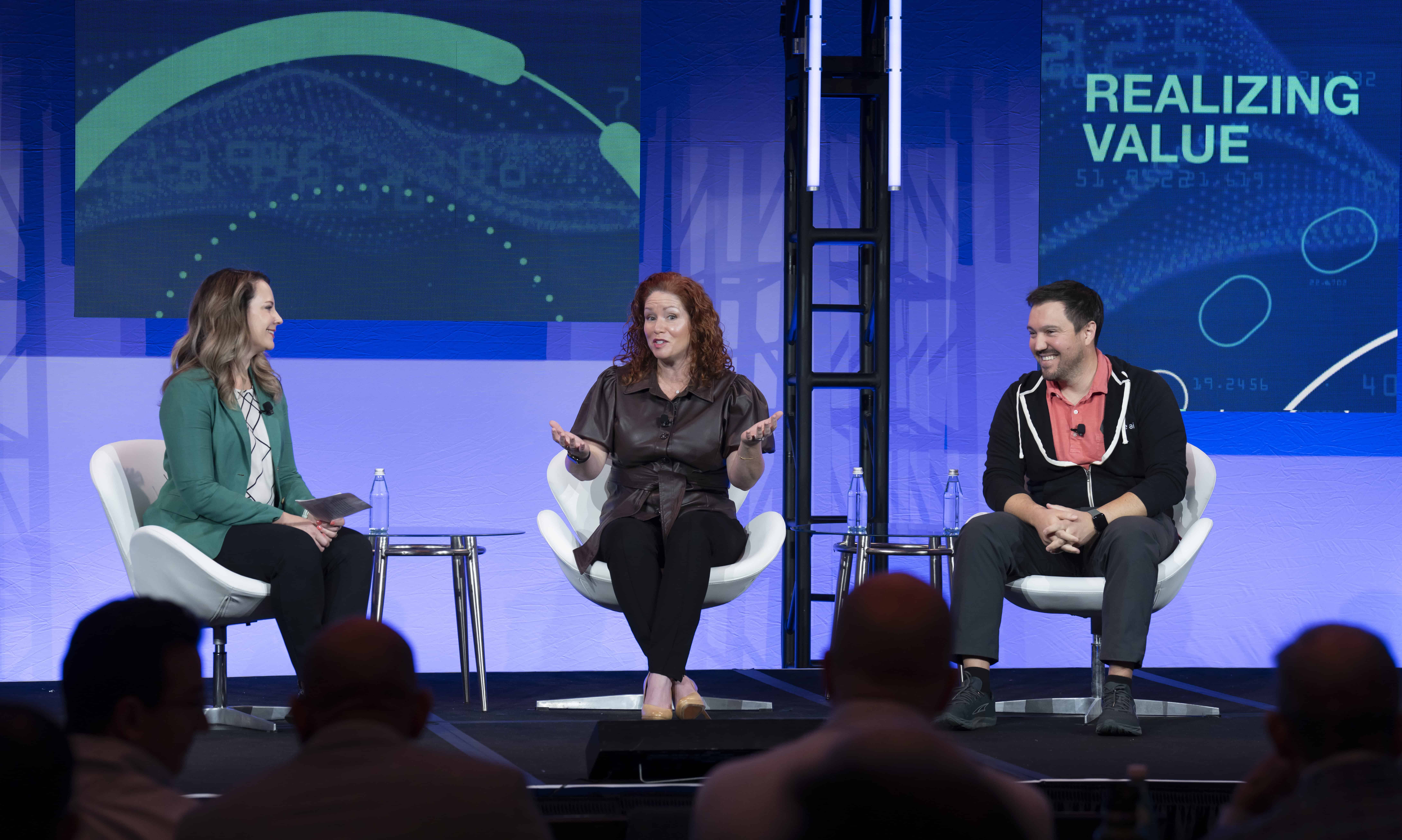
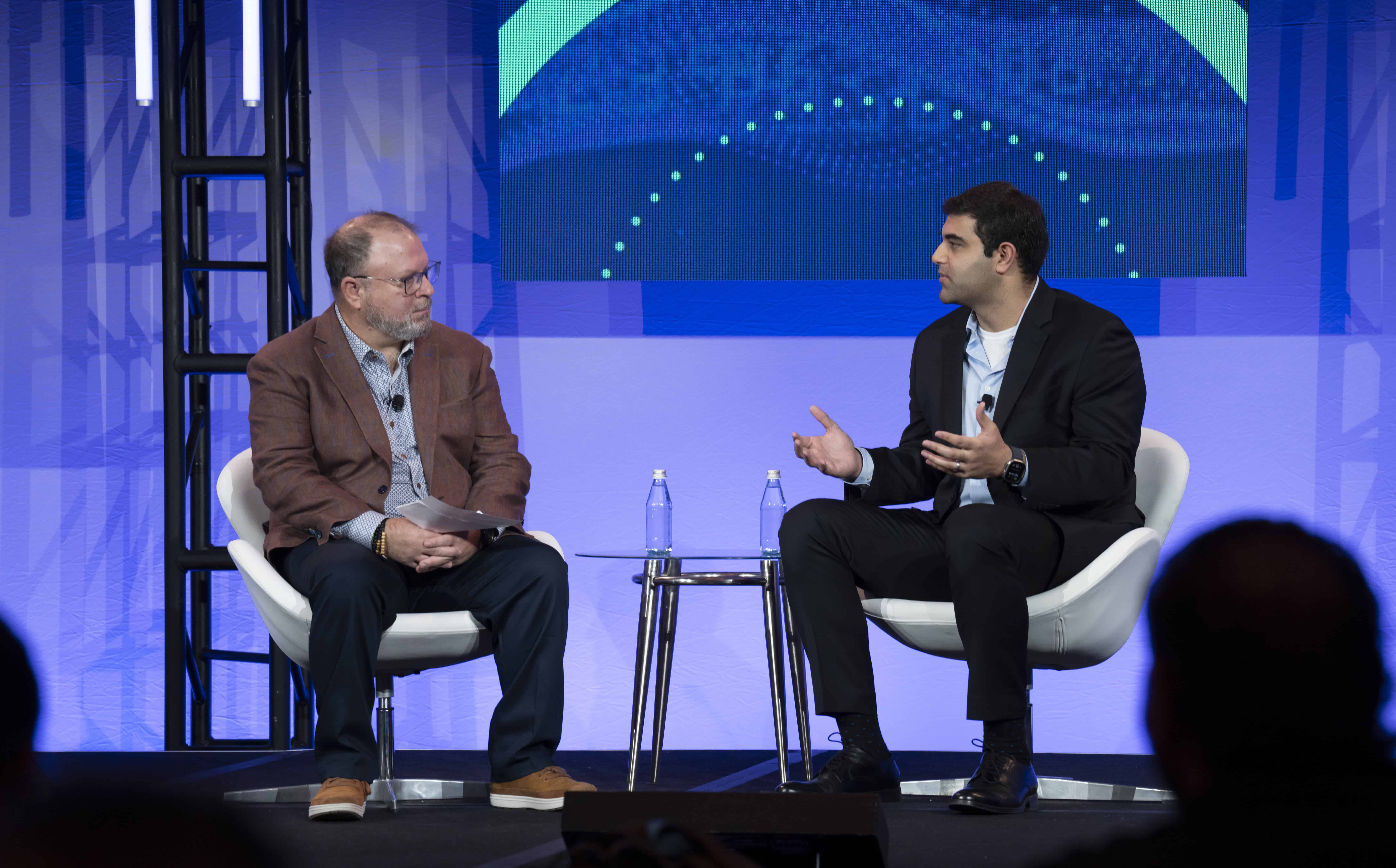
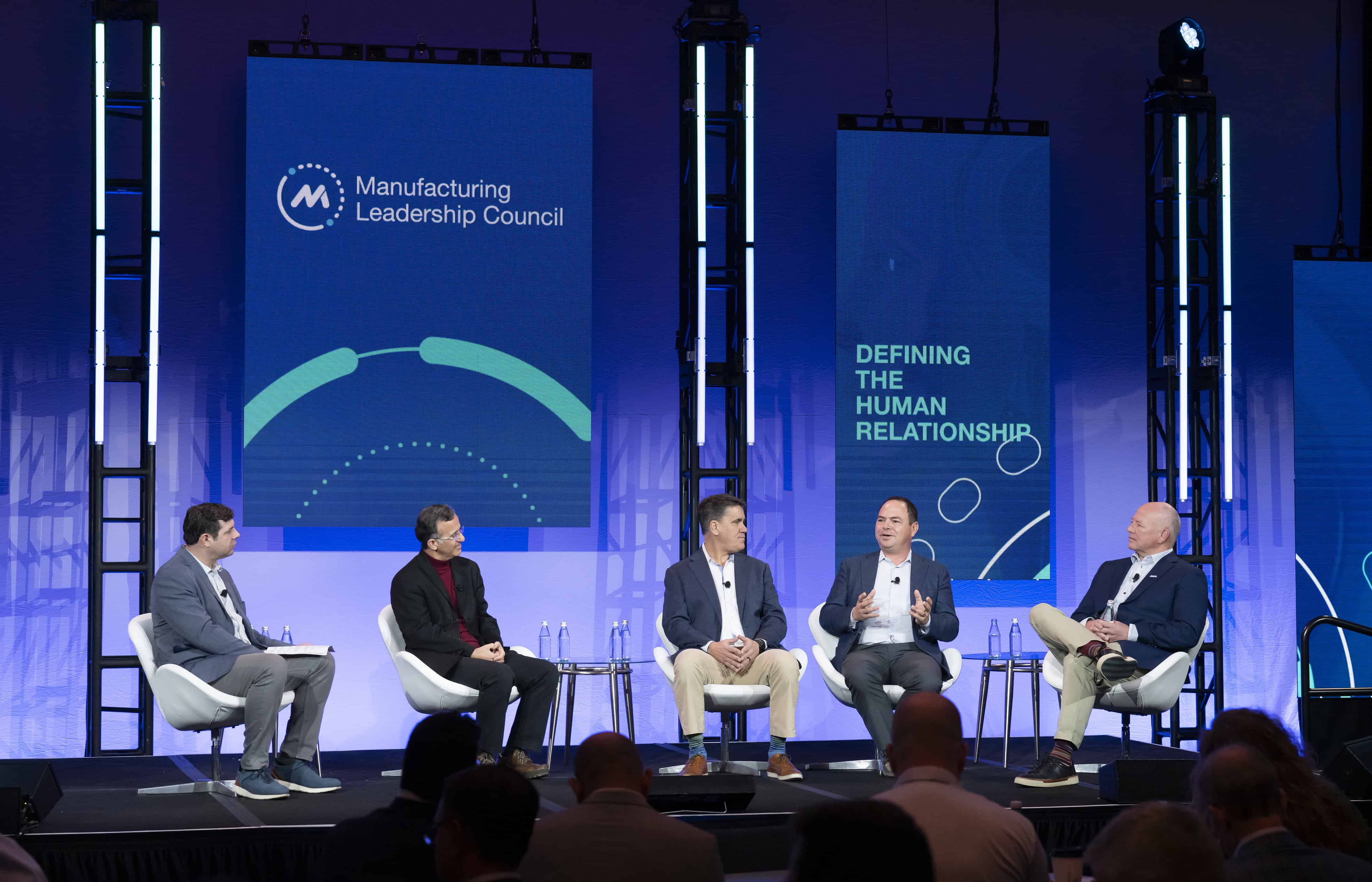
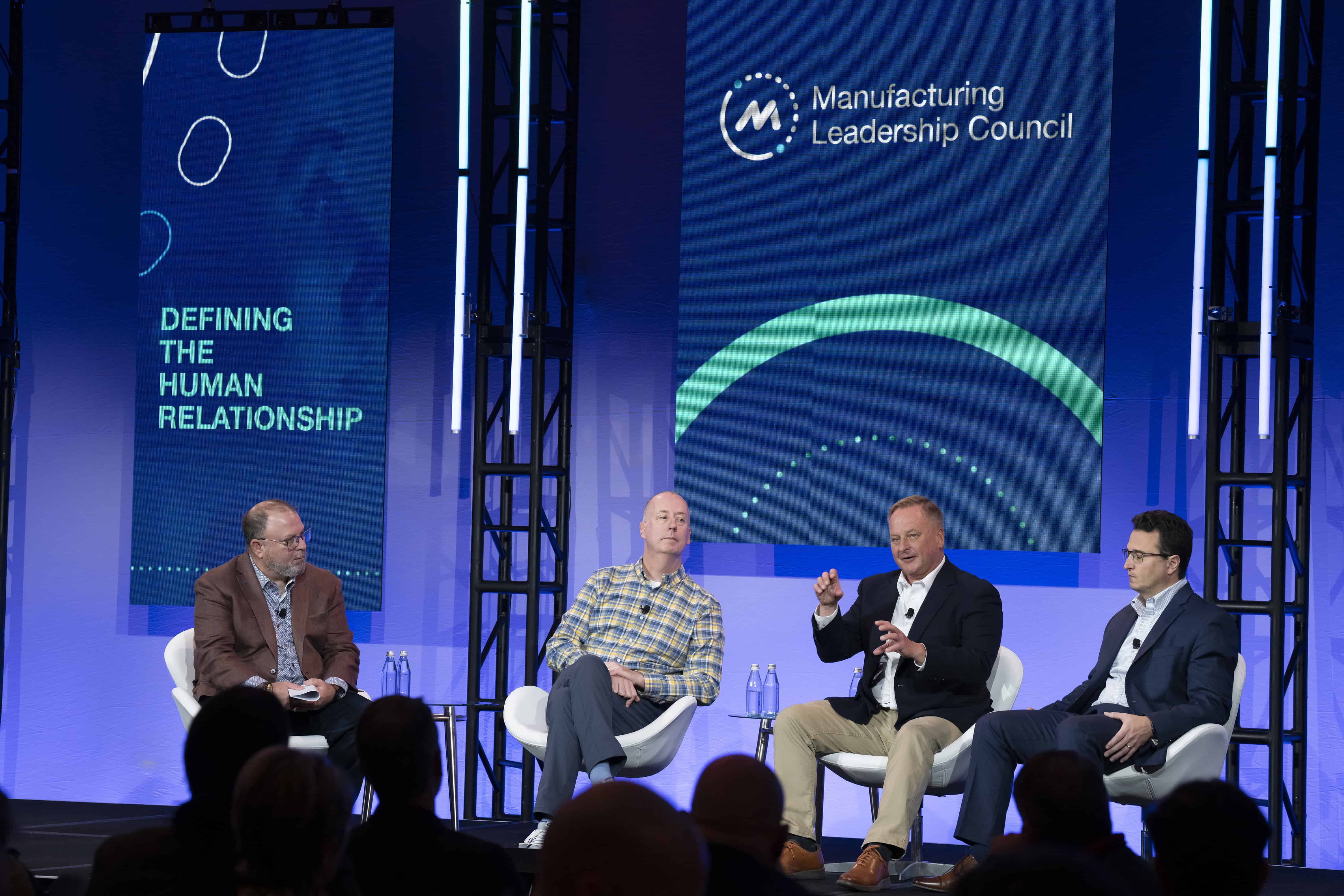
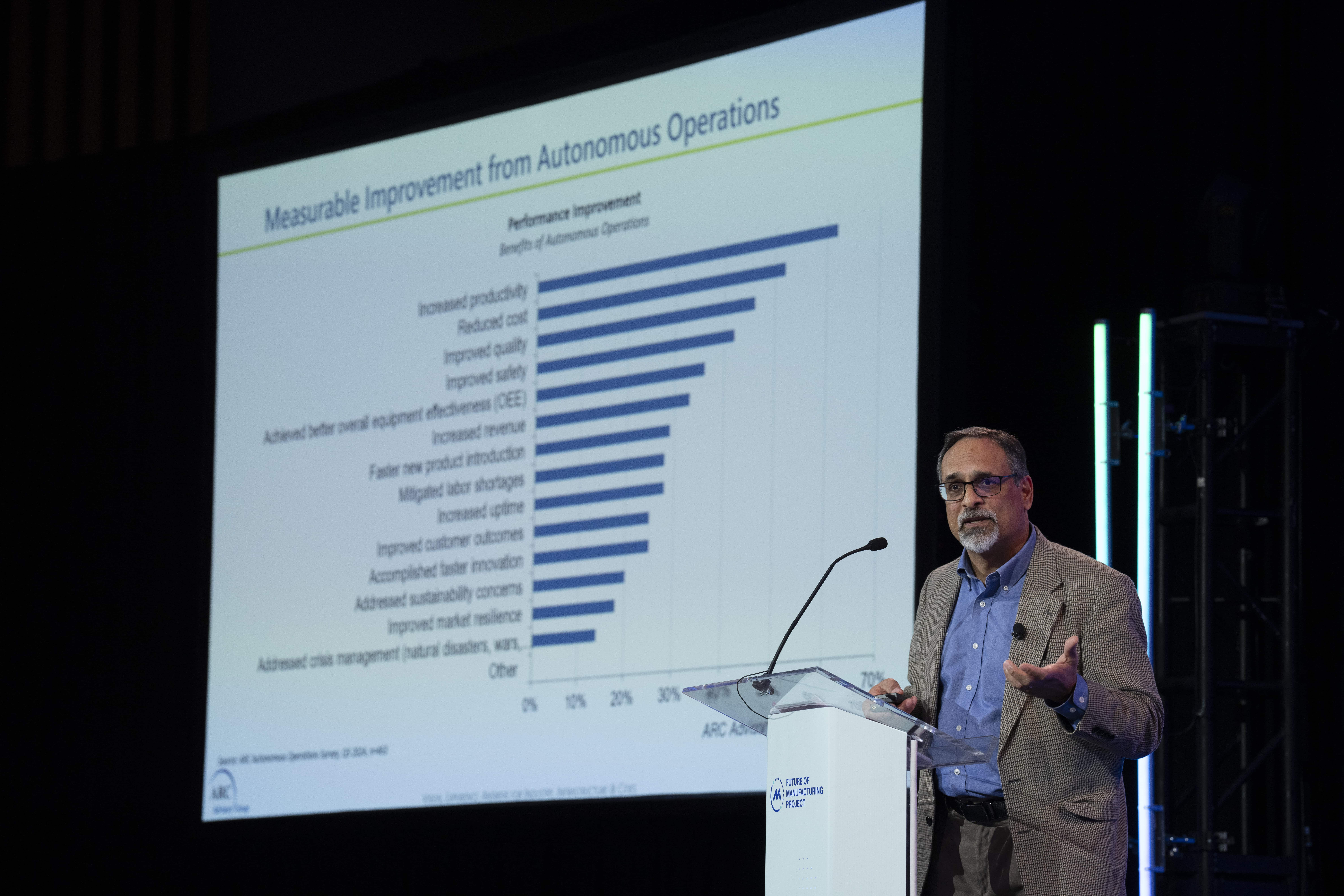
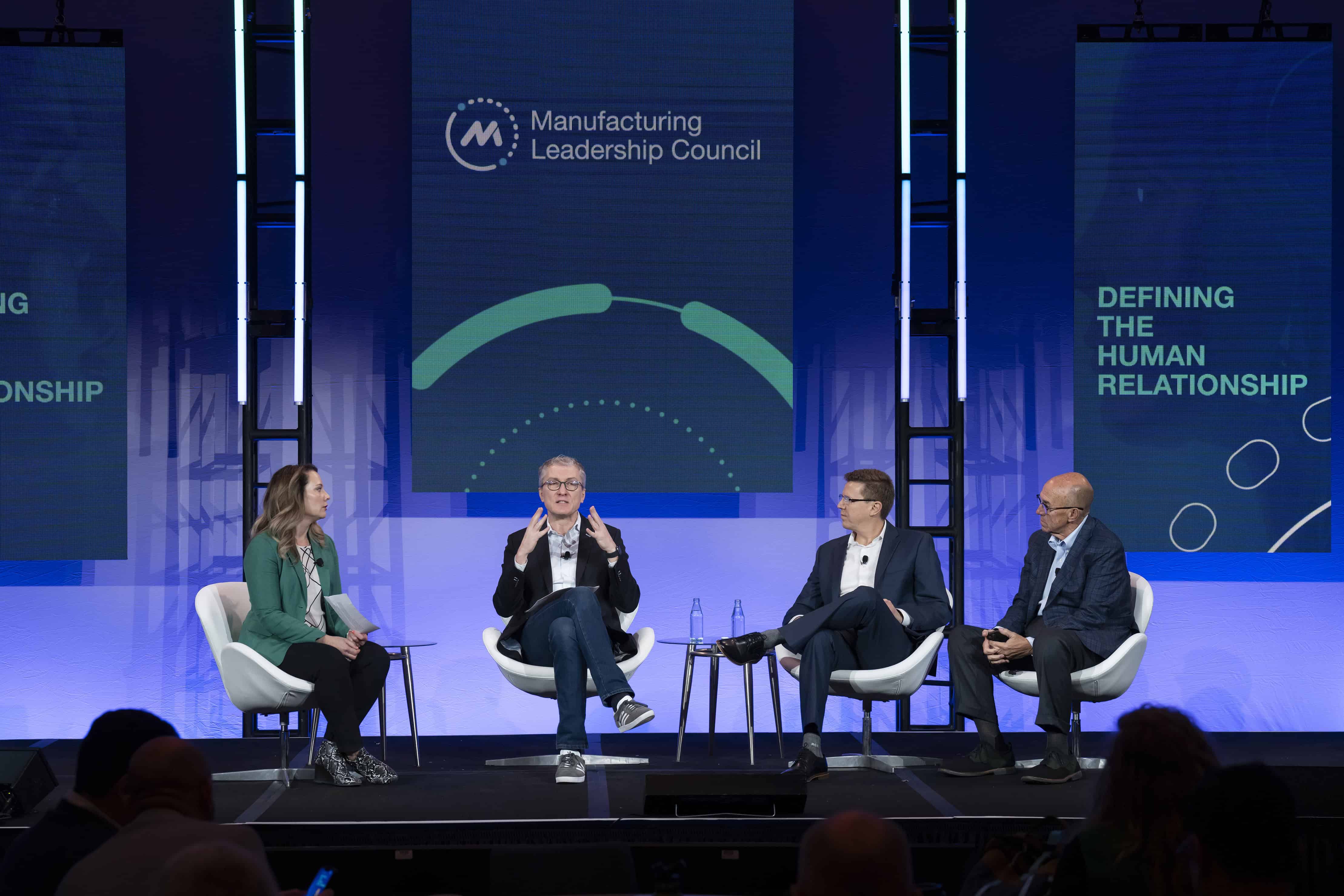
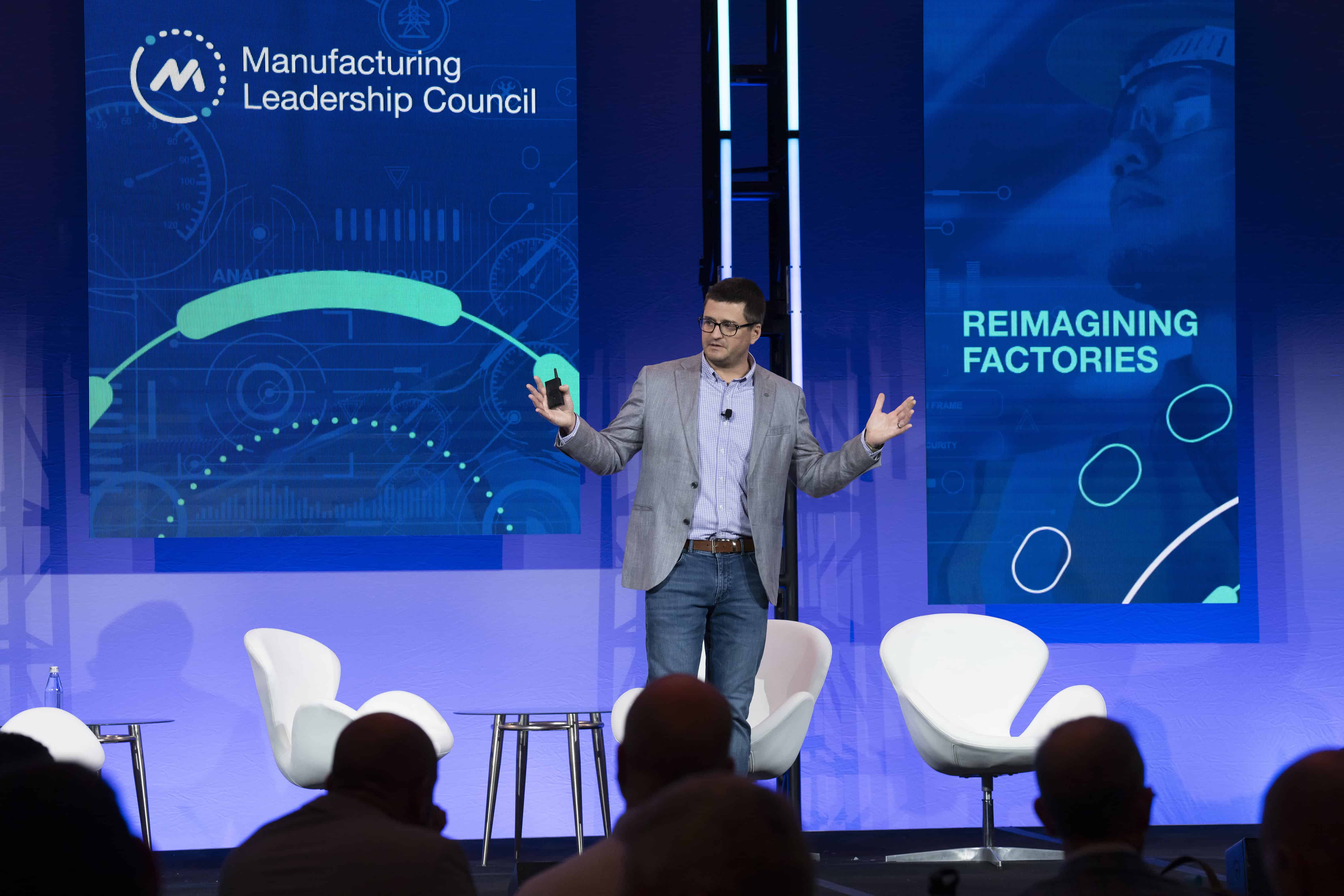
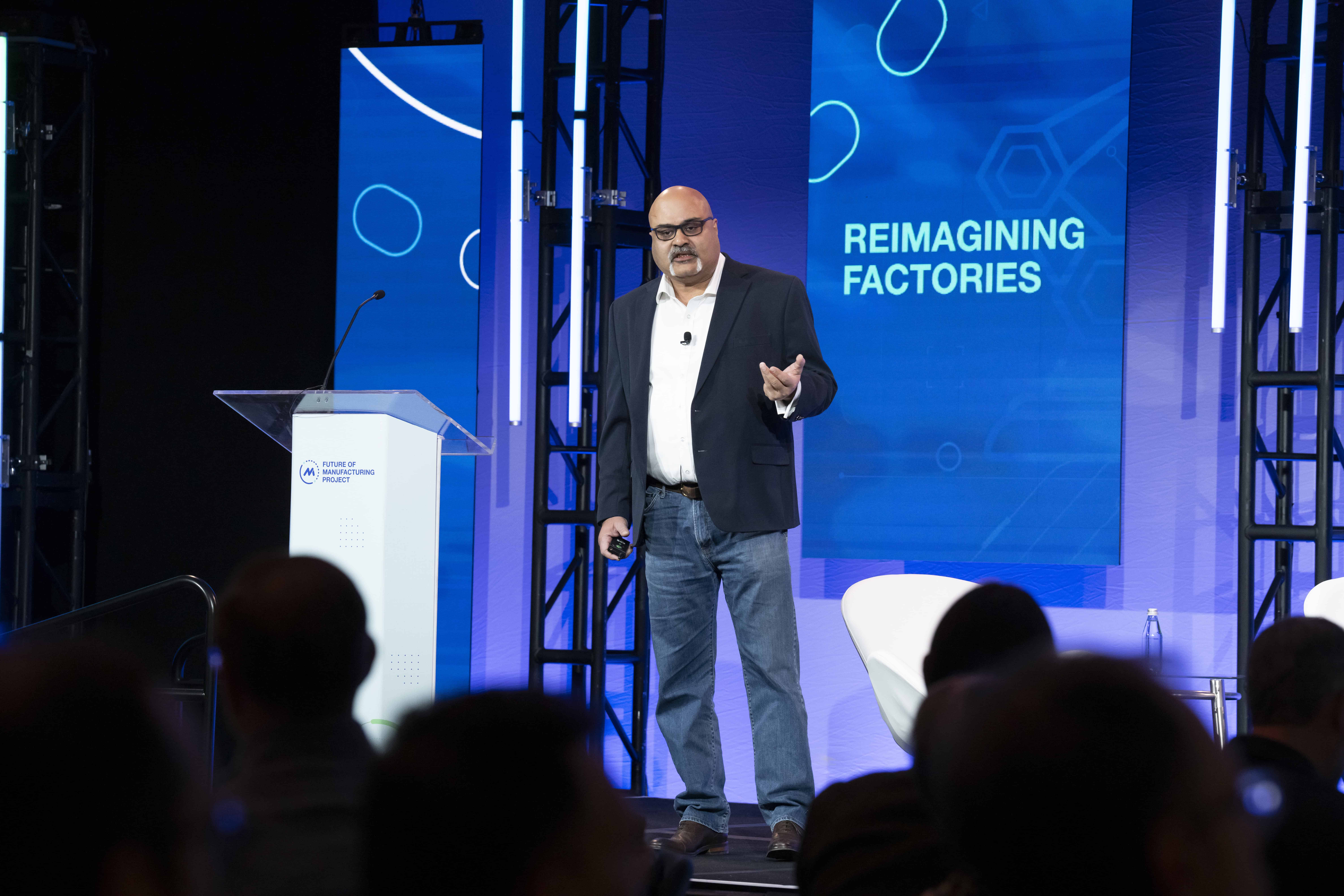
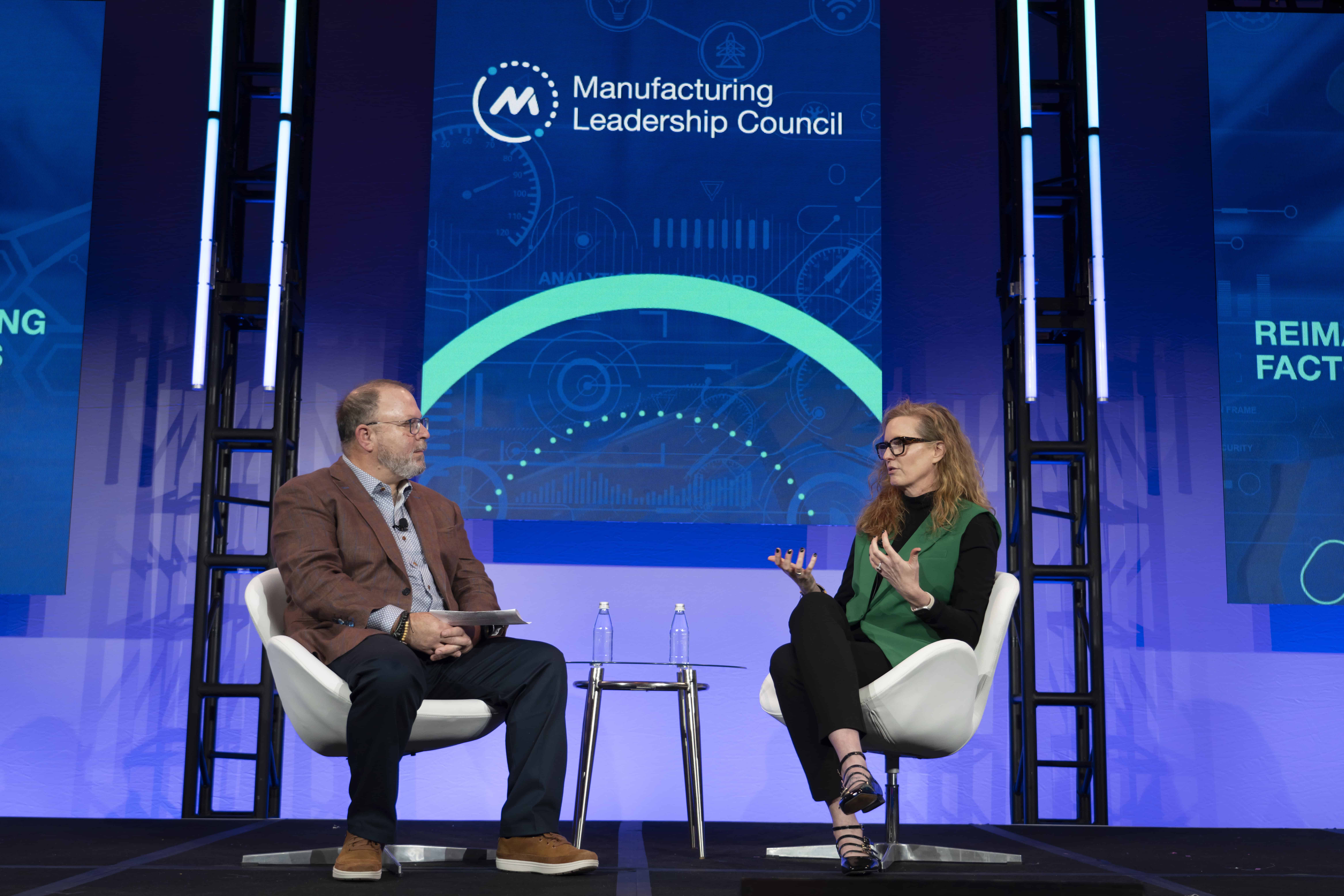
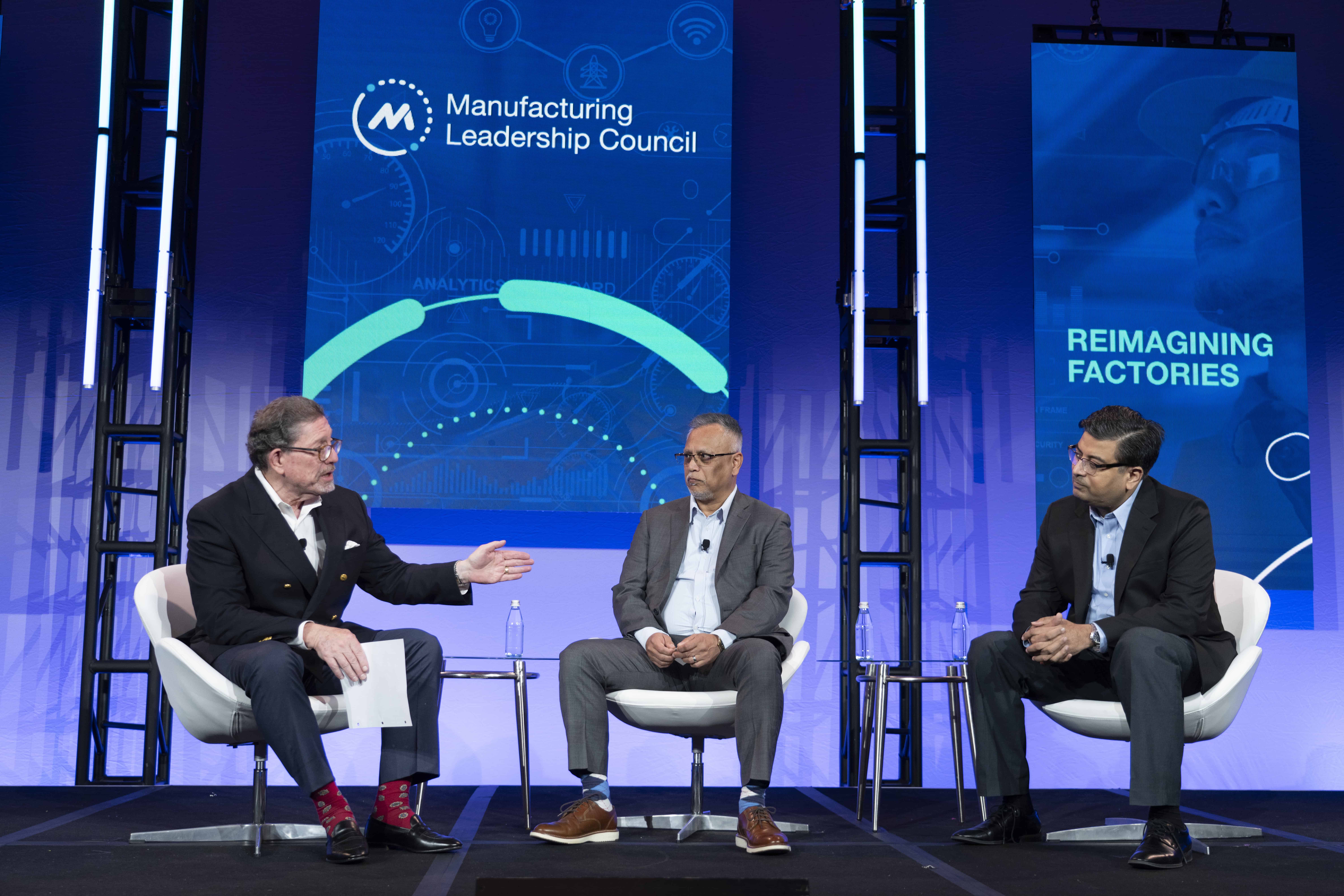
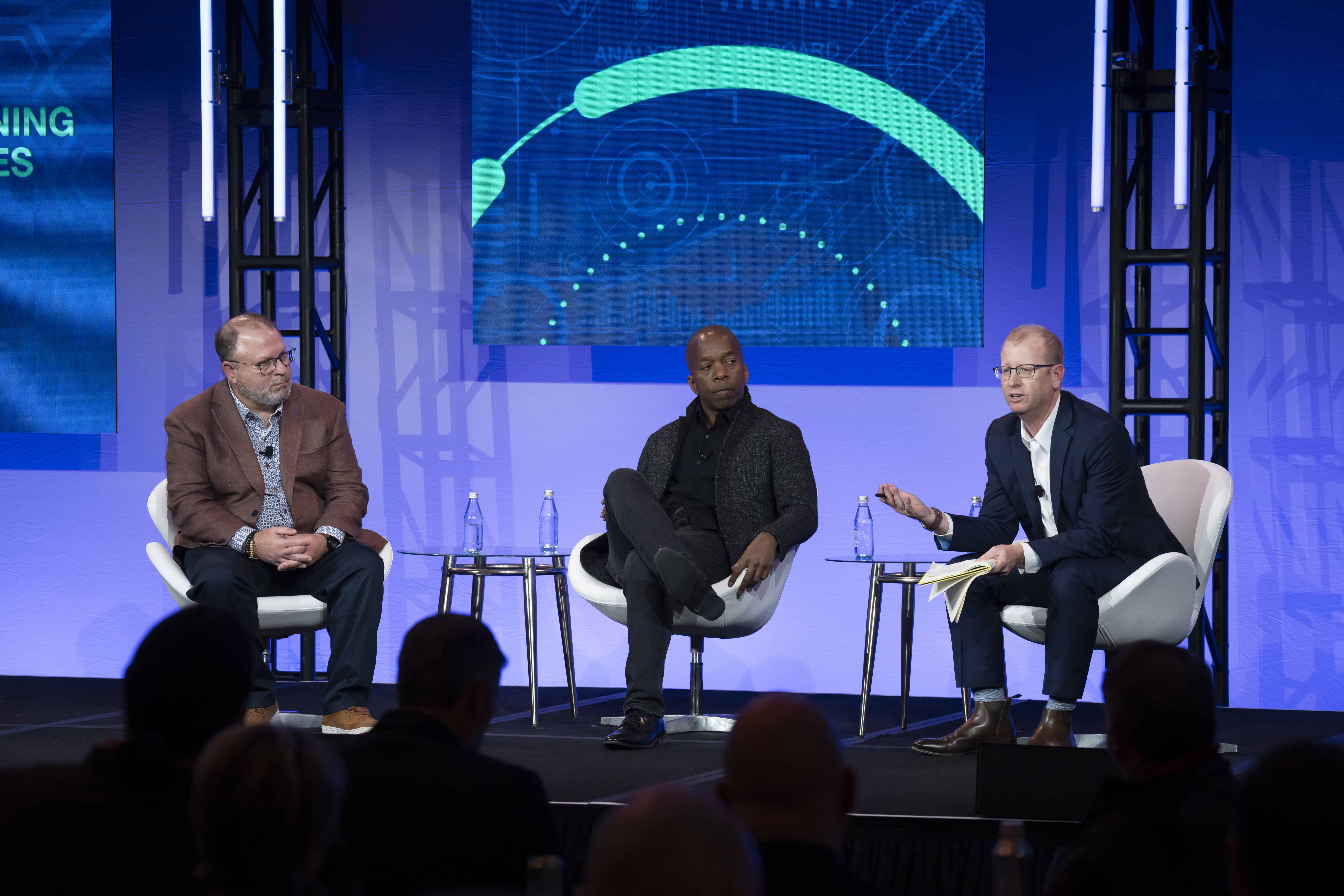
About the author:
David R. Brousell is the Founder, Vice President and Executive Director, Manufacturing Leadership Council
All Aboard the AI Train as Manufacturing Leaders Embrace the Future
The Manufacturing Leadership Council’s 2024 Future of Manufacturing Project event drew forward-thinking manufacturing leaders to Orlando, Fla., for a deep dive into AI-centered factories of the future. The Future of Manufacturing Project is an on-going initiative focused on key trends manufacturers must understand today to help them realize a better future.
David R. Brousell, MLC’s Founder, Vice President and Executive Director, opened the event with a sweeping overview of AI’s transformative role in the manufacturing sector and beyond. He began by highlighting the explosive growth of generative AI tools like ChatGPT, which became a global phenomenon almost overnight. Brousell explained how this rapid adoption of AI has served as a critical inflection point, fueling innovation, competition and investment at unprecedented levels across industries and nations. He underscored the staggering economic potential of AI, citing projections that it could contribute nearly $20 trillion to the global economy by 2030.
“A worldwide competition for dominance in AI is underway — and it appears to be intensifying,” Brousell said.
Major investments and initiatives by the U.S., China and Saudi Arabia highlight the AI race. He illustrated how these efforts reflect the high stakes of AI’s development, with nations and corporations alike vying for leadership. However, he cautioned against overhyped expectations, acknowledging the historical challenges of AI adoption and the – unlikely as it may seem – potential for a third AI winter. To navigate these uncertainties, he emphasized the importance of understanding both the opportunities and the risks that AI presents.
Drawing from MLC research and recent industry studies, the opening address included key findings on how manufacturers perceive and are implementing AI. While most manufacturers recognize AI as a game-changing technology, only a small percentage rate their current AI maturity as advanced. Despite this, many companies are ramping up their investments and seeing early signs of positive returns, particularly in areas like operational efficiency and productivity. Brousell also identified significant challenges, such as data quality, skill gaps and the need for metrics to measure AI’s impact.
To guide manufacturers in their AI journeys, Brousell offered practical recommendations: think big and envision bold possibilities, stay informed on technological advancements, collaborate with industry communities, establish ROI metrics and prioritize a human-centered approach to AI adoption.
He closed by reflecting on the manufacturing sector’s historic role as a driver of societal progress, calling on leaders to define their partnership with AI responsibly. His message was clear: the AI train is leaving the station, and manufacturers must be ready to board.
Access full text and a video of David R. Brousell’s opening address.
Future of Manufacturing Project: The Growing Competition for AI Supremacy
The Future of Manufacturing Project has become the premier platform for exploring the transformative forces shaping the manufacturing industry. At the 2024 event, David R. Brousell, MLC’s Founder, Vice President and Executive Director, delivered a powerful opening address that set the tone for the discussions and presentations to come. In his speech, Brousell examined the meteoric rise of AI technologies like ChatGPT, the growing global competition for AI leadership, and the profound implications for manufacturers. He offered data-driven insights, candid reflections on challenges, and practical guidance for navigating the AI-driven future. Below is a recording and the full text of his address — an essential read for anyone seeking to understand the opportunities and responsibilities that lie ahead for manufacturing and beyond.
Good morning, everyone, and welcome to the fourth annual Future of Manufacturing Project event.
Two years ago in Nashville, when MLC first devoted this event to examine the potential as well as challenges with artificial intelligence in manufacturing operations, OpenAI had just released the generative AI tool ChatGPT.
I had mentioned OpenAI in my talk that day, but little did we know what would soon be coming. ChatGPT took off like a rocket ship, capturing the imagination of the public, businesses, and governments, raising both aspirations as well as fears about the technology.
ChatGPT reached 100 million users in just two months after its launch, becoming the fastest growing consumer application in history at that time. Today, ChatGPT has a reported 200 million users.
ChatGPT became an inflection point for AI in general. The energy and momentum around AI in its many forms – IBM alone has identified seven types of AI — have not only continued but appear to be picking up even more steam. In the generative AI market alone, a flood of competitors has joined ChatGPT including Midjourney, Stable Diffusion, Bard, and, of course, Copilot, and others.
The amount of literature being produced about AI – and I use the term “literature” with an asterisk attached – is almost impossible to keep up with. Every day, it seems, there are multiple newspaper and magazine articles, research reports, case studies, government reports, product and service announcements, and conferences extolling both the virtues and warning of the challenges with the technology.
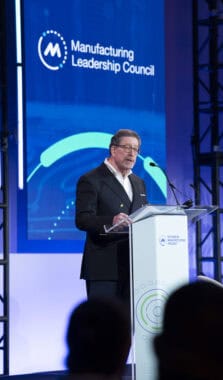
Huge sums of money are flowing into the AI market as nations earmark funds, announce national strategies, and set up organizations to capitalize on the technology; as technology and service providers produce new AI and augmented products and offer implementation methods; and as businesses assess the effect of AI on their competitive posture and develop their own goals and objectives with the technology.
As I said in Nashville two years ago, a worldwide competition for dominance in AI is underway – and it appears to be intensifying.
Here are a few datapoints to consider:
- In a report published in September, International Data Corp. predicted that AI would have a cumulative global economic impact of $19.9 trillion through 2030, representing 3.5% of global GDP by that year. Every new dollar spent on business-related AI solutions and services will generate, IDC estimated, $4.60 into the global economy, in terms of indirect and induced effects.
- Meanwhile, spending on AI is growing at double-digit rates. IDC estimated that worldwide spending on AI, including AI-enabled applications, infrastructure, and related IT and business services, will grow at a 29% compound annual growth rate between this year and 2028, reaching $632 billion in 2028. In terms of use cases, Smart Factory floor investments are expected to grow at a 32.5% CAGR in that time period.
- The number of AI patents granted worldwide rose 62.7% between 2021 and 2023, according to the 2024 Stanford AI Index Report. In 2022, China led global AI patent origins with a 61.1% share, significantly outpacing the U.S., which accounted for 20.9% of AI patent origins. In that same year, however, the U.S. far exceeded China in terms of financial investments. In the U.S., AI investments reached $67.2 billion in 2022, 8.7 times more than China.
- Announcing AI strategies has become commonplace among nation states. The U.S. has its National AI Initiative Act, a Presidential Executive Order on AI, and is in the process of standing up an AI Institute under the America Makes program.
- In China, well, we know there is much activity but getting reliable data is a challenge. For example, International Data Corp. estimates that China’s investment in AI will reach $38 billion in 2027. Meanwhile, a report in Yahoo Finance in September said that China’s AI industry could invest $1.4 trillion into developing the technology in the next six years. Pass the salt!
- On the other side of the world, the European Union has its AI Innovation Strategy, which calls for the creation of AI Factories across the European Union. Back in Asia, India has its AI Mission initiative; Japan’s Ministry of Economy, Trade and Industry has allocated $740 million to subsidize the AI computing industry in Japan; and South Korea has a plan to spend $6.9 billion by 2027 on artificial neural processing units and high bandwidth memory chips.
But one of the more notable announcements this year came from Saudi Arabia, which created a $100 billion fund to invest in AI and other technologies.
***
Now, cautious observers, particularly those with a historical context, might say that all the posturing and the financial projections and investment numbers merely reflect an inflated state of expectations, a euphoria born of excitement about the shiny new car. We are in what Gartner calls a hype cycle that will, in time, deflate as the challenges of implementing and optimizing use of the technology become better understood and experienced.
No doubt there is some truth in this. As we know from the experiences we’ve had with other technologies, strategic, organizational, cultural, and human behavioral challenges govern whether a technology project will ultimately be successful. I myself have talked for many years about what I call The Absorption Gap – the time and effort required to go from implementation to benefit. It often takes much longer to get to benefit than we think at the outset.
Could history repeat itself with AI? Could we yet experience a third AI winter, after the two that occurred last century when expectations were disappointed?
Anything is possible, of course, but I wouldn’t bet on it. The foundational conditions that were lacking years ago to enable AI to take off – compute power, storage, communications bandwidth, sufficiently large data sets – have all been addressed. Moore’s Law will ensure that price/performance will improve, perhaps significantly, over time. And the unrelenting need to find new competitive advantage will compel companies to go forward into the known and, perhaps more importantly, the unknown.
My friends, the AI train is leaving the station – and you must be on it.
***
The encouraging news is that aspirations with AI among manufacturers are strong and there is already some evidence that AI is, as they say, worth the squeeze.
Recent MLC research shines a light on what manufacturers are thinking about the use of AI in their operations.
Among the many findings of our new AI study, results of which were published in the Manufacturing Leadership Journal in August, perhaps the most important is that a majority of manufacturers, 55%, see AI as a “game-changer” in the industry by 2030, even though only 5% at this point in time assess their maturity level with AI in operations as “advanced”.
Perhaps propelled by this perception, 78% said they plan to increase spending overall on AI tools over the next 12 to 24 months, with
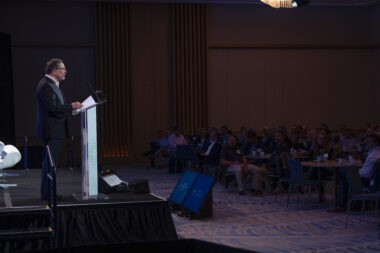
one-fifth expecting to increase their spending by more than 30%. Already, more than half are using GenAI tools such as ChatGPT or Microsoft’s Copilot, and more than 80% said they expect to increase the use of such tools in the next two years.
As manufacturers ramp up their AI investments, early indications about the impact and return on these investments are looking positive.
In July, EY, a member of the Manufacturing Leadership Council and a partner in the Future of Manufacturing Project, published the results of its first AI Pulse survey, which asked 500 senior leaders across industries in the U.S. about AI investments, impacts, and challenges.
The study found that senior leaders whose organizations are investing in AI have seen tangible results across business functions, including about three-quarters who are already experiencing positive ROI in operational efficiencies, employee productivity, and customer satisfaction.
Moreover, the EY study found that organizations that are committing 5% or more of their budgets on AI are seeing “outsized returns.”
And all of this is happening as AI continues to advance. This year’s Stanford report assessed that AI has now surpassed human performance on several benchmarks, including in image classification, visual reasoning, and English understanding. However, the technology still lags human capabilities in competition-level mathematics, visual common-sense reasoning, and planning, Stanford said.
I suppose that we can all take some comfort in those findings. We humans will continue to dominate – at least for the moment. We don’t know how fast AI will evolve and how capable and powerful it will become in the years ahead, but we know one thing for sure – with the amount of capital flowing into the AI market and the amount of effort being applied to its development, AI will not sit still.
As it continues to move, manufacturers have a host of issues to deal with to ensure that AI is used effectively to advance their organizations’ business and operational goals, and in a responsible manner.
Once again, MLC research spotlights some of the challenges.
The top concern, say 68%, is around data – quality, contextualization, and validation. More than 40% also identify AI skills and AI business case development as issues. Sixty-six percent say they do not have a specific set of metrics to measure the effectiveness and impact of AI implementations at this time.
This all goes back to that single digit finding I mentioned earlier about maturity levels with AI. The question is: what will it take to accelerate adoption and use of AI in operations and enable companies to climb up the maturity curve?
The easy answer is time, money, and persistence. But there’s a bit more to it than these three foundational elements. Please allow me to offer a few recommendations:
First, I can’t emphasize strongly enough about having a vision for what you want to do with AI. That may sound obvious, and it is on one level, but it takes a lot of thinking, strategizing and even soul-searching to craft an idea of what your company could be in 10 or 15 or 20 years.
So, think big about AI and what your company might be able to do with it. Think about the art of the possible. Think about growth and not just in what you are doing now but what you could be doing that’s new, innovative, and exciting.
At first, you will turn your attention to using AI to become more efficient, more cost-effective, faster. These are all important objectives, but don’t stop there. Imagine a better future.
And once you craft a business vision for the future – a vision that should be flexible enough to change when needed – make sure your AI strategy “functions as the fuel,” as Council member Deloitte says, for the business strategy.
Second, keep up with the evolution of the technology. There is a lot of energy and momentum around AI, as I have detailed, and new developments are occurring frequently. The technology must be monitored and understood, as well as the rules, laws, and regulations that will govern its use. Designate someone or a specific business unit in your company to keep track of developments to understand their potential to help your business.
Third, don’t try to go it alone. Plug into the community. MLC is here to bring together your fellow manufacturers, technology and services providers, academics and research organizations, and sister groups such as the IRI, CESMII, and MxD so you have access to a robust community for help.
Fourth, work on the metrics. Experimentation with AI is necessary and important, but the laws of business must be served. Find, adopt, and use ROI models to prove return so that investment continues.
Fifth, it is essential to take a human-centered approach to AI. Bring people along by showing them the benefits and being candid with them about the challenges. Change management and training are key.
Taken together, these simple, common-sense tenets can help you navigate your journey with AI.
***
What we do here today, and what you do after this conference when you go back to your companies, will help propel the manufacturing industry forward.
But our responsibility is greater than just our own industry. AI has not only the potential to reshape manufacturing but society itself. As it has many times throughout history, this industry is playing a vital role in deciding what the future might look like. Once again, the mantle of change lies at our feet.
I would like to close with a quote from a book called “The Age of AI And Our Human Future,” authored by Henry Kissinger, Eric Schmidt and Daniel Huttenlocher:
“The advent of AI, with its capacity to learn and process information in ways that human reason alone cannot, may yield progress on questions that have proven beyond our capacity to answer. But success will produce new questions … Human intelligence and artificial intelligence are meeting, being applied to pursuits on national, continental, and even global scales.
“Understanding this transition, and developing a guiding ethic for it, will require commitment and insight from many elements of society: scientists and strategists, statesmen and philosophers, clerics and CEOs. This commitment must be made within nations and among them. Now is the time to define both our partnership with AI and the reality that will result.”
Thank you and have a great conference.
About the author:
David R. Brousell is the Founder, Vice President and Executive Director, Manufacturing Leadership Council
Photos by David Bohrer / National Assoc. of Manufacturers