AI-Driven Factories of the Future: It’s a Lot More than Just Autonomy
The future of manufacturing is about intelligently blending autonomous operations, augmented intelligence, and flexibility to redefine production.
TAKEAWAYS:
● Manufacturers can achieve greater consistency and scalability in operations by leveraging AI, robotics, and digital twins.
● Fully autonomous factories are often more obtainable for large-scale manufacturers with high-volume, low-variation production.
● Small and medium-size companies will likely derive more benefits from targeted lights-out processes, known as dim factory operations.
Imagine a factory that operates seamlessly around the clock, with artificial intelligence (AI)-powered robots building products at an efficiency and precision unmatched by human hands. Supervisors step in only for exceptions, while AI ensures optimal performance and quality. The concept of a lights out (or dark) factory is often attributed to a 1955 science fiction story, “Autofac,” by author Phillip Dick.
As manufacturers worldwide invest aggressively in artificial intelligence (AI), the idea of fully autonomous dark factories is becoming increasingly viable. These facilities promise significant improvements in throughput, quality, safety and efficiency. Yet, while the enabling technology exists, only 15% of manufacturers have transitioned to fully autonomous operations. The future of manufacturing isn’t just about going dark; it’s about intelligently blending autonomous operations, augmented intelligence, and flexibility to redefine production. Lights out or not, the ideal AI-driven factory of the future will be Scalable, Automated, Serviceable, Intuitive (Accessible), Flexible, and Interoperable.
The Quest for Competitive Advantage
Dark factories — operating 24/7 with minimal human intervention — are often heralded as the pinnacle of manufacturing efficiency. By leveraging advanced AI, robotics and digital twins, among other advanced technologies, these facilities can achieve unparalleled consistency and scalability.
The global AI in manufacturing market is valued at over $5 billion in 2024 and is growing at a 38.5% CAGR. According to the International Federation of Robots, there are now over four million operational industrial robots in operation, nearly double the number since 2016, with installations surpassing 500,000 units annually. Ninety-six percent of manufacturers intend to continue to increase AI investments over the next five years, and nearly half (49%) have fully integrated AI-driven product and/or service changes into their capital allocation process.
However, fully autonomous factories are not a one-size-fits-all solution. They require significant capital investment, robust digital infrastructure and standardized processes — factors that are often more attainable for large-scale manufacturers with high-volume, low-variation production. The stakes have never been higher for garnering return on innovation investments.
The Promise of Going Dark
Dark factories are best considered as weapons of mass production. They are most suited for larger manufacturers that have a) steady, high volume production runs, b) largely standardized production processes, c) precise specifications and quality controls, d) highly digitized assets and robust digital twins/threads, and e) a workforce that is prepared to embrace change. These larger manufacturers can also benefit from economies of scale that will improve the return on investment. Several examples are:
- FANUC, a pioneer in lights-out manufacturing, has been operating an autonomous factory in Japan since 2001. Robots are building other robots (quite a dystopian-sounding concept!) at a rate of about 50 per 24-hour shift and can run unsupervised for as long as 30 days at a time.
- Linglong Tire’s new $990 million European factory started mass production in September 2024. It integrates cutting-edge technologies such as artificial intelligence, mobile sensing, industrial big data and industrial robots to achieve full automation — from the warehousing of raw materials to the delivery of finished products, greatly improving product quality and production efficiency.
- Philips’ factory in the Netherlands uses 128 robots to produce electric razors with minimal human intervention on certain shifts. There are only nine human quality assurance workers who oversee the end of the manufacturing process.
A More Practical Path: Dim Factories
For many manufacturers, especially small to mid-sized enterprises, the dim factory model offers a more viable alternative. By automating specific processes or shifts, these manufacturers can enhance efficiency, reduce costs, and address labor shortages without the need for full autonomy. This approach balances technological ambition with operational practicality, allowing for incremental improvements tailored to unique business needs.
Small and mid-size manufacturers and contract manufacturers typically deal with lower production volumes, higher product variety and more variable production demands. For these manufacturers, dark factory operations may be less practical or desirable. These types of manufacturers, as well as many large enterprises, would likely derive more benefits from targeted lights-out processes — or dim factory operations — vs. fully autonomous production operations.
Consider that manufacturers of all sizes that have modernized, AI-led processes achieve 2.5x higher revenue growth and 2.4x greater productivity. Process modeling and automation have also unlocked efficiency gains across end-to-end supply chains — reducing energy consumption by 22%, reducing inventory by 27% and reducing waste by 55%. Gartner research estimates that 60% of manufacturers will have more than two lights-out processes in at least one of their facilities by the end of 2025. The cost/benefit of a fully automated dark factory must be weighed against the value of these targeted, AI-driven process improvements.
As manufacturers invest in AI, the idea of fully autonomous factories is becoming increasingly viable.
Industry segments will also influence the potential benefits of AI-driven factory operations. For instance, customers in some industry segments (e.g., consumer products) are demanding a greater degree of customization or personalization. A recent survey showed that over 50% of consumers prefer personalized products and are willing to pay a slightly higher price for them. Manufacturers who deal with such demands may derive benefits from employing micro-factories, a concept that was first introduced in 1990 by the Mechanical Engineer Laboratory (MEL) of Japan. These smaller, agile, and highly automated micro-factories are capable of providing high-mix, low-volume customized products with a high return on investment.
Digital twins are becoming the backbone for AI-driven factories, providing a collaborative, connected information conduit across product design, production engineering, manufacturing execution, automation, and data-driven intelligence. Applications of digital twins have moved beyond simulation and modeling to automatically effecting manufacturing operation adaptations that maximize throughput and asset utilization, adjust to production variations, and mitigate impacts of unplanned disruptions.
Converting a traditional factory to a completely dark factory is a significant and capital-intensive undertaking. Brownfield factories have the burden of technical debt, siloed data architectures and repositories, a patchwork of IT and OT systems, and an abundance of non-digitized assets, among other challenges. These manufacturing facilities can still reap the incremental benefits of business-driven process automations as cited above. Conversely, new greenfield factories can incorporate digital and AI/automation from the onset without the additional cost and complexity of legacy systems, processes, and architectures. Indeed, the preponderance of the AI-driven manufacturing lighthouses are digital natives that benefit from those advantages.
The bottom-line is that while we are seeing automation and AI being deployed increasingly across the manufacturing sector, dark factories are far from a panacea. AI investments should always be aligned with business drivers and outcomes, not just technological feasibility or FOMO (fear-of-missing out). Business drivers for AI investments may may range from workforce and supply chain challenges, shifting consumer demands, economic and cost pressures, sustainability imperatives, necessary safety and quality improvements, and increasing global competition.
Human + AI: The Augmentation Advantage
Rather than viewing AI as a replacement for human workers, the most successful manufacturers see it as a partner. This shift toward “augmented intelligence” emphasizes collaboration, where AI enhances human decision-making and performance efficiency.
The manufacturing automation wave that was prolific at the turn of the century largely consisted of automating manual processes and workflows, resulting in a reduction of about 2.5 million jobs, according to Human + Machine: Reimagining Work in the Age of AI. We are now in an era of augmented intelligence, where automation and AI are working in concert with people to improve overall performance and output. Instead of replacing jobs, new jobs are being created in areas such as AI, data science, robotics, model-based systems engineering and related functions. Manufacturers investing in upskilling and reskilling their workforce are not just future-proofing operations but establishing a catalyst for innovation.
Major Challenges and Opportunities
Achieving the transformative promise of AI-driven manufacturing is not without its challenges. Manufacturers consistently identify five critical barriers: data quality and integration, cybersecurity threats, technical debt, workforce preparedness, and ecosystem complexity. These hurdles underscore the complexity of transitioning to AI-powered operations.
- Enterprise data management
AI thrives on high-quality, well-integrated data, but for many manufacturers, this condition remains elusive. AI applications are most often hampered by inadequate data quality (57%), poor data integration (54%), and weak data governance (47%). Only one in five manufacturers have production assets with data ready for use in existing AI models. The bigger the manufacturer, the greater the problem. Over half (52%) of manufacturers view a unified IT/OT data platform as essential for enabling industrial DataOps. Achieving this requires investments in modernizing data infrastructure, breaking down silos, and adopting scalable solutions. - Cybersecurity risks
Manufacturing is now the most targeted industry for cyber attacks, with operational technology (OT) bearing the brunt. Cyber-attacks have increased by 75% over the past five years. The manufacturing industry was the most targeted industry in 2023, and over 70% of cyber-attacks were OT related according to Zscaler Research. Ransomware costs are forecast to reach $265 billion by 2031, up from $20 billion in 2021. Recognizing these risks, 75% of manufacturers have made cybersecurity a top priority, and 70% of CEOs plan to increase investments in data, technology, and cyber defenses. Proactive strategies such as robust OT/IT integration and real-time monitoring are essential for safeguarding AI-driven operations.
“Dark factories are best considered as weapons of mass production.”
- Technical debt
Outdated systems and fragmented processes create technical debt that stifles innovation. Technical debt would require over $1.5 trillion to fix — and costs the U.S. $2.41 trillion a year in cybersecurity and operational failures, failed development projects, and maintenance of outdated systems. This technical debt also constrains interoperability of systems between engineering and design and the factory and between OT and IT. - Workforce preparedness
The human element remains both a challenge and an opportunity for scaling AI in factories. Hiring and training (upskilling and reskilling) remain among the top constraints to scaling AI in engineering, design, and factory operations. Seventy percent of executives are focused on reskilling/upskilling (vs. hiring) compared to 41% in 2020. In the future, every worker will become a knowledge worker and data literacy will be a foundational requirement. Knowing how to perform a process doesn’t mean a person understands the process or the potential enabling or constraining factors. Decision-making will be augmented by AI-powered insights, and employees at all levels will need to become more comfortable understanding and acting on data. To prepare for this future, manufacturers must embed continuous learning into their culture, equipping employees to collaborate effectively with AI systems. - Ecosystem partnerships
Although 69% of businesses are engaging actively in collaborative ecosystems, a large proportion (60%) believe that multisided partnerships are difficult to execute in practice. Poor strategic alignment with partners is the leading inhibitor of ecosystem strategies, along with other factors such as limited awareness of collaboration opportunities, a resistance to share data, and limited vendor understanding of business objectives. Speed and security are the top criteria when considering potential ecosystem partners, further echoing the need to efficiently scale solutions while addressing critical data governance and security requirements. Seventy-one percent of manufacturing CEOs who have high-performing partner ecosystems affirm that those ecosystems are providing greater growth opportunities than traditional M&A.
An AI Readiness Checklist
The journey to AI-driven manufacturing is complex, but with the right strategies, manufacturers can unlock unprecedented levels of efficiency, innovation, and resilience. Before embarking on the journey toward AI-driven manufacturing or dark factory operations, assess your organization’s readiness in these critical areas:
- Operational readiness: Are your processes standardized enough to benefit from automation? How diverse is your product portfolio? Have you effectively incorporated lean manufacturing principles? How scalable are your current operations? Where are your major bottlenecks?
- Technological readiness: Do you have digitized assets, robust data platforms, and integrated IT/OT systems? To what extent are you employing digital twins/threads (e.g., for products, factory, production, and performance)? How much technical debt is embedded in your current operations? To what extent are you using cloud/edge computing? What are your current AI/ML use cases and what are the impediments to scaling?
- Workforce and organizational readiness: Is your team equipped with the skills and cultural mindset needed to work alongside AI? Have you adopted a continuous learning mindset? How robust are your training, development and change enablement capabilities? Do you have leadership buy-in, commitment, and alignment with long-term strategy?
- Financial readiness: Have you accounted for initial capital investment, R&D, and recurring costs? What are your expectations for ROI and the payback period? Have you established KPIs to measure expected performance improvements? What is your risk tolerance? Are there government and/or tax incentives that can be leveraged to offset some costs?
- Security readiness: What is the extent of your network security and segmentation? How are you protecting your IT and OT systems against dynamic and increasing cyber threats? How robust is your data encryption? How are you addressing the vulnerability of the human factor? How are you addressing potential vulnerabilities across your supply chain? What training have you implemented for management and threat detection and deterrence?
- Data readiness: Do you have access to reliable, well-integrated data to support AI applications? Do you have someone who is clearly responsible and accountable for data management and governance? How mature are your enterprise data management processes? Have you accounted for the potential impacts (e.g., compliance, cost, risk) of evolving global policies and regulations regarding AI, data privacy and protection, and data residency?
- Enterprise readiness (e.g., Suppliers, Partners): How are you managing integration of third-party systems and data? How robust are your suppliers’ capabilities and what are the major risks? How effective are your ecosystem partnerships and relationships with software and technology providers? What is your strategy for in-sourcing/outsourcing aspects of your operations?
Closing Reflections
The factories of the future won’t just be automated; they’ll be adaptive, intelligent and collaborative. Future manufacturing operations will range from dark factories to dim factories to micro-factories, and employ digital twins and digital commercial manufacturing exchanges that will enable secure, agile, on-demand manufacturing production.
When determining how to best improve or transform your manufacturing operations, avoid a binary view that only considers function allocation between humans and machines (automation); also consider augmented intelligence and performance benefits from human + AI collaboration. Regardless of the level of automation and AI, it is imperative to keep humans in-the-loop as they will ultimately be held accountable for outcomes of actions and decisions.
For small and medium-size manufacturers, the dim factory model offers a viable alternative.
While AI has had periods of disillusionment (i.e., AI winters) due to over-hyped expectations and technical limitations, the conditions are quite different this time. Today, our world is significantly more digitized, sensorized, connected, and software-driven. Instead of a lack of real-time data, we now are challenged with deriving decision intelligence from a tsunami of data. The next generation of workers are digital natives who embrace, rather than fear, technology innovation. Accelerating technological advances in areas such as computing power and speed, cloud/edge computing, computer vision, generative AI, digital twins/threads will make AI-driven factories — including dark factories — more viable over the next five+ years.
The future is here; it’s just unevenly distributed. AI is already delivering value for many manufacturers, ranging from predictive maintenance to supply chain optimization to quality control. AI-driven robots, machine vision, and optimization algorithms are improving efficiency and flexibility. Addressing challenges related to cost, security, integration, data management, and workforce development will be crucial to unlock the full potential and widespread adoption of AI-driven factories.
Now is the time to take steps forward toward this vision — whether that’s injecting AI to automate specific manufacturing processes, improve human performance, or build a fully automated lights-out factory. Let’s shape the future together with confidence!
AI-driven factories of the future was the theme of the Manufacturing Leadership Council’s Future of Manufacturing Project event in December 2024. The EY organization is a member of the MLC. M
About the authors:
Brian M. Legan Principal, Ernst & Young LLP | Industrials and Energy Innovation Leader
Disclaimer: The views expressed in this article are solely those of the author and do not necessarily represent the views of Ernst & Young LLP, other members of the global EY organization or other organizations cited.
Unlocking Manufacturing Excellence with Digital Twins
Manufacturing leaders can leverage digital twin technology to drive operational transformation while ensuring sustainable, long-term value creation.
TAKEAWAYS:
● Manufacturing organizations must develop comprehensive data governance frameworks before implementing digital twins to ensure long-term success.
● Successful digital twin initiatives require cross-functional collaboration and clear stakeholder alignment across all organizational levels.
● Leaders should prioritize cultural transformation and workforce development alongside technical implementation to maximize digital twin value.
Manufacturing stands at a pivotal moment of transformation. As Industry 4.0 technologies mature, digital twins emerge as a cornerstone of operational excellence, bridging the gap between physical assets and digital intelligence. Yet, successful implementation demands more than technological capability — it requires a comprehensive strategy encompassing organizational readiness, data capabilities, and workforce development.
Building the Foundation
Digital twin success begins well before implementation. Manufacturing leaders must first assess their organization’s digital maturity and readiness for this transformative technology. This assessment should evaluate current capabilities, identify gaps, and create a roadmap aligned with broader business objectives.
A robust data strategy forms the cornerstone of this foundation. Without it, organizations risk several critical pitfalls:
- Data Quality Challenges: Incomplete or inconsistent data can lead to unreliable simulations and eroded stakeholder trust.
- Integration Complexities: Manufacturing environments often combine legacy systems with modern equipment, requiring careful integration strategies.
- Performance Issues: Without reliable, real-time data, insights may lack timeliness and relevance.
- Resource Inefficiencies: Poor data management often requires costly retroactive cleanup and standardization.
The Human Element
Technology adoption success relies heavily on cultural transformation. Leaders must recognize that implementing digital twins affects roles across the organization, from operations to maintenance and quality control. Clear communication about how these changes improve daily work and contribute to business success proves essential for adoption.
Cross-functional collaboration becomes particularly crucial. IT and operations teams must work closely to ensure accurate data capture and integration. Similarly, input from frontline workers who operate equipment daily can provide invaluable insights that enhance digital twin accuracy and utility.
Implementation Strategy
For mid-market manufacturers, successful implementation often starts with simplifying data integration. This might involve:
- Utilizing middleware solutions designed specifically for manufacturing environments
- Implementing basic but effective security measures
- Establishing regular calibration and update schedules
- Appointing digital twin champions to oversee integration and maintenance
The key lies in starting small while maintaining accuracy. Unlike other digital initiatives where “quick and dirty” prototypes might suffice, digital twins require precision from the outset to deliver value.
Stakeholder Alignment
Digital twin initiatives affect multiple organizational functions and stakeholders. Success requires clear value propositions for each group and effective communication channels. Beyond traditional meetings, organizations benefit from hosting workshops, particularly those focused on innovation, to align stakeholders around a shared vision. These sessions typically introduce participants to methodologies like design thinking while providing structured environments to identify solutions to pressing challenges.
Cross-functional workshops serve multiple purposes:
- Surfacing technical opportunities and cultural barriers
- Revealing differing priorities and potential resistance points
- Creating opportunities for greater departmental synergy
- Building shared ownership of the transformation process
Organizations should ensure these collaborative sessions translate into tangible outcomes through post-workshop initiatives such as pilot projects and regular follow-up meetings.
Future Considerations
The evolution of digital twin technology continues to accelerate. Integration with artificial intelligence promises enhanced predictive capabilities, while extended reality visualization could transform how teams interact with digital twins. Blockchain technology may soon ensure data integrity across complex supply chain networks.
To prepare for these advances, manufacturing leaders should focus on:
- Building cross-functional teams bridging operational and information technology
- Developing clear governance frameworks for data sharing
- Creating scalable architectures accommodating emerging technologies
- Establishing strategic technology partnerships
Digital twins represent more than a technological advancement — they offer a pathway to reimagining manufacturing excellence. As organizations embrace this journey, success depends on fostering a culture of continuous learning and adaptation. M
About the Author:
Jason Hehman is the Industry 4.0 Vertical Lead and Host of The Modern Industrialist podcast at TXI.
Harnessing Manufacturing 4.0: A Supply Chain Perspective
Manufacturing 4.0 technologies—including AI, machine learning, the Internet of Things, and cloud computing—can improve supply chain innovation and performance.
TAKEAWAYS:
● M4.0 technologies can process the vast volumes of data generated at every step of the production and distribution processes.
● Adopt a 4-part strategy: Implement cloud-based supply chain platforms; use the power of AI and predictive analytics; create a strong data integration framework; and manage change effectively.
● To accelerate the adoption of M4.0 technologies, start with a clear vision and roadmap, collaborate with technology partners, and focus on scalable solutions.
As manufacturers embrace Manufacturing 4.0 (M4.0) and the concept of “smart” manufacturing, many focus on the shop floor, implementing advanced technologies to improve integrated, efficient, and agile production processes. For smart factories to reach their full potential, supply chains must change to be equally intelligent and responsive. M4.0 technologies such as artificial intelligence (AI), machine learning (ML), the Internet of Things (IoT), blockchain, and cloud computing are changing supply chain management. These tools enhance operations by improving visibility, streamlining processes, and enabling real-time decision-making. Deploying supply chain solutions is complicated, however, due to challenges like system integration, legacy infrastructure, and the need for stakeholder alignment. This article identifies the most pressing supply chain challenges in the M4.0 era and offers recommendations for deploying M4.0 technologies to foster success.
Understanding the Complexity of Modern Supply Chains
Supply chains today are more complex than ever. They span global networks, involve different stakeholders, and are influenced by dynamic external factors such as geopolitical events, natural disasters, and shifting consumer demands. To make matters more difficult, data across the supply chain are often siloed within different departments and systems. With growing market pressures and increasingly complicated logistics, manufacturers are challenged by limited visibility, slower decision-making, and the need to balance cost-cutting with responsiveness to client demands.
This complexity is compounded by the integration challenges posed by legacy systems that have historically supported supply chain operations. For example, many manufacturers still rely on outdated enterprise resource planning (ERP) systems, transportation management systems (TMS), and warehouse management systems (WMS), which are often unable to communicate with new technologies or provide real-time insights.
“The challenge lies in managing these data effectively, integrating them from disparate systems, and using them to make informed, real-time decisions.”
In the era of M4.0, where data are generated at every step of the production and distribution processes, manufacturers must focus on how to seamlessly connect disparate systems and manage increasing volumes of data. This is where advanced technologies like AI, ML, the IoT, and cloud computing can be transformative for supply chain management.
Challenges in Supply Chain Management in the M4.0 Era
While many of these advanced technologies hold immense promise, manufacturers need to address four critical hurdles to ensure these technologies are adopted and deployed effectively in supply chains:
1. Complexity of interconnected systems
Supply chains involve multiple parties, including suppliers, distributors, retailers, and logistics providers. Each stakeholder may have its own systems, business processes, and levels of technological sophistication. This interdependence makes it difficult to achieve seamless integration. The lack of standardization in data formats and systems, along with the difficulty of aligning these varied systems and technologies, adds to the complexity.
2. Data complexity and integration
A key feature of M4.0 is the sheer volume of data being generated by sensors, devices, and machines. In supply chain operations, these data are spread across different nodes of the network—from suppliers’ warehouses to distribution centers to customers. The challenge lies in managing these data effectively, integrating them from disparate systems and using them to make informed, real-time decisions. Effective data analytics, predictive modeling, and ML algorithms are required to extract meaningful insights from these data, and they are dependent upon high-quality data.
3. Coordination across multiple stakeholders
The need for coordination between various external partners often compounds the complexity of modern supply chains. Information and logistics must flow smoothly across borders, companies, and systems. However, data privacy concerns, differing technological capabilities, and trust issues between stakeholders can hinder effective collaboration. Achieving true end-to-end visibility across the entire supply chain requires close alignment of business goals and technological frameworks.
4. Legacy systems and change management
A significant barrier to the adoption of M4.0 in supply chains is the prevalence of legacy systems. Many manufacturers still rely on older ERP, WMS, and TMS platforms that are not designed to work with modern cloud-based solutions or to handle the volume of data generated by connected devices. Transitioning to more advanced systems often requires significant investments in software upgrades, infrastructure, and training, which can slow down the adoption of M4.0 solutions.
Strategies for Deploying M4.0 Technologies
To address the challenges outlined above, manufacturers need to take a strategic approach when they deploy M4.0 technologies. Here are four practical recommendations for overcoming these challenges:
1. Implement cloud-based supply chain platforms
One of the most impactful ways to simplify the complexity of interconnected systems is by implementing cloud-based supply chain management platforms. These platforms can integrate data across multiple systems and provide real-time visibility into the entire supply chain. With cloud technology, manufacturers can centralize data from suppliers, warehouses, distributors, and retailers in a single system, making it easier to track inventory, shipments, and demand trends.
“One of the most impactful ways to simplify the complexity of interconnected systems is by implementing cloud-based supply chain management platforms.”
By using cloud-based platforms, manufacturers can be more flexible, work better with outside partners, and make sure that information flows smoothly across the whole network. Furthermore, these platforms often have built-in analytics tools that provide insights into performance metrics, enabling manufacturers to proactively manage supply chain risks and opportunities.
2. Use the power of AI and predictive analytics
The complexity of modern supply chains is further compounded by the need for real-time decision-making and the ability to predict future trends. AI and ML are transforming how manufacturers can analyze and use data. Predictive analytics can help companies identify potential bottlenecks, forecast demand fluctuations, optimize inventory levels, and improve logistics planning.
For example, AI-powered demand forecasting tools can analyze historical data and identify patterns, which helps businesses plan more accurately for peak demand periods or seasonal fluctuations. AI can also be used to optimize routes in transportation logistics, reducing lead times and transportation costs. By implementing these solutions, manufacturers can make more informed decisions, improve efficiency, and reduce costs across their supply chains.
3. Create a strong data integration framework
A critical step in implementing M4.0 solutions is ensuring that the systems and data sources are properly integrated. Manufacturers should invest in data integration technologies that can connect disparate systems and allow for seamless data flow. This includes integration between legacy systems and new, cloud-based platforms, as well as between internal systems and those of external partners.
The concepts of data governance and master data management are increasingly important. Ensuring data completeness, standardization, and integrity is required to ensure the effectiveness of data-enabled capabilities of M4.0.
4. Manage change effectively
Adopting new technologies in supply chain management often involves significant change, not only in terms of technology but also organizational processes and culture. Managing this change is crucial for ensuring successful technology deployment and adoption. Manufacturing executives should focus on the following strategies to ensure smooth implementation:
- Stakeholder buy-in: Engage key stakeholders—supply chain managers, IT, procurement, and finance—early in the process to gain their support. Ensuring alignment across the organization will increase the likelihood of successful adoption.
- Training and upskilling: Provide employees with the necessary training to operate new systems effectively. Equip the workforce with the skills to use data analytics tools, AI applications, and new supply chain management platforms.
- Iterative deployment: Rather than a big-bang approach, consider rolling out new technologies in phases, starting with a pilot program. This allows the organization to test solutions in real-world scenarios, measure their effectiveness, and make adjustments before full-scale implementation.
Best Practices for Accelerating Technology Adoption
To speed up the deployment of M4.0 solutions and maximize return on investment, manufacturers should adopt three best practices:
1. Start with a clear vision and roadmap
Before implementing any technology, manufacturers should define a clear vision for what they want to achieve with M4.0 in their supply chain. This vision should align with the company’s broader business objectives, whether that is reducing costs, improving customer satisfaction, or enhancing sustainability. A well-defined roadmap will outline the necessary steps, timelines, and milestones for deployment. This roadmap should be flexible enough to adapt to changes in technology and business needs over time.
2. Collaborate with technology partners
To ensure successful deployment, manufacturers should collaborate closely with technology vendors, consultants, and integrators. These partners can help navigate the complexities of system integration, provide industry-specific insights, and accelerate the adoption of best practices. By choosing experienced technology partners, manufacturers can reduce implementation risks and ensure that their M4.0 initiatives are aligned with current technological trends.
3. Focus on scalable solutions
Manufacturers should prioritize scalable solutions that can grow with their business needs. M4.0 technologies should not be seen as a one-time investment but rather as an ongoing journey. By choosing flexible platforms and technologies that can scale and adapt over time, manufacturers can future-proof their supply chains and ensure they are well-positioned to take advantage of emerging technologies and evolving market conditions.
Embracing M4.0 for Supply Chain Innovation
In conclusion, deploying M4.0 technologies in supply chain management offers manufacturers an opportunity to improve efficiency, enhance resiliency, and gain a competitive edge. The complexity of interconnected systems, data integration, stakeholder coordination, and legacy systems must be carefully managed to achieve success, however. By adopting cloud-based platforms, using AI, ML, and creating strong data integration frameworks and effectively managing change, manufacturers can accelerate solution design, deployment, and adoption. With a clear vision and strategic approach, M4.0 can further a new era of supply chain innovation and performance. M
About the author:
Michael Platz is a senior leader of the Supply Chain Consulting practice at NTT DATA.
All Aboard the AI Train as Manufacturing Leaders Embrace the Future
The Manufacturing Leadership Council’s 2024 Future of Manufacturing Project event drew forward-thinking manufacturing leaders to Orlando, Fla., for a deep dive into AI-centered factories of the future. The Future of Manufacturing Project is an on-going initiative focused on key trends manufacturers must understand today to help them realize a better future.
David R. Brousell, MLC’s Founder, Vice President and Executive Director, opened the event with a sweeping overview of AI’s transformative role in the manufacturing sector and beyond. He began by highlighting the explosive growth of generative AI tools like ChatGPT, which became a global phenomenon almost overnight. Brousell explained how this rapid adoption of AI has served as a critical inflection point, fueling innovation, competition and investment at unprecedented levels across industries and nations. He underscored the staggering economic potential of AI, citing projections that it could contribute nearly $20 trillion to the global economy by 2030.
“A worldwide competition for dominance in AI is underway — and it appears to be intensifying,” Brousell said.
Major investments and initiatives by the U.S., China and Saudi Arabia highlight the AI race. He illustrated how these efforts reflect the high stakes of AI’s development, with nations and corporations alike vying for leadership. However, he cautioned against overhyped expectations, acknowledging the historical challenges of AI adoption and the – unlikely as it may seem – potential for a third AI winter. To navigate these uncertainties, he emphasized the importance of understanding both the opportunities and the risks that AI presents.
Drawing from MLC research and recent industry studies, the opening address included key findings on how manufacturers perceive and are implementing AI. While most manufacturers recognize AI as a game-changing technology, only a small percentage rate their current AI maturity as advanced. Despite this, many companies are ramping up their investments and seeing early signs of positive returns, particularly in areas like operational efficiency and productivity. Brousell also identified significant challenges, such as data quality, skill gaps and the need for metrics to measure AI’s impact.
To guide manufacturers in their AI journeys, Brousell offered practical recommendations: think big and envision bold possibilities, stay informed on technological advancements, collaborate with industry communities, establish ROI metrics and prioritize a human-centered approach to AI adoption.
He closed by reflecting on the manufacturing sector’s historic role as a driver of societal progress, calling on leaders to define their partnership with AI responsibly. His message was clear: the AI train is leaving the station, and manufacturers must be ready to board.
Access full text and a video of David R. Brousell’s opening address.
Future of Manufacturing Project: The Growing Competition for AI Supremacy
The Future of Manufacturing Project has become the premier platform for exploring the transformative forces shaping the manufacturing industry. At the 2024 event, David R. Brousell, MLC’s Founder, Vice President and Executive Director, delivered a powerful opening address that set the tone for the discussions and presentations to come. In his speech, Brousell examined the meteoric rise of AI technologies like ChatGPT, the growing global competition for AI leadership, and the profound implications for manufacturers. He offered data-driven insights, candid reflections on challenges, and practical guidance for navigating the AI-driven future. Below is a recording and the full text of his address — an essential read for anyone seeking to understand the opportunities and responsibilities that lie ahead for manufacturing and beyond.
Good morning, everyone, and welcome to the fourth annual Future of Manufacturing Project event.
Two years ago in Nashville, when MLC first devoted this event to examine the potential as well as challenges with artificial intelligence in manufacturing operations, OpenAI had just released the generative AI tool ChatGPT.
I had mentioned OpenAI in my talk that day, but little did we know what would soon be coming. ChatGPT took off like a rocket ship, capturing the imagination of the public, businesses, and governments, raising both aspirations as well as fears about the technology.
ChatGPT reached 100 million users in just two months after its launch, becoming the fastest growing consumer application in history at that time. Today, ChatGPT has a reported 200 million users.
ChatGPT became an inflection point for AI in general. The energy and momentum around AI in its many forms – IBM alone has identified seven types of AI — have not only continued but appear to be picking up even more steam. In the generative AI market alone, a flood of competitors has joined ChatGPT including Midjourney, Stable Diffusion, Bard, and, of course, Copilot, and others.
The amount of literature being produced about AI – and I use the term “literature” with an asterisk attached – is almost impossible to keep up with. Every day, it seems, there are multiple newspaper and magazine articles, research reports, case studies, government reports, product and service announcements, and conferences extolling both the virtues and warning of the challenges with the technology.
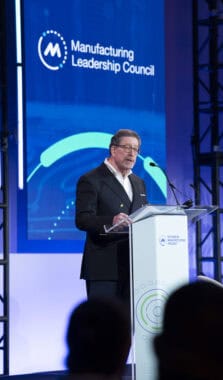
Huge sums of money are flowing into the AI market as nations earmark funds, announce national strategies, and set up organizations to capitalize on the technology; as technology and service providers produce new AI and augmented products and offer implementation methods; and as businesses assess the effect of AI on their competitive posture and develop their own goals and objectives with the technology.
As I said in Nashville two years ago, a worldwide competition for dominance in AI is underway – and it appears to be intensifying.
Here are a few datapoints to consider:
- In a report published in September, International Data Corp. predicted that AI would have a cumulative global economic impact of $19.9 trillion through 2030, representing 3.5% of global GDP by that year. Every new dollar spent on business-related AI solutions and services will generate, IDC estimated, $4.60 into the global economy, in terms of indirect and induced effects.
- Meanwhile, spending on AI is growing at double-digit rates. IDC estimated that worldwide spending on AI, including AI-enabled applications, infrastructure, and related IT and business services, will grow at a 29% compound annual growth rate between this year and 2028, reaching $632 billion in 2028. In terms of use cases, Smart Factory floor investments are expected to grow at a 32.5% CAGR in that time period.
- The number of AI patents granted worldwide rose 62.7% between 2021 and 2023, according to the 2024 Stanford AI Index Report. In 2022, China led global AI patent origins with a 61.1% share, significantly outpacing the U.S., which accounted for 20.9% of AI patent origins. In that same year, however, the U.S. far exceeded China in terms of financial investments. In the U.S., AI investments reached $67.2 billion in 2022, 8.7 times more than China.
- Announcing AI strategies has become commonplace among nation states. The U.S. has its National AI Initiative Act, a Presidential Executive Order on AI, and is in the process of standing up an AI Institute under the America Makes program.
- In China, well, we know there is much activity but getting reliable data is a challenge. For example, International Data Corp. estimates that China’s investment in AI will reach $38 billion in 2027. Meanwhile, a report in Yahoo Finance in September said that China’s AI industry could invest $1.4 trillion into developing the technology in the next six years. Pass the salt!
- On the other side of the world, the European Union has its AI Innovation Strategy, which calls for the creation of AI Factories across the European Union. Back in Asia, India has its AI Mission initiative; Japan’s Ministry of Economy, Trade and Industry has allocated $740 million to subsidize the AI computing industry in Japan; and South Korea has a plan to spend $6.9 billion by 2027 on artificial neural processing units and high bandwidth memory chips.
But one of the more notable announcements this year came from Saudi Arabia, which created a $100 billion fund to invest in AI and other technologies.
***
Now, cautious observers, particularly those with a historical context, might say that all the posturing and the financial projections and investment numbers merely reflect an inflated state of expectations, a euphoria born of excitement about the shiny new car. We are in what Gartner calls a hype cycle that will, in time, deflate as the challenges of implementing and optimizing use of the technology become better understood and experienced.
No doubt there is some truth in this. As we know from the experiences we’ve had with other technologies, strategic, organizational, cultural, and human behavioral challenges govern whether a technology project will ultimately be successful. I myself have talked for many years about what I call The Absorption Gap – the time and effort required to go from implementation to benefit. It often takes much longer to get to benefit than we think at the outset.
Could history repeat itself with AI? Could we yet experience a third AI winter, after the two that occurred last century when expectations were disappointed?
Anything is possible, of course, but I wouldn’t bet on it. The foundational conditions that were lacking years ago to enable AI to take off – compute power, storage, communications bandwidth, sufficiently large data sets – have all been addressed. Moore’s Law will ensure that price/performance will improve, perhaps significantly, over time. And the unrelenting need to find new competitive advantage will compel companies to go forward into the known and, perhaps more importantly, the unknown.
My friends, the AI train is leaving the station – and you must be on it.
***
The encouraging news is that aspirations with AI among manufacturers are strong and there is already some evidence that AI is, as they say, worth the squeeze.
Recent MLC research shines a light on what manufacturers are thinking about the use of AI in their operations.
Among the many findings of our new AI study, results of which were published in the Manufacturing Leadership Journal in August, perhaps the most important is that a majority of manufacturers, 55%, see AI as a “game-changer” in the industry by 2030, even though only 5% at this point in time assess their maturity level with AI in operations as “advanced”.
Perhaps propelled by this perception, 78% said they plan to increase spending overall on AI tools over the next 12 to 24 months, with
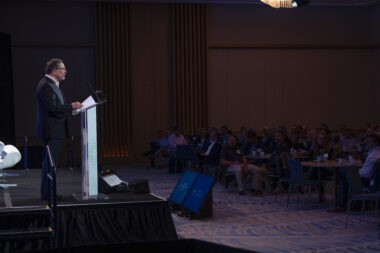
one-fifth expecting to increase their spending by more than 30%. Already, more than half are using GenAI tools such as ChatGPT or Microsoft’s Copilot, and more than 80% said they expect to increase the use of such tools in the next two years.
As manufacturers ramp up their AI investments, early indications about the impact and return on these investments are looking positive.
In July, EY, a member of the Manufacturing Leadership Council and a partner in the Future of Manufacturing Project, published the results of its first AI Pulse survey, which asked 500 senior leaders across industries in the U.S. about AI investments, impacts, and challenges.
The study found that senior leaders whose organizations are investing in AI have seen tangible results across business functions, including about three-quarters who are already experiencing positive ROI in operational efficiencies, employee productivity, and customer satisfaction.
Moreover, the EY study found that organizations that are committing 5% or more of their budgets on AI are seeing “outsized returns.”
And all of this is happening as AI continues to advance. This year’s Stanford report assessed that AI has now surpassed human performance on several benchmarks, including in image classification, visual reasoning, and English understanding. However, the technology still lags human capabilities in competition-level mathematics, visual common-sense reasoning, and planning, Stanford said.
I suppose that we can all take some comfort in those findings. We humans will continue to dominate – at least for the moment. We don’t know how fast AI will evolve and how capable and powerful it will become in the years ahead, but we know one thing for sure – with the amount of capital flowing into the AI market and the amount of effort being applied to its development, AI will not sit still.
As it continues to move, manufacturers have a host of issues to deal with to ensure that AI is used effectively to advance their organizations’ business and operational goals, and in a responsible manner.
Once again, MLC research spotlights some of the challenges.
The top concern, say 68%, is around data – quality, contextualization, and validation. More than 40% also identify AI skills and AI business case development as issues. Sixty-six percent say they do not have a specific set of metrics to measure the effectiveness and impact of AI implementations at this time.
This all goes back to that single digit finding I mentioned earlier about maturity levels with AI. The question is: what will it take to accelerate adoption and use of AI in operations and enable companies to climb up the maturity curve?
The easy answer is time, money, and persistence. But there’s a bit more to it than these three foundational elements. Please allow me to offer a few recommendations:
First, I can’t emphasize strongly enough about having a vision for what you want to do with AI. That may sound obvious, and it is on one level, but it takes a lot of thinking, strategizing and even soul-searching to craft an idea of what your company could be in 10 or 15 or 20 years.
So, think big about AI and what your company might be able to do with it. Think about the art of the possible. Think about growth and not just in what you are doing now but what you could be doing that’s new, innovative, and exciting.
At first, you will turn your attention to using AI to become more efficient, more cost-effective, faster. These are all important objectives, but don’t stop there. Imagine a better future.
And once you craft a business vision for the future – a vision that should be flexible enough to change when needed – make sure your AI strategy “functions as the fuel,” as Council member Deloitte says, for the business strategy.
Second, keep up with the evolution of the technology. There is a lot of energy and momentum around AI, as I have detailed, and new developments are occurring frequently. The technology must be monitored and understood, as well as the rules, laws, and regulations that will govern its use. Designate someone or a specific business unit in your company to keep track of developments to understand their potential to help your business.
Third, don’t try to go it alone. Plug into the community. MLC is here to bring together your fellow manufacturers, technology and services providers, academics and research organizations, and sister groups such as the IRI, CESMII, and MxD so you have access to a robust community for help.
Fourth, work on the metrics. Experimentation with AI is necessary and important, but the laws of business must be served. Find, adopt, and use ROI models to prove return so that investment continues.
Fifth, it is essential to take a human-centered approach to AI. Bring people along by showing them the benefits and being candid with them about the challenges. Change management and training are key.
Taken together, these simple, common-sense tenets can help you navigate your journey with AI.
***
What we do here today, and what you do after this conference when you go back to your companies, will help propel the manufacturing industry forward.
But our responsibility is greater than just our own industry. AI has not only the potential to reshape manufacturing but society itself. As it has many times throughout history, this industry is playing a vital role in deciding what the future might look like. Once again, the mantle of change lies at our feet.
I would like to close with a quote from a book called “The Age of AI And Our Human Future,” authored by Henry Kissinger, Eric Schmidt and Daniel Huttenlocher:
“The advent of AI, with its capacity to learn and process information in ways that human reason alone cannot, may yield progress on questions that have proven beyond our capacity to answer. But success will produce new questions … Human intelligence and artificial intelligence are meeting, being applied to pursuits on national, continental, and even global scales.
“Understanding this transition, and developing a guiding ethic for it, will require commitment and insight from many elements of society: scientists and strategists, statesmen and philosophers, clerics and CEOs. This commitment must be made within nations and among them. Now is the time to define both our partnership with AI and the reality that will result.”
Thank you and have a great conference.
About the author:
David R. Brousell is the Founder, Vice President and Executive Director, Manufacturing Leadership Council
Photos by David Bohrer / National Assoc. of Manufacturers
The Best of Rethink
The 20th anniversary of Rethink brought together thought leaders and manufacturers to share digital transformation best practices and lessons learned.
MLC members celebrated Rethink’s 20th anniversary by doing what they’ve done at the past 19 summits: learning about the technology, leadership, and organizations driving Manufacturing 4.0 forward. Over the course of three days, more than 60 speakers shared their insights during keynotes, case studies, panel discussions, (Re)Think Tanks, fireside chats, lightning rounds, and executive interviews.
Here are a few of the exceptional sessions Rethink participants experienced.
Access to the MLC Member Portal is required to view the videos.
Opening Address: What’s Next?
David R. Brousell, Founder, Vice President and Executive Director, Manufacturing Leadership Council
Over the past 20 years, the manufacturing industry has worked hard to understand and adopt the digital model of production, learning much along the way. But as new technologies emerge, the proverbial finish line continues to move, leading to new possibilities, aspirations, and opportunities as well as challenges. MLC’s founder shares his views on what manufacturing might look like by mid-century.
Case Study: 25 Years of Transformation—Triumphs and Pitfalls from a Manufacturing Digital Native
Robert Bodor, CEO and President, Protolabs, Winner of the 2022 ML Awards Small/Medium Enterprise Manufacturer of the Year
Protolabs began as a company aimed at filling a specific manufacturing need: injection molded parts really fast. As one of manufacturing’s original “digital natives,” that speed was a result of automation and digitizing a once-traditional process. Since those early days, the company has expanded its offerings and experienced significant growth. The ongoing digital transformation that followed over the next 25 years as a custom components manufacturer has provided a case study in digital evolution comprised of technology advancements, key acquisitions, difficult trade-offs, and even a few hard lessons learned along the way. Learn more about the company’s ongoing Manufacturing 4.0 journey that has driven its mission to serve customers on the forefront of innovation.
Panel Discussion: Next-Generation Leaders
Angela Accurso, Director of Workforce Programs, MxD
Marlon Alberto Gonzalez Martinez, Storage Order Management Fulfillment Team Leader, IBM
Megan McCarthy, Business Process Manager for Global Manufacturing Electrical, General Motors
Jonathan Miller, Automation and OpEx Manager, Saint-Gobain Life Sciences
Facilitator: Penelope Brown, Senior Content Director, Manufacturing Leadership Council
What’s on the minds of the next generation as they develop into tomorrow’s leaders? This panel discussion examines the hopes, aspirations, and visions for the future from some of 2024’s top finalists for the Manufacturing Leadership Awards Next-Generation Leadership award.
Case Study: Hardwiring Innovation Processes from Tech Into a 155-year-old Company
Craig Slavtcheff, Executive Vice President and Chief R&D and Innovation Officer, Campbell Soup Company
The pace and scope of innovation demanded in food by consumers continues to be high, driven by a range of macrotrends including food exploration and nostalgia, ag-tech, and sustainability. To keep pace, food companies have to innovate on how they innovate, focused on the three drivers of “speed to insight,” “speed to design,” and “speed to execution”. Campbell has looked to the world of tech, including machine learning and AI, as inspiration to transform its innovation processes, building on its 150+ year old history of inventing delicious, nutrition, safe, and affordable foods.
Case Study: Making the World a Better Home through M4.0
Regan Gallo, Director of Operations, Coated Abrasives NA, Saint-Gobain North America
With more than 145 manufacturing locations across North America alone and several acquisitions in the past few years, Saint-Gobain faces steep challenges when it comes to deploying a unified digital strategy across the organization. With takeaways applicable to manufacturing companies of any size, learn where the company has found success, where it needed to make pivots, and the progress it has made as it works to meet its mission – making the world a better home.
About the author:
Jeff Puma is Content Director for the Manufacturing Leadership Council
How Will GenAI Prompt a Step Change Toward Autonomous Supply Chains?
GenAI is a necessary tool for competitive advantage, but companies will need to navigate the risks and capitalize on the opportunities—or risk being left behind.
TAKEAWAYS:
● Most supply chain and operations executives (73 percent) are planning to deploy GenAI, yet only 62 percent have reassessed projects, and merely 7 percent have completed implementation.
● Organizations further ahead in the autonomous supply chain journey are 5.2 times more likely to see success with GenAI, further widening the digital gap.
● The greatest gains from GenAI come when projects align to a strategic vision, data are AI-ready, and value is maximized by addressing cyber and data risks.
Since 2020, the global economy has been in a new paradigm, marked by disruptions and changes that are more forceful, appear more frequently, trigger more interconnected and widespread impacts, and often strike immediately. Chief operating officers and supply chain managers know this all too well—the supply chains they oversee exist on the frontier of this high-risk climate, bearing the brunt of its disruption.
In response, many companies refocused on boosting resilience in supply chains, which involved diversifying operations across multiple countries and suppliers. Supply chains that had once been ultra-lean gained protection against external shocks—but often at the price of reduced efficiency.
Enter generative artificial intelligence (GenAI), which can help companies leapfrog their technical maturity and accelerate their path toward an autonomous supply chain. GenAI is being used to not only analyze and interpret vast amounts of data but also to create new scenarios, generate innovative solutions, and remove frictions in real-time. As a result, managers have end-to-end visibility and more human time for higher-order work.
“Companies see GenAI as a critical capability for remaining competitive in the future and are investing in it accordingly.”
Unlike traditional AI, which primarily focuses on data-driven insights and automation, GenAI can design new processes, forecast future demands with greater accuracy, and seamlessly identify the most cost-efficient routes and carriers in the event of a disruption.
But the combination of GenAI and traditional AI is a game changer in bridging the gap to self-driving supply chains, because of GenAI’s breakthrough capabilities and the ways in which the strengths of the two technologies complement each other. It is a compelling vision, but one that has so far remained elusive.
EY global research of 460 supply chain and operations executives has found that even among organizations that have started preparing for GenAI in their supply chain, only 28 percent have achieved a low-human-touch supply chain, and only 50 percent have achieved end-to-end visibility across the supply chain.
The Great AI Reset
Companies see GenAI as a critical capability for remaining competitive in the future and are investing in it accordingly. Three-quarters (75 percent) are planning to deploy GenAI in their supply chains, and 80 percent believe it can reinvent supply chains. In addition, 69 percent believe that failing to integrate GenAI into their supply chains will put them at a competitive disadvantage.
Despite this optimism, a retrenchment is taking place. In the past 12 months, 62 percent of respondents have reassessed their GenAI supply chain initiatives, and only 7 percent have gone on to complete deployment.
Why?
Two reasons:
- Concern and lack of understanding around the unique risks created by GenAI; and
- Challenges of implementing this complex technology.
Our findings suggest this reset is about achieving scale and maximizing impact. In-depth interviews with seven supply chain and operations executives revealed that it was tougher than expected to make the technical leap from proof-of-concept to GenAI at scale.
Leading the Way to Autonomous Supply Chains
Organizations further ahead on the journey to autonomous supply chains (front-runners) have created strong digital foundations that enable them to adopt and take advantage of GenAI quickly. This momentum is likely to widen the digital divide, unless those who are behind (followers) take prompt action.
GenAI is giving organizations a faster pathway to the autonomous supply chain. Front-runners are more ambitious about using GenAI in the next two years, anticipating deploying GenAI in 12 use cases for the supply chain on median compared to eight for followers. Front-runners are going beyond the supply chain to align more closely with other business functions and external parties and driving end-to-end visibility across the supply chain.
Most organizations’ supply chains (82 percent) are using both AI and GenAI across a wide array of use cases to take advantage of their different, often complementary strengths. Traditional AI is rules-based, requiring prepared datasets and predefined logic to solve business problems. GenAI is great for text-rich environments and unstructured data, creating new content based on the data it has been trained on. For example, companies using traditional AI for demand forecasting and quality optimization are finding that a GenAI layer improves accuracy and democratizes adoption of the tools.
Substantial GenAI Growth Expected
Substantial growth in GenAI is anticipated over the next two years. Given that front-runners already use traditional AI more and are more confident about future growth, the gap between them and followers is likely to widen. Looking more broadly at where front-runners are focusing the first wave of use cases, those with high GenAI deployment today and continued high anticipated use in two years will likely focus on
- Product design
- Logistic network design
- Global trade optimization
- Demand forecasting
These are areas where traditional AI has long been available but has been limited by the need for highly trained data scientists, which has made them too expensive for many. GenAI provides the benefit of a natural language, interpretive layer that can become a democratizing force that puts these tools in the hands of the workforce. These areas are also supply-chain functions with well-defined datasets, a high percentage of unstructured data and high value to be gained.
The next wave of use cases, those with lower GenAI deployment today but high anticipated use in two years, potentially includes
- Supplier management
- Production yield or quality optimization
- Risk management
- Customer service chatbots and product training
These high-growth areas also offer clear commercial returns, either by improving the speed of customer service through chatbots or reducing costs through quality optimization in manufacturing. Demand forecasting is another key area of focus for GenAI. It’s perceived to be a use case that will resolve a lot of pain points for the supply chain and offers clear metrics that can make the business case an easier sell to the CEO and the Board of Directors.
Many of these use cases suffer from back-end challenges when using traditional AI (quality optimization, predictive maintenance) and require bespoke solutions. Customer service chatbots also have a high degree of risk given the chatbots’ direct interaction with the public. Both factors may be contributing to the time horizon suggested here.
Three Actions to Overcome Implementation Challenges
Implementing GenAI in the supply chain involves a complex interplay of technical, organizational, and operational challenges. Organizations should take the following three action steps to advance their journey to the autonomous supply chain.
1. Align people and investments to strategic vision
For front-runners, the top factors for success in GenAI deployments are securing support from leadership (67 percent), building support from third parties (65 percent), and availability of technical talent (64 percent). The largest gaps between front-runners and followers are in prioritizing the strategic vision and support from third parties, which highlight the importance of a cohesive vision and external support in ensuring GenAI pilots and implementations are focused on delivering business value.
A cohesive, strategic vision can clarify investment priorities against the seemingly endless list of use cases of GenAI, minimize the risk of multiple business units duplicating investments, and improve AI outcomes by guiding the augmentation of large language models (LLMs) with reusable algorithmic patterns, such as retrieval-augmented generation.
2. Prioritize data readiness when considering use cases
The demands of GenAI are shining a spotlight on the myriad complexities of enterprise data management. Despite data availability, quality, and privacy being top of mind when prioritizing use cases, organizations are still struggling. Maintaining data quality is the number one implementation challenge cited by respondents (38 percent), with access to data (33 percent) another top challenge.
Any organization hoping to compete in GenAI needs to get its data house in order, by prioritizing data cleansing, standardization, systems, and engineering to reduce latency, and enhancing metadata so data can be consumed by retrieval augmented generation (RAG) systems to improve the accuracy of GenAI outcomes.
3. Maximize GenAI value by mitigating cyber and data risks
GenAI is a nascent technology, so it is not surprising that 40 percent of respondents say their organizations do not fully understand the new risks and challenges of GenAI in supply chain. GenAI poses new vulnerabilities—for example, through prompt injection attacks designed to provoke LLMs into leaking sensitive data or manipulate their outcomes. Front-runners are more likely than followers to focus on the new risks that GenAI poses, such as inaccuracies and hallucinations, exposure to legal liability through IP infringement, overreliance on untested technology, brand or reputation damage and job insecurity.
The need for stronger cybersecurity is paramount as organizations look to deploy GenAI. Supply chain and operations leaders need to work closely with cybersecurity teams from the beginning to help with the secure adoption of GenAI in the supply chain. This includes embedding the cyber team in use case identification and governance to ensure the value potential of GenAI in the supply chain is maximized.
GenAI’s Potential and Pitfalls
GenAI is emerging as a transformative tool, enabling more autonomous supply chains with end-to-end visibility and real-time problem-solving capabilities. EY research suggests that GenAI adoption is crucial for competitiveness, but challenges in understanding risks and implementation complexities have led to a strategic reassessment. Front-runners in GenAI adoption are leveraging it for improved demand forecasting and operational efficiency. To overcome these challenges, companies must align strategic visions, prioritize data readiness, and mitigate cyber risks. For more information, visit Will GenAI accelerate autonomous supply chains? | EY – US. M
Authors:
Ayoub Abielmona is an EY Global GenAI Supply Chain Leader.
Matthew Burton is an EY EMEIA Supply Chain and Operations Leader.
Sumit Dutta is Principal, Supply Chain & Operations, Ernst & Young LLP.
David Guarrera is Principal with EY Americas Technology Consulting, leading Generative AI initiatives.
Jocelyn Hallum is EY Global Supply Chain Transformation, Planning and PLM Transformation Solution Leader.
Glenn Steinberg is EY Global Supply Chain and Operations Leader.
MLC Research Shines a Light on the State of M4.0
Over the course of 2024, five research studies depicted an industry still very much in transition to the digital model of manufacturing.
Ever since its founding in the early 2000s, the Manufacturing Leadership Council has been devoted to using survey research to help take the pulse of digital transformation in manufacturing. Regularly surveying the operational leaders who are leading digital efforts in their companies has yielded insights into digital maturity levels, how advanced technologies are being used, what challenges manufacturers are having in embracing the digital model, and their aspirations for the future.
MLC survey research is usually associated with the Council’s Critical Issues agenda, but with the launch of the Future of Manufacturing Project in 2021, the scope of research widened to include more topics and in-depth white papers.
This pattern continued in 2024, a year in which MLC fielded five major Manufacturing 4.0-related research studies – on Smart Factories; Digital Maturity; Data Mastery, a white paper under the Project; Artificial Intelligence; and Leadership, Culture, Organization, and People.
In aggregate, MLC research for 2024 suggests that manufacturers are at an intermediate stage with M4.0 adoption, with many still working at redesigning their organizational structures, adapting their company cultures, scaling the use of new technologies, and growing leadership skills and behaviors for the digital age.
Here are the key findings of MLC’s research during 2024, as published in the Manufacturing Leadership Journal and in white paper format:
“Smart Factories Are Still a Work in Progress”, February MLJ – This study chartered the stage of M4.0 adoption and found that most respondents are at an intermediate stage in their transformation journeys. The study also found that the most significant roadblocks to implementing a smart factory strategy are organizational structures or cultures that resist change.
“For Manufacturers, Digital Maturity Hasn’t Yet Come of Age”, MLJ April 2024 – A key finding was that a majority of manufacturers either do not have a formal process for measuring their digital maturity or do informal benchmarking. Another important finding was that a majority of manufacturers have a digital maturity target of Manual Execution with Digital Support, with an equal balance of companies on track or behind schedule on this goal.
“Data Mastery: A Key to Industrial Competitiveness”, White Paper, June 2024 – This White Paper, under MLC’s Future of Manufacturing Project, examined data governance and organization, how manufacturing data is being used, the business impact of the data, and future trends. A key finding of the study was that 86% of respondents said that the effective use of manufacturing data in their organizations will be “essential” to their competitiveness in the future.
“Manufacturers See AI as a ‘Game-Changer’ as They Ramp Up Investments”, MLJ August 2024 – No doubt spurred by the interest in generative AI, 78% of manufacturers said they plan to increase spending on AI tools in the next two years. And a majority of respondents, 56%, said they expect AI to change the rules of the industry by 2030, despite issues with data and a lack of AI-related workforce skills.
“Leadership Preparedness Improves, But Gaps Remain”, MLJ October 2024 – Understanding digital roles and skills for the future manufacturing enterprise is only somewhat understood, said 71% of survey respondents. To change that equation, digital manufacturing leaders need to augment traditional leadership qualities with new skills such as fostering a data-driven culture and guiding the workforce through change. M
About the author:
David R. Brousell is the Founder, Vice President and Executive Director, Manufacturing Leadership Council
The Best of the Manufacturing Leadership Journal
Celebrating expert insights from a year of manufacturing innovation and leadership.
As the manufacturing landscape continues to evolve, 2024 brought a wealth of insights, breakthroughs, and transformative ideas to the forefront. From exploring the practical applications of digital twins to harnessing the potential of Generative AI, the articles featured in this year’s best-of issue highlight the ingenuity driving Manufacturing 4.0 forward. The Manufacturing Leadership Journal not only showcased cutting-edge technologies but also delved into the leadership practices, workforce strategies, and cultural shifts necessary to thrive in an era of rapid change.
In this special compilation, we revisit some of the most compelling narratives of the past year. Discover how manufacturers are leveraging AI and machine learning to optimize operations, using smart technologies to attract a new generation of talent, and adopting innovative frameworks for digital transformation. Whether you’re looking to refine your Manufacturing 4.0 approach, improve organizational alignment, or tackle workforce challenges, these articles offer a roadmap for success in the dynamic world of modern manufacturing.
While every article published in the Manufacturing Leadership Journal is chock full of ideas and information that is worth exploring, here are a few of 2024’s best articles.
FEBRUARY / MARCH 2024
SMART FACTORIES AND TRANSFORMATIVE TECHNOLOGIES
How Digital Twin Applications Can Help Manage and Optimize Inventory
By Casey Chapman and Joe Krause, RSM US LLP
Discover how a midsize industrial food production company leveraged digital twin simulations to optimize operations, avoid unnecessary capital expenditures, and enhance decision-making. Highlighting the transformative potential of digital twins, this case study offers insights into improving inventory management, mitigating risks, and driving profitability in a competitive manufacturing landscape.
Connect, Analyze, Improve — Then Rinse and Repeat
By Randal Kenwothy and Kris Slozak, West Monroe and AJ Alexander, Sorba.ai
Struggling to start your smart manufacturing journey? This article explores how connecting equipment and using machine data to solve specific problems can build a strong foundation for Manufacturing 4.0 success, with real-world case studies demonstrating rapid ROI and expanded operational benefits.
APRIL / MAY 2024
THE M4.0 MATURITY CURVE
GenAI Ushers Human-Like Intelligence in Manufacturing
By Amol Adgaonkar, Microsoft; Gonzalo Chavez, Alex Lemken and James Zhang, PTC; and Howard Heppelmann, PTC ThingWorx IoT Solutions
Generative AI is set to revolutionize manufacturing by democratizing data analysis, automation, and problem-solving across the value chain. By leveraging techniques like Retrieval Augmented Generation (RAG) and building a unified, contextualized data foundation, manufacturers can identify high-impact use cases, pilot innovative solutions, and drive significant productivity and efficiency gains.
Unlocking Manufacturing Excellence with the M4.0 Maturity Model
By Roberto Cisneros and Krishnan Venkat, Softtek
The Manufacturing 4.0 maturity model provides manufacturers with a structured framework to assess readiness and capabilities across key dimensions, including supply chain management, technology, sales and operational management, and knowledge, skills, and attitude. By uncovering gaps and setting actionable goals, the model empowers organizations to navigate technology adoption, foster continuous improvement, and achieve operational excellence.
JUNE / JULY 2024
CHANGE MANAGEMENT IN THE M4.0 ERA
The Human Factor in Industry 4.0: Capability-Led Change
By Mike Doheny, Roberto Migliorini, Ewelina Gregolinska and Justin Grover, McKinsey
A leading global CPG company successfully scaled its digital transformation by prioritizing people, aligning organizational goals with tailored learning programs, and fostering a culture of continuous capability building. Through a structured approach that emphasized local and global collaboration, measurable outcomes, and on-the-job application, the initiative achieved a double-digit uplift in throughput across its manufacturing network while enhancing employee engagement and talent retention.
Technology as Manufacturing’s Skills and Applicant Solution
By John Coykendall, Deloitte LLP; Victor Reyes, Deloitte Human Capital; Kate Hardin and John Morehouse, Deloitte Research Center for Energy & Industrials; Gardner Carrick, The Manufacturing Institute
The U.S. manufacturing industry is experiencing robust growth, yet persistent workforce challenges threaten its trajectory, with nearly 1.9 million manufacturing jobs potentially going unfilled by 2033. By leveraging technology to attract, engage and empower workers — through flexible work arrangements, advanced training tools, and high-tech environments — manufacturers are innovating to address the talent gap and meet evolving workforce expectations.
AUGUST / SEPTEMBER
AI IN MANUFACTURING
Unlocking AI and ML’s Potential in Manufacturing
By Adrian Wood, Dassault Systèmes
Artificial intelligence and machine learning are transforming manufacturing by optimizing production execution, predictive maintenance, and scheduling, resulting in improved quality, reduced downtime, and greater operational efficiency. Successful implementation requires robust data management, collaboration, scalable pilots, and workforce training, while emerging technologies like GenAI and autonomic systems promise to revolutionize self-optimizing operations and model development.
Exploring the Potential Value of Generative AI Throughout Manufacturing
By John Coykendall, Deloitte LLP; Kate Hardin, John Morehouse and Kruttika Dwivedi, Deloitte Research Center for Energy & Industrials
Generative AI represents a transformative step in the manufacturing industry’s digital evolution, with potential applications in product design, training, supply chain management, and aftermarket services. By leveraging GenAI, manufacturers can enhance efficiency, resilience and innovation, addressing challenges like labor shortages and operational disruptions. As companies explore this technology, they stand to unlock significant value and strengthen their competitive position.
OCTOBER / NOVEMBER 2024
LEADERSHIP, CULTURE, ORGANIZATION, PEOPLE
Effective Digital Transformation Leadership Practices
By Chris LeBeau, Advanced Technology Services, Inc. (ATS)
Successful digital transformation in smart factories requires clear problem-solving focus, flexible frameworks, and strong leadership. By leading through influence, celebrating small wins, and fostering collaboration, leaders can drive cultural alignment and organizational buy-in. This approach, combined with scalable solutions and trusted expertise, ensures smart factory initiatives deliver lasting value and support long-term strategic goals.
How Manufacturers Can Use Industry 4.0 to Attract Gen Z
By Buddhi Ratawal, Dassault Systèmes
Industry 4.0 technologies offer manufacturers innovative ways to attract Gen Z talent by aligning with their values of autonomy, collaboration, and purpose-driven work. By leveraging data-driven empowerment, eliminating repetitive tasks, and embedding sustainability into operations, manufacturers can redefine worker experiences and improve their appeal to the next generation of the workforce.
About the author:
Jeff Puma is Content Director for the Manufacturing Leadership Council
The Best of the Future of Manufacturing Project
The Future of Manufacturing Project provides a how-to guide for manufacturers to achieve future success
As manufacturing accelerates into a future defined by rapid innovation and transformative trends, the Manufacturing Leadership Council’s Future of Manufacturing Project has become an essential guide for industry leaders. This project continues the council’s longstanding mission to challenge manufacturers to “imagine a better future.” Through curated insights, the Future of Manufacturing Project brings clarity to the shifts that will shape industry in the years to come.
We’ve gathered some of 2024’s best articles and webinars from the Future of Manufacturing Project, each delving into critical developments that every manufacturer should know. From groundbreaking tech advances to leadership strategies for an evolving workforce, these pieces reveal how embracing change isn’t just necessary – it’s the key to thriving in manufacturing’s next era.
Future of Manufacturing Project: The Coming Data Value Revolution – Reflections on Nashville Panel Discussion
Listen in as this expert panel addresses some of the most important aspects of how manufacturers can leverage operational data to enhance their competitiveness today and in the future, including:
- What the future holds for data monetization, data ecosystems and data-driven innovation;
- How data-driven manufacturing will reshape the future workforce;
- How AI, data visualization, and industrial metaverse technologies will redefine operational excellence;
- And, drawn from the interactive sessions at the event, what manufacturers are planning to do now to prepare themselves for a data-centric future.
Access to the MLC Member Portal is required to view this video.
Seeing the Unseen: Observability in Manufacturing
Eric Danzinger, CEO and Co-Founder, Invisible AI
What if manufacturers could achieve the same deep visibility into operations as software engineers do with code? Explore how the principles of software observability are transforming manufacturing, making real-time monitoring and data-driven improvements possible on the factory floor.
Placing Innovation at the Heart of Transformation
David Takeuchi, Global Strategy and Transformation Business Model Innovation Leader, EY
Jerry Gootee, Global Advanced Manufacturing Sector Leader, EY
Claudio Knizek, Global Advanced Manufacturing and Mobility Leader, EY-Parthenon
To build the right foundation for long-term growth, manufacturers must put innovation and digital strategies at the heart of transformation.
Crystal Ball: Business Resilience in 2030 and the Digital Dexterity Effect
Siva Gurupackiam, Senior Vice President of Manufacturing Industry Solutions, NTT DATA
Digital transformation is today’s tested and proven path to business resilience. This era is defined less by permanence than by alacrity of adaptation. Manufacturers must already evolve their approach to business resilience to remain competitive.
About the author:
Jeff Puma is Content Director for the Manufacturing Leadership Council