The Need to Accelerate Industrial AI Adoption By 2030
AI will become a key player in driving manufacturing competitiveness in the years ahead.
David R. Brousell, Co-founder of the NAM’s Manufacturing Leadership Council, called on all manufacturers to accelerate their understanding and use of new Artificial Intelligence (AI) technologies in his opening speech at the MLC’s latest Manufacturing in 2030 event, Let’s Talk About AI, in Nashville, Tenn., this morning.
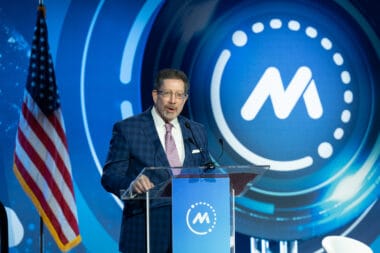
“The stakes for our industry and our country couldn’t be greater as our economy becomes increasingly digital,” asserted Brousell. “Global competition for dominance in AI is underway, with manufacturing as a key player in the race. Our competitiveness as an industry at home and abroad will increasingly be defined by AI expertise, application, and experience – and in a trusted and responsible way.”
Technologically, he noted, AI is finally coming into its own after a long development period and researchers now estimate that the value of the global AI industry will rise rapidly from $93.5 billion in 2021, to a substantial $1.8 trillion by 2030.
AI is also a pervasive technology, he continued, meaning that it will be incorporated into many other technologies including semiconductors, software applications and platforms, and communications equipment. It will increasingly power the operation of front-office applications, ERP, PLM, MES, CRM and other key operational applications. Robotic systems, too, will increasingly be guided by AI.
And although only 9% of respondents to a recent MLC study said that they saw AI and ML as a game-changer for the industry today, by 2030, 53% said that they believed it would indeed become a game-changing transformative force.
Citing a U.S. Patent Office report from October 2020 that stated, “AI is poised to revolutionize the world on the scale of the steam engine and electricity”, Brousell stressed that’s why manufacturers now need to better understand how AI may shape how they run their factories and plants, how it will influence their workforce strategies, what business benefits may attend AI use, and what challenges the industry must overcome to realize its potential in the years ahead.
However, “as with any important technology,” he added, “let alone one as unique as AI, there will be a learning curve replete with twists and turns.”
One of those twists is the fact that AI remains a controversial technology. Some see it as an existential threat to humanity; others see it unleashing a new wave of productivity and efficiency and enabling people to have better and more rewarding business lives.
Brousell also believes that AI’s unique ability to learn, and what that ability implies for predicting machine and operational patterns and behavior, qualifies it to be “in a special place among all of the technologies associated with digital transformation.”
“I can’t think of another technology that we have employed in our factories and plants,” he added, “that requires us to ask the question: do we need a code of ethics for AI use?” According to recent MLC research, he noted, 75% of manufacturing executives already believe a code of ethics will be needed in the years ahead.
Nevertheless, predicted Brousell “AI is here to stay”, and that its influence will only grow in operations, in the workforce, in the interactions across supply chains, and with customers and partners in the years ahead.
“AI”, he concluded, “is truly a force to be reckoned with” for the future of manufacturing, and manufacturers now need to act with urgency to accelerate its adoption to drive competitiveness in the years ahead.
Ten Takeaways from M in 2030 Project A Lens on the Future Panel
With the recent release of the Manufacturing in 2030 Project survey A Lens on the Future, MLC assembled an expert panel of speakers to discuss the results and bring context to the survey findings.
Moderated by David R. Brousell, the MLC’s Co-Founder, Vice President and Executive Director, the panel included Dennis McRae of West Monroe, Greg Wagner of EY, Chirag Rathi of Infor, and Joe Zakutney of NTT DATA.
Here are ten key takeaways from the discussion:
1. 84% of survey respondents expect an increased pace of digital adoption in the next decade
Dennis McRae, West Monroe’s Senior Partner and Practice Leader, Consumer & Industrial Products, noted that the acceleration rate depends on the size of the company, but he said the biggest thing is if you haven’t started your Manufacturing 4.0 journey in a meaningful way, now is the time to do it to maintain competitiveness.
“One of the first things you can do is really establish a team focused on digital with the right leaders, the right innovators, and also the right disruptors who can really challenge and do what leadership needs to get done,” McRae said.
2. 58% of survey respondents have autonomous factory operations on their radar by 2030
Chirag Rathi, Senior Director, Industry and Solution Strategy at Infor, pointed out that the desire for autonomous operations is not new and that General Motors was talking about this in the 1980s. Citing deep-learning and self-learning algorithms in machine learning, digital twins, blockchain, and autonomous transportation, he sees autonomous operation as a game changer, but he cautioned that full autonomy is unlikely in many circumstances.
“The cost of doing full autonomy in most industrial manufacturing processes might be too high,” he said. “So you will have part autonomy in several manufacturing arenas where the business case makes sense, but it will be a decision made on a case-by-case basis.”
3. 76% believe manufacturing should adopt an AI code of ethics
For Joseph Zakutney, NTT DATA’s Vice President, Manufacturing Industry Consulting and Digital Transformation, thinking about AI’s future means protecting against biases and cyberattacks, while accounting for safety.
“Procedures will need to be put in place to make sure that we’re complying to [a code],” he said. “We need to make sure that the software that we are releasing is fair, reliable, explainable, takes data protection and government regulations into consideration, and is focused on the well-being of society.”
4. Almost half of respondents indicated they expect workforce shortages to continue through 2030
Traditionally, manufacturing doesn’t have the best image according to Greg Wagner, EY’s Data Driven Manufacturing Leader. It is considered dirty, loud, and can be physically intense at times, but Wagner pointed out that those seeking purpose-based work should be attracted to manufacturing. The old adage of “being a cathedral maker and not a bricklayer” fits here, according to Wagner.
“If we change the paradigm and what we’re looking for, the types of job experiences we can give people, and use automation to get rid of some of those menial tasks that people don’t enjoy and free up their capacity to focus on bigger problem solving, it will mean more impactful types of roles,” Wagner said. “That’s going to really start to attract people and start to soften some of that gap we see right now in hiring.”
5. 81% of manufacturers are looking for greater speed and flexibility
When we think about speed and flexibility, what people really want is responsiveness, according to Wagner.
“If we really want to be able to respond quicker, we need to know what’s going on and we need to invest in better end-to-end visibility of what’s happening within our factories and what’s happening across our network so that we can be more adaptive and have the right insights to drive that change,” he said.
McRae added, “There’s a big opportunity for manufacturers in terms of connecting with their customers, building that client experience, and really monetizing a lot of the data that’s already in the business.”
6. By 2030, 50% of respondents believe digital adoption will be a game changer.
For Rathi, we’ll be closer to Industry 5.0 by 2030 with hyper-customization, responsive and distributed supply chains, and business model innovations. In fact, Rathi said we already have the building blocks to make this a reality.
“We have certainly got a lot of the raw materials to make that transformation happen,” he said. “So we will have a lot of transformative changes by that time period.”
7. Digital acumen is important across functions and at various leadership levels
One challenge McRae sees is getting everyone on the same page using the same language so that data assets are understood across company levels by all who manage data and products in the business.
“It’s not just around different levels, but also across functions,” added Wagner, noting that there is a wide array of digital understanding and how those technologies can be applied.
8. Hiring for a digital background versus a subject matter expert depends on the project
“I think we’re seeing the rise of data scientists and citizen data scientists at some organizations. At the same time a lot of data DIY products are becoming available, and they will become more prevalent by 2030, meaning that people with subject matter expertise will be able to basically design and develop their own data science projects,” Rathi said. Because of that, he believes subject matter experts will be in higher demand in most cases vs. data scientists.
9. For companies slowing down their digital projects due to the economy, focusing on specific things can help prevent losing ground
“Digital adoption is really a people play right now,” said Zakutney. “I’d stay focused on people and process, because ultimately, that’s what you’re going to end up automating [when funding comes back].”
“You can’t quit your digital investments,” added McRae. “If you don’t become digital, you’re going to be left behind. At the same time, prioritizing those digital investments specifically around areas that are going to improve your customer experience and take costs out over the next few years are going to help you win.”
10. Beyond the panel discussion, the survey report provides insightful data about the future of manufacturing
The MLC’s Manufacturing in 2030: A Lens on the Future research survey includes front-line insights from over 260 senior manufacturing industry executives, spanning multiple functional roles, and representing large-, medium-, and small-sized manufacturing companies from multiple industry sectors. Armed with this rich combination of real-world predictions and forward-thinking understandings, the MLC hopes that manufacturers can better plan their longer-term future and find ways to enhance their value, competitiveness, and contribution to society. Download the complete survey data and report.
M2030: Making Business Model Innovation a Priority
Manufacturing Leadership Journal content and MLC resources are exclusively available to MLC members. Please sign up for an account or log in to view this content.
The Next Phase of Digital Evolution: Business Model Innovation
Data mastery and AI are key drivers for the future of manufacturing, say industry experts during a discussion of the MLC’s new Manufacturing in 2030 Project white paper.
“Manufacturing is poised to unleash the next engine of production,” declared Manufacturing Leadership Council (MLC) Co-Founder David R. Brousell, in his opening remarks at the recent launch of the MLC’s white paper on the future of the industry, Manufacturing in 2030: The Next Phase of Digital Evolution.
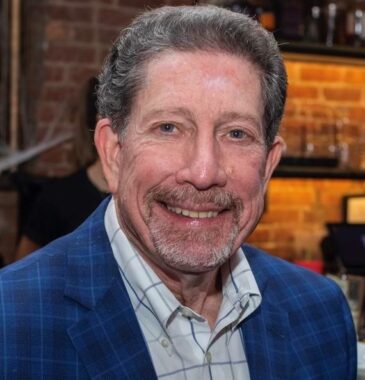
The pandemic taught us, noted Brousell, that manufacturing needs to be able to act with greater agility and be better prepared for future disruptions, whatever form they may take. The MLC recognized the urgent need for manufacturers to take a longer view of things to come, he explained. The Manufacturing in 2030 Project has been created to help enable those companies to envision what manufacturing might look like by the year 2030, to better plan their future, and to help their leaders find new ways to enhance value, competitiveness, and their contribution to society.
Brousell was joined by a panel of industry experts from Manufacturing in 2030 Project partners EY, Infor, NTT DATA, and West Monroe, plus MLC Board Vice Chair, Dan Dwight, CEO of the Cooley Group. They went on discuss key highlights from the forward-looking 52-page white paper, which explores the multiple megatrends and industry themes that will dominate the manufacturing world by 2030, from demographic shifts and global economic trends to rapid advances in technology, new approaches to workforce development, and the importance of greater sustainability.
Optimism for the Future
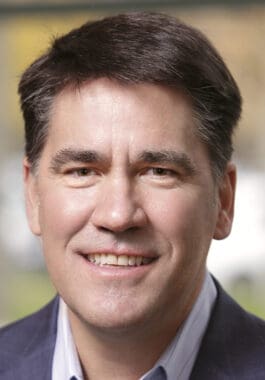
“For me, there are three reasons for optimism about manufacturing’s future,” commented Randal Kenworthy, Senior Partner and Consumer and Industrial Products Practice Leader at West Monroe. “The levels of investment in digital solutions that we are already seeing in manufacturing, the widespread recognition that manufacturing is essential to the future of the U.S. economy, and the opportunity to address one of the most existential challenges facing mankind: climate change. We have to solve this. Failure is not an option.”
“I certainly think digital is going to be the way of operation for survival in the future,” added Baskar Radhakrishnan, Senior Director, Manufacturing Industry Solutions at NTT DATA. Many of today’s factories are almost unrecognizable from the way they were 10 years ago, he observed. “And the industry is only going to continue to evolve. So, I envision future manufacturing organizations to be data driven digital enterprises, fully hyperconnected, with more distributed, agile, and value-driven ecosystems.”
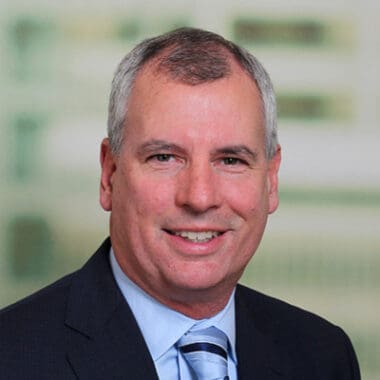
Highlighting the impact of the numerous disruptive forces at play today, Brad Newman, Advanced Manufacturing & Mobility Industry Market Leader, Americas, at EY, noted that, “The collective sum of all those forces is creating a bias for action and a need for change.” The industry already has the building blocks and the technology tools in place today, he continued, “which will help business models to evolve to ensure functions are more connected and help build better and smarter products for people in more sustainable ways. All of this will make the manufacturing industry much more rewarding for all the workers and stakeholders involved.”
The Challenge of Complexity
While the accelerated adoption of ever-more powerful and intelligent digital technologies over the next few years will underpin many of those transformational changes, the huge increase in the volumes of data generated by those technologies will have its own challenges.
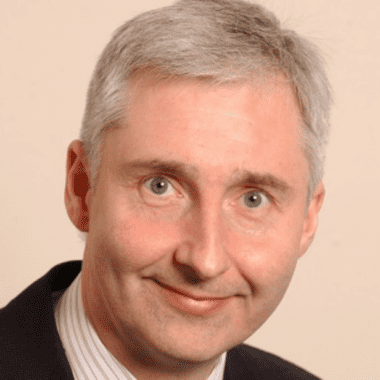
“It may take another 10 years to get to real maturity with AI, but that’s the technology we can see being highly important for the future,” commented Andrew Kinder, Senior Vice President International Strategy at Infor. “There are fantastic opportunities around AI and it’s only just beginning. But I think one of the challenges of that transition is that we have to pay more attention to data.”
Traditionally, noted KInder, manufacturing has always talked about people, processes, and technology, but he believes that companies now need to add data to that essential mix. “We’re already good at getting data, at streaming it into data lakes, and we’re getting better at turning it into actionable insights,” he added. “But now we need to focus much more on how we mature our data mastery for the years ahead.”
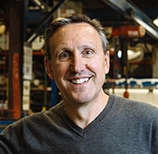
“But before you get to data mastery,” argued MLC Board Vice Chair, Dwight, “you first have to go from legacy to smart tech. The next phase then gets more complicated as the amount of data compounds and we start to adopt new AI approaches with complex algorithms. To make that work, we first need to develop confidence that the data we are gathering is telling us the right story and that becomes more complex as data volumes increase and spread across the enterprise. To cope with that, manufacturers need to be constantly rethinking their business model.”
And it’s not just about rising complexity within the four walls of the company, added EY’s Newman. “The bigger picture is looking at the end-to-end value chain,” he said. “For example, digitizing the supply chain with more accurate forecasting and optimized planning puts pressure on companies along the chain to catch up. As supply chains become more intelligent and complex, companies will need to be more flexible and agile to create more scalable and responsive digital platforms.”
New Business Models
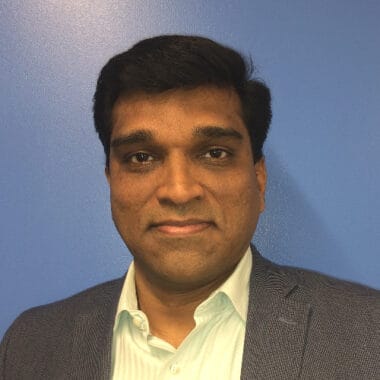
However, warned Radhakrishnan at NTT DATA, “digital will become yesterday’s advantage if organizations are not thinking about moving to the next level. They must think differently about their transformation initiatives so that the traditional ways of operating become a thing of the past. So, with the people, processes, and digital infrastructures they create using new digital technologies, manufacturers should think about reinventing their business models with outcome-based business models, or usage-based business models, or product as a service, and offering their customers more customized products and services.”
Yet that’s a big step for many manufacturers, noted the MLC’s Brousell, and for some it may be a little scary.
“But to me,” added Cooley’s Dwight, “all manufacturing companies, regardless of their relationship to M4.0, demand reinvention. To rest too firmly on last year’s expectations and commitments, prevents your ability to evolve. As I say all the time, the only constant is change – and I don’t mean incremental change.”
“At Cooley,” he continued, “we went through a deep cultural transformation to break down silos to drive collaboration with the objective to become more adaptive and more agile. But for companies who are not change driven, who have not been investing in digitization, who are not rethinking and reinventing, they are the ones who are failing to see the power of digitization and the power of transforming on a constant basis.”
Leadership Advice for the Future
Brousell concluded the discussion by asking the panel: ”What’s your most important piece of advice for manufacturing leaders as they head to 2030?”
“Put together your strategic vision and plan,” responded Kenworthy at West Monroe, “If you don’t have that strategic vision for 2030, you need to start thinking about it now. That will set your roadmap for your digital roll out and plan for the future.”
“I think there a number of dimensions,“ added NTT DATA’s Radhakrishnan, “Develop a fully integrated strategy with very clear transformation goals; leadership commitment all the way from the Board and CEO to middle management; having the right team of high caliber tech talent and subject matter experts; adopt an agile mindset that will drive the broader adoption of digital technology; track progress and measure well-defined success; and, finally ensure there are business-led, modular, technology and data platforms to enable the real transformation”.
“Leaders also need to focus on people,” suggested Infor’s Kinder. “The talent shortage is global and it’s not going to go away. Technology will help, of course, and there is already a change from location centric, to human centric, so leaders need to focus on the reduction or elimination of any non-value-added tasks when intelligent equipment can do that and leave humans to do the high value decision making work. Focus on the people and bring the people with you.”
“Building a digital business ecosystem is only going to reach its full potential when the entire organization is digitally driven, and driven seamlessly across traditional functional lines,” said Dwight. “As the white paper says: ‘Digital is agnostic about functional boundaries.’ I believe this transformation is going to be the most difficult piece of digital evolution that leaders are going to have to grapple with.”
EY’s Newman also added his final thoughts: “While the future will be centered on cross-industry collaboration,” he observed, “I look forward to seeing the manufacturing industry take the lead when it comes to innovating new business models and engaging ecosystems. I think our ability to do this will wildly change the trajectory of the industry, driving better investments across safety, sustainability, technology, and most importantly, the development of our people.”
———-
* Download the full MLC White Paper, Manufacturing in 2030: The Next Phase of Digital Evolution
* Listen to the insights shared during the Manufacturing in 2030 White Paper Panel Discussion
M2030 Perspective: The Manufacturing Metaverse
Manufacturing Leadership Journal content and MLC resources are exclusively available to MLC members. Please sign up for an account or log in to view this content.
The Rise of Digital Manufacturing Ecosystems
Manufacturing Leadership Journal content and MLC resources are exclusively available to MLC members. Please sign up for an account or log in to view this content.
The Coming Distributed Enterprise: Local, Customized, and Flexible
Manufacturing Leadership Journal content and MLC resources are exclusively available to MLC members. Please sign up for an account or log in to view this content.
M2030 Perspective: Moving to M4.0’s Second Phase
Manufacturing Leadership Journal content and MLC resources are exclusively available to MLC members. Please sign up for an account or log in to view this content.
Putting Intelligence Back into AI
Today’s version of artificial intelligence is at risk of undercutting the power of knowledge.To free this important technology, here are 10 recommendations that will enable AI in manufacturing operations to prosper. By Jim Davis
Artificial intelligence and machine learning have taken on silver bullet status for advanced manufacturing with entire countries investing in programs and competing for global leadership. This resurgence in interest has been propelled over the past 10 years or so with significant and publicly prominent advances in particular AI areas, notably game playing, robotics, self-driving cars, speech recognition, facial recognition, and many decision systems we experience as consumers of products and services. Of course, the other major factor is today’s access to data management and computational capabilities that simply did not exist a decade ago.
Unprecedented capacity to collect, work with, and manage massive amounts of data are now commonplace with network-based cloud services; graphical processor units, not just CPUs; and, more recently, computationally rich edge-cloud capabilities. Altogether, breakthrough data science, IT infrastructure and methods now exist for learning systems applied to amazingly large data sets. These include deep convolutional neural networks, a host of data clustering approaches, reinforcement learning, recurrent neural networks, and statistical learning.
A particularly important class of AI/ML applications in manufacturing involves measuring the state of an operation, analyzing it, and determining automated and human-centered actions to be taken at the right time. For those of us involved with the early work on this class of AI/ML applications in the 1980’s, we know all too well why the technology did not take off and all but disappeared. We were working with primitive graphics, experimental AI machines, and early object-oriented and AI languages. The web did not yet exist, ERP systems had not come into vogue, and cloud services had not been thought about. On the plus side, cyber security was not yet an issue.
More importantly, manufacturing’s prevailing coding mindset was asset- and operations-oriented. Object-oriented programming did not get any traction (and still has not) as a concept for structuring process knowledge for better usability. Digitization was occurring but digitalization and the data revolution had not begun. The industry just wasn’t ready conceptually, technically, or with adequate infrastructure.
For engineering applications, the term AI literally became a negative in the 1990’s. Those of us who continued in the field disguised our work with terms like Data Analysis and Interpretation and Intelligent Systems. Keep in mind that Smart Manufacturing did not enter the vocabulary of interest until around 2005. Industrie 4.0 in Germany started about the same time under the heading of Smart Factory. What we did do in the 1980’s was learn a lot about the role of domain knowledge and how to use and embed knowledge with ML solutions.
What Has Been Lost
Fast forward to today, the global resurgence in AI/ML, largely since 2005, is amazing to observe, having advocated for its value for so many years. Furthermore, it is remarkable just how far the processors, tools, platforms, infrastructure and foundational data science have progressed in such a short time in comparison to how constrained we were in the 80’s and 90’s. Notably, this resurgence has produced a large number of software tools and platforms with the critical IT and data science underpinnings that can respond computationally to today’s massive increases in data that are in amounts that far surpass human capability to assimilate.
In an era of IIoT, it is quite possible to quickly and almost randomly apply tools to produce apparently reasonable results with huge data sets. When the covers are peeled back, however, we are seeing AI/ML solutions that are constructed without the needed analysis of the data or the alignment of data with objective. For example, it is easy to construct solutions with the faulty assumptions that there are enough right data, the data contain every scenario of interest, and the algorithm will figure it out. All too often, solutions are re-learning the physics of an operation or are erroneously making decisions keying off noise or overly dominant variables.
Without a thorough grounding in understandablility, AI/ML
approaches will be biased and untrusted, and more likely to fail.
Put more generally, today’s version of AI has undercut the power of knowledge and is over relying on the data tools and data science. This is a trap that has long proved faulty. Furthermore, AI/ML solutions are so focused on the ML aspect that the capacity for cognitive interpretation, explanation, and understandability, which are AI needs and strengths, are essentially missing in action. The reality is that without a thorough grounding in understandability and explainability, AI/ML applications in manufacturing operations are more likely to be biased, brittle, unpredictable, untrusted, difficult to sustain, and much more likely to fail as a result.
In addition, apparently lost is just how much the engineering of an AI/ML solution is a human-centered process, albeit significantly enabled by technology. The industry surely needs to be engaging in the low-hanging fruit opportunities, while it also needs to be wary of a false sense of readiness given the progress with new data capabilities.
Interest Spans Many Levels
In the space of manufacturing execution and control, applications (measuring state, analyzing, and taking action), there is interest in AI applications spanning all operational levels – device, process, line operation, and supply chain. It is important to consider cognition, explanation and understandability, the need for embedded knowledge, and the requirement to select and contextualize data for objective. When characterized from these perspectives, manufacturing AI application interests include discover, monitor, diagnose, predict, optimize, self-interrogate, customize, and visualize (interoperate with humans). Individually and in combination, all are for the larger purposes of controlling and managing an operation to economic, social, consumption, environmental, and safety objectives that define competitiveness.
In looking to 2030, let’s dust off some of the old lessons learned from the 80’s and 90’s. These characterize aspects to watch out for now and that need attention and development for AI/ML solutions in manufacturing operations to prosper.
- An AI/ML solution is doomed to failure if the assumption is that the algorithm will figure it out. Also, if you are confused about the problem definition, the system will be too.
- Cognition, interpretation, understandability, and applied knowledge are key strengths and requirements for AI solutions. Explainable models and models that can explain go hand-in-hand.
- Use the full span of modeling to include not only ML, first principles, and math modeling but also qualitative modeling and interpretation modeling. Explainable models are hybrid.
- Be careful not to formulate an AI/ML solution to take on too many decisions with a single method. It won’t be able to do it and you won’t be able to explain it.
- Formulate AI solutions to align with how operational complexity typically emanates as interactions among simpler elements. Causation is as important as correlation and patterns.
- AI/ML algorithms that work well for math modeling, such as neural networks and statistical modeling, do not necessarily work well for interpretation. The reverse is true for interpretation modeling.
- State measurements in time-varying systems involve time-windows applied over time. Interpretation and the role of time need to be engineered carefully with the domain in mind.
- No ML model ever works without enough of the right data, no matter the volume. It is not possible to circumvent the knowledge and lift needed to contextualize data for an objective.
- Build infrastructure, technology, and practice for nurturing an AI system for sustainability, learning, unlearning, and continuous improvement. An AI system is a human-centered engineering process.
- People trust people first, not machines, but also easily over rely on algorithms. Don’t underestimate, be prepared, and engineer AI/ML systems for explaining ethical considerations.
Looking ahead, the great opportunity is to engineer sustainable AI applications that produce understandable and explainable results.
The Limits of Math
We engineers value our mathematics and there is a very rich arsenal of tools and platforms for processing numeric data and doing mathematical modeling1. Advances in ML have significantly added to this arsenal. As valuable as the math-based approaches are, they have their limits as higher level considerations and/or greater amounts and breadths of data become of interest. Examples include reasoning about cause and effect, time and workflow, conditional considerations, and combinations of systems. There is also the need to explain predictions, and interface with humans.
In manufacturing, these are problems where there often is not enough of the right data and where interpretation and explanation are not only important, but also take advantage of useful knowledge modeling strategies in the form of decomposition, causality, inference, patterns, clusters, and workflow. Also, physics-based models in qualitative forms can be rigorous and as useful as math-based models.
The good news is that after all these years, AI/ML is poised for success in manufacturing operations. That success will depend on the amount of right data and the right models that address the application of knowledge. CESMII, the Clean Energy Smart Manufacturing Innovation Institute2 has, for example, focused considerable effort on infrastructure for managing contextualized data and the development of reusable application profiles which capture critical forms of knowledge and connects them to the contextualized data for operational use.
Looking ahead to 2030, the great opportunity for AI in manufacturing is to be able to engineer sustainable AI applications that produce understandable and explainable results and that can be trusted as a result. New data science-based platforms, tools, and methods are in sight that bring today’s extensive ML capabilities together with the ability to more easily contextualize data to objective, reuse embedded knowledge, and incorporate a far richer array of modeling strategies. M
1 Consider univariate, probabilistic and multivariate categories of single scale, multiscale, projection-based, kernel-based, partition-based linear and non-linear methods.
2 CESMII is one of the fifteen Manufacturing USA Institutes and is among the five that are sponsored by DOE.
The Shape of Things to Come
The future state of manufacturing is envisioned as a highly efficient, high-tech engine of mass customization. But competitive advantage will flow only to those companies that master the use of advanced technologies and orchestrate change well.
By David R. Brousell
In 2017, the Manufacturing Leadership Council published “Vision 2030: The Factory of the Future”,an attempt to paint a picture of what manufacturing might look like in the years ahead based on trends that were underway as part of the Fourth Industrial Revolution.
Beginning with this issue of the Journal, the MLC picks up on this work in order to see what is new, what has changed, and what has remained the same since the work was conducted more than three years ago. We will also use various other MLC venues and formats next year to update and refine our vision about the future of manufacturing.
The Vision 2030 effort consisted of extensive research into industry databases and published reports, interviews with MLC members, and a two-day workshop with technology experts, academics, and members of MLC’s Board of Governors. The result was the development of a framework consisting of broad economic, social, and technology “mega-trends” affecting manufacturing as well as manufacturing-specific themes flowing from the broad trends.
Among the mega-trends identified at the time were: economic and social trends such as globalization and urbanization; smart approaches to innovation and sustainability; business model advances based on smart products and data analytics; and what Vision 2030 called “ambient intelligence”, including cloud-based solutions, digital platforms and applications, machine learning, and the Internet of Things.
The manufacturing-specific themes identified were Intelligent Design, enabling mass customization of products as well as production closer to the point of consumption; the Services Revolution, which predicted the rise of as-a-service business models based on technologies such as IoT and 3D printing; the Platform Revolution, in which data will be used to improve designs as well as anticipate consumer preferences; and Human-to-Machine Convergence, which discussed the need for human capital transformation as a result of the new skills and competencies required with digital manufacturing.
The Trends Continue to Unfold
In large part, the megatrends and themes MLC identified continue to this day and will continue to play out in the years ahead. What has changed since the 2017 report is greater emphasis today on the digital disciplines necessary for manufacturers to succeed with digital transformation.
In particular, there is a heightened need for manufacturers to achieve what the MLC now calls “digital acumen” in their leadership ranks and digital literacy in their workforces; mastery over the collection, organization, and analysis of data; and the need to understand the potential of advanced technologies to create new business models, products, and services. Many manufacturers are still in the early stages of developing competencies in these areas.
Over the next 10 years, the underlying technical infrastructure of computing and communications will continue to get stronger.
What was not foreseen at the time Vision 2030 was crafted was that a global pandemic would erupt in 2020, killing hundreds of thousands of people and severely damaging economies worldwide. But the good news, according to MLC surveys taken in the wake of the pandemic, is that a significant number of manufacturing companies plan to accelerate their adoption of the digital model as a direct result of the pandemic in order to have the flexibility and agility necessary to cope with disruption.
If indeed the inflection point reached this year is sustained over time, the manufacturing industry will accelerate its journey to a future state that will bear unprecedented levels of efficiency, productivity, and innovation, the result of which will change the rules of competition in the global manufacturing industryy
“New technologies are demanding higher skill levels, raising the capital intensity of production, elevating the importance of innovation ecosystems, and requiring strong digital infrastructure and readiness for manufacturers to be competitive,” said a Brookings Institution report published in March. “Countries that currently possess or are actively investing in the skills, capital, and infrastructure of the future are the ones that will dominate global manufacturing in the years ahead.”
Based on MLC’s 2017 research and what we have learned since, the general outlines of what future factories will look like are coming more clearly into view. Future factories will be high-tech, software-driven engines of customized mass production. They will be more automated, more connected and integrated, and more information-intensive than ever before. They will be organized for greater speed, flexibility, and productivity and efficiency.
The people who work in them will have advanced skills and technical competency with the ability able to work cross-functionally across the connected enterprise. Routine, repetitive work will become the province of intelligent machines. And leaders will have digital acumen – the ability to understand the potential of advanced technologies to not only achieve incremental improvements in efficiency and cost effectiveness but also to change business models and create new opportunities.
The underlying trends compelling these changes appear to be inexorable. As we have seen over several decades, manufacturing prowess – its ability to be efficient, productive, and fast-moving – has been increasingly linked to adoption of both operational technologies used on the plant floor as well as information technologies used to manage many aspects of the enterprise, two technology streams that have been converging for more than 20 years.
The Technical Infrastructure
Over the next 10 years, the underlying technical infrastructure of computing and communication networks is expected to become more powerful. The infrastructure includes platforms that enable business and operational automation, and the data collection and analysis necessary to make decisions on everything from demand forecasting to inventory levels.
“Future factories will be more automated, more connected and integrated, and more infomration-intensive than every before.”
Microprocessors, the foundation of computing power, are expected to continue to increase in power based on Moore’s Law, which holds that the number of transistors that can be placed on a chip doubles every 18 months. Price/performance improvements follow the law. For example, in 1970, a chip with 2,000 transistors cost $1,000 to build. Today, that same chip would cost $0.02 to build.
Power improvements follow a similar curve. In 1970, the Intel 4004 chip contained about 1,000 transistors. Today, the Samsung V-NAND flash memory chip contains two trillion devices.
Similarly, as more people and physical objects become networked, the power and potential of networks continue to increase based on Metcalfe’s Law, which holds that a network’s value is proportional to the square of the number of nodes in a network. As manufacturing networks both inside the manufacturing enterprise and outside in a company’s business ecosystem continue to expand, the possibilities for greater information and business-changing insights from it become exponentially larger.
More powerful and inexpensive electronics, coupled with advancements in software, drive improvements in computing power, resulting in advances such as nano-computing and quantum computing. Over the next 10 years, continued advancements will enable manufacturers to process an even larger quantity of data from operations, supply chains, customers, and partners as companies move toward artificial intelligence-based systems and into a pervasively connected business ecosystem.
On the shop floor, more and more automation of routine and even semi-sophisticated tasks will occur as robotics systems become more capable, as equipment maintenance becomes more predictive through better analysis of sensor-generated data, and the overall manufacturing process is improved through digitization.
The improving computing and communications capabilities are facilitating a shift to mass customization, making it possible to satisfy individual needs in everything from transportation to medicine. Pervasive connectivity is generating vast new quantities of information about every functional aspect of the manufacturing enterprise, the products and parts that are made, and the customers these products are sold to. As a result, we can envision extensive digital threads spanning from design to end-customer usage, an information loop that will enable manufacturers to continuously improve increasingly intelligent products.
The advances will also enable manufacturers to move from reacting to problems with machines and other equipment on the plant floor and elsewhere to predicting those issues through data- and analytics-based insights, driving far greater operational efficiency and improvements in uptime.
Organizationally, extensive process digitization will enable manufacturers to shift from what has largely been functional automation of processes, which has historically created siloes of information, to cross-functional integration of people, processes, and systems. Accompanying this is a shift from command-and-control organizational structures to flatter, more collaborative models of organizing work and people, with decisions made at the lowest level possible. As digitization continues, its effect in changing how manufacturing companies are structured will be felt throughout the organizational layers that currently make up how many companies are structured, in particular middle management.
A Heterogeneous Industry
As a result of these trends, manufacturers will benefit from insights into how manufacturing processes and manufactured products can be improved, how unmet customer needs can be addressed, and how new business models and opportunities can be identified.
But the vision of manufacturing as a highly agile and flexible operation powered by data-driven insights will not play out in identical fashion in every sector that makes up the diverse manufacturing industry. Sectors such as aerospace and defense will continue to require huge centralized factories and extended supply chains to produce their large products. On the other side of the spectrum, consumer product goods companies will be increasingly focused on providing local production capabilities to serve their markets.
One of the most important keys to competitive success will be how well manu-
facturers use digital tools.
But regardless of sector, the common threads are evident. In the decade ahead and beyond, factories and plants will be distinguished by a now evolving set of technological, organizational, and leadership characteristics that will set them apart from facilities of the past.
The extent and depth of change underway will be profound. The day is coming when human beings will work routinely alongside machines that can learn, think, and act. The convergence between people and intelligent machines now underway will be as revolutionary as the advent of mass production techniques a century ago. Quantum leaps in productivity and efficiency will result. But one of the key challenges over the next 10 years will be for industry to proactively redefine the relationship between humans and machines and understand the impact of this evolving relationship on how production is conducted, on the composition of the manufacturing workforce, and on work itself.
Overall, future factory models will change dramatically as manufacturers ride the wave of mass customization. Depending on industry sector and type of product produced, manufacturers will design their factory footprints in myriad ways, from micro factories positioned to serve local markets to mega factories designed to make the largest and most complex products. The thing they all will have in common, though, is the ability to operate with a far greater level of operational intelligence and flexibility as a result of the new technologies now available.
And just where will the growth opportunities be for manufacturers as we head toward 2030?
Population and Economic Directions
Let’s look at this question through the lens of population and economic trends.
The world’s population is expected to grow to about 8.5 billion people by 2030, from 7.8 billion this year. In the United States, the population is projected to increase 7.4% to 359.4 million in the next 10 years, compared with 334.5 million today.
But the U.S. and other western countries will not be the areas where population growth will be the strongest. According to studies by the United Nations, Africa, currently at 1.3 billion people, is expected to more than triple its population by the end of the century.
On the global economic front, studies done before the COVID-19 pandemic also indicate a shift in growth patterns.
A 2017 study by consultancy PwC projects that the world economy could more than double in size by 2050, far outstripping population growth due to technology-driven productivity improvements. But emerging markets, PwC said, could grow twice as fast as advanced economies, on average.
“MxD recognizes that the number of roles — 247 — by itself can be intimidating.”
As a result, six of the seven largest economies of the world are projected to be what PwC calls “emerging economies” by 2050, led by China, India, and Indonesia. In this scenario, the U.S. would drop to third place, from second place; Japan would drop to eighth place, from fourth; Germany would drop to ninth place, from fifth; and the United Kingdom would be in 10th place. France and Italy would leave the top 10 list entirely. Taking Europe as a whole, PwC said that the EU27’s share of world GDP could fall below 10% by 2050.
Like any business, manufacturing companies are similar to biological organisms; they either grow or they wither and die. A key question based on population and economic trends, particularly for global manufacturing companies, is: where should factory and plant investments be made in the next 10 years to capitalize on these growth trends?
Keys to Success
As manufacturers ponder these questions, the winds of technological change will continue to blow, reshaping conceptions of how factories and plants will operate and even how manufacturers define success in the digital age.
The art and science of devising digital metrics as well as return on investment models may also require redefinition. As a Deloitte report published in April said: “The rise of advanced manufacturing technologies appears to be redefining traditional methods for measuring production, inventory, and other traditional performance metrics.”
Moreover, realizing the future state of manufacturing will not be easy, inexpensive, or without missteps or even failure.
Manufacturers will have to carefully adopt a vast array of new technologies, ranging from artificial intelligence, to augmented reality systems, to 3D printing machines. They will have to plan investments in plants and equipment wisely based on understanding changing markets, consumer expectations, political sentiments, and emerging growth markets. They will have to understand the roles, skills, and competencies they need to succeed with the digital model and adjust their workforces accordingly. And they will have to be willing to experiment to see the possibilities in the new digital age and from time to time accept failure on their journey forward. For many, massive cultural and organizational change is on their doorsteps.
One of the most important keys to competitive success for manufacturers, who will be operating in an industry where over the next 10 years widespread adoption of advanced technologies will be the equivalent of having a college degree, is how well they actually use the digital tools that are available to all. That’s an equation that will require significant efforts in learning and training; change management, including culture change; and orchestration by leadership. Competitive advantage will flow to the companies that master these challenges.
But as we said in 2017, perhaps the most important requirement for success will be trust, the confidence that pervasive connectivity will not result in theft, interruptions, or damage, and that decision-critical information is indeed true, accurate, and secure. Moreover, workers will need to trust that their companies will help them make the transition to the digital way of doing things, and nations will need to trust that the shift to more automation, often in response to global trends such as the pandemic, will not create unacceptable societal disruptions.
Throughout the ages, trust has been perhaps the one truly indispensable ingredient for business. It will be no different for manufacturers in 2030.
Figure 1: The Road to 2030: Key Megatrends to Watch
World Population: By 2030, the world’s population is expected to be 8.5 billion people, up from 7.8 billion today. Africa will see the strongest growth rate.
Global Economy: PwC estimates the global economy could double in size by 2050, with China, India, and Indonesia leading the way.
Globalization: Will nationalistic political winds continue to blow through western democracies?
Environmental: Manufacturers will continue to emphasize circular economy ideas. The incoming Biden Administration may rejoin the Paris climate agreement.
Consumer Expectations: Personalization, the online experience, and rapid delivery will continue to drive changes in production, supply chain, and logistics models.
Cybersecurity: Information security will continue to rise in importance. Manufacturers will need to weave cybersecurity into their cultures much like they did with physical safety.
Figure 2: The Road to 2030: Key 4.0 Disciplines
- Keep the technology infrastructure modernized. Cloud adoption will increase.
- Maintain lean disciplines as a foundation for digital.
- Rethink processes in parallel with tech and automation investments.
- Break down silos, cross-functionally integrate, and build common operating platforms for plants and factories.
- Develop digital acumen in leadership teams.
- Become data-driven and develop mastery in how data is analyzed and used in decision-making processes.
- Diversify the workforce, including bringing in people from non-manufacturing sectors.
- Maintain a willingness to take risks.
- Disrupt your market before you are disrupted.
1 Sath Rao, “Digital Innovation: Scaling for the Fast and Furious Future,” Manufacturing Leadership Journal, April 2020, https://www.manufacturingleadershipcouncil.com/2020/04/10/digital-innovation-scaling-for-the-fast-and-furious-future/
2 Jared Spataro, “2 Years of Digital Transformation in 2 Months,” Microsoft 365 (blog), April 30, 2020, accessed August 10, 2020, https://www.microsoft.com/en-us/microsoft-365/blog/2020/04/30/2-years-digital-transformation-2-months/
3 Sath Rao, “The Case of the Missing Insights,” Manufacturing Leadership Journal, October 2019, https://www.manufacturingleadershipcouncil.com/2019/10/04/the-case-of-the-missing-insights/
4 Knut Alicke, Richa Gupta and Vera Trautwein, “Resetting Supply Chains for the Next Normal,” McKinsey & Company, July 21, 2020, https://www.mckinsey.com/business-functions/operations/our-insights/resetting-supply-chains-for-the-next-normal
5 Joglekar N., Parker G., and Srai J. S., “Winning the Race for Survival: How Advanced Manufacturing Technologies Are Driving Business-Model Innovation,” https://papers.ssrn.com/sol3/papers.cfm?abstract_id=3604242
6 Sath Rao, “Digital Innovation.”
7 Fleming Shi, “Threat Spotlight: Coronavirus-Related Phishing,” Barracuda, March 20, 2020, https://blog.barracuda.com/2020/03/26/threat-spotlight-coronavirus-related-phishing/
8 Edward Barriball, Katy George, Ignacio Marcos and Philipp Radtke, “Jump-Starting Resilient and Reimagined Operations,” McKinsey, May 11, 2020, https://www.mckinsey.com/business-functions/operations/our-insights/jump-starting-resilient-and-reimagined-operations
9 Sath Rao, “The Case of the Missing Insights.”
10 David Uberti, “How BMW Used Pandemic Plant Stoppages to Boost Artificial Intelligence,” The Wall Street Journal, July 28, 2020, https://www.wsj.com/articles/how-bmw-used-pandemic-plant-stoppages-to-boost-artificial-intelligence-11595939400?mod=djemAIPro
11 Rajesh Devnani and Justin Bean, “Health and Safety of Workers,” Hitachi, https://social-innovation.hitachi/en-us/think-ahead/manufacturing/health-and-safety-of-workers/
12 Chris Walton, “3 Ways Starbucks Will Emerge from COVID-19 Stronger Than Before,” Forbes, April 3, 2020, https://www.forbes.com/siteschristopherwalton/2020/04/03/3-ways-starbucks-will-emerge-from-covid-19-stronger-than-before/#1d89d6641844